Handling constrained multi-objective optimization problems with heterogeneous evaluation times: proof-of-principle results
Memetic Computing(2022)
摘要
Most real-world optimization problems consist of multiple objectives to be optimized and multiple constraints to be satisfied. Moreover, the performance assessment of the objective and constraints often requires running different software packages separately along with evaluating mathematically defined functions with significantly different (heterogeneous) computing times. A single software package may compute a functional group of objectives and constraints as a block, thereby creating a complicated computing pattern in evaluating a single population member. While much effort has been made to handle computationally expensive functions in the literature, little attention has been paid to handle heterogeneously expensive optimization problems. This paper focuses on efficient and adaptive ways to schedule an evaluation of different functional groups of objectives and constraints by utilizing the potential of offspring population members in terms of their multi-objective ranks, the accuracy of low-fidelity models to predict their function values, and their computing times. Results on a number of unconstrained and constrained two and three-objective optimization problems show significantly better convergence than non-heterogeneous methods. This proof-of-principle study must now be extended by taking full advantage of surrogate-assisted optimization methods to propose more comprehensive and practical optimization algorithms.
更多查看译文
关键词
Heterogeneously expensive functions, Multi-objective optimization, Constrained optimization
AI 理解论文
溯源树
样例
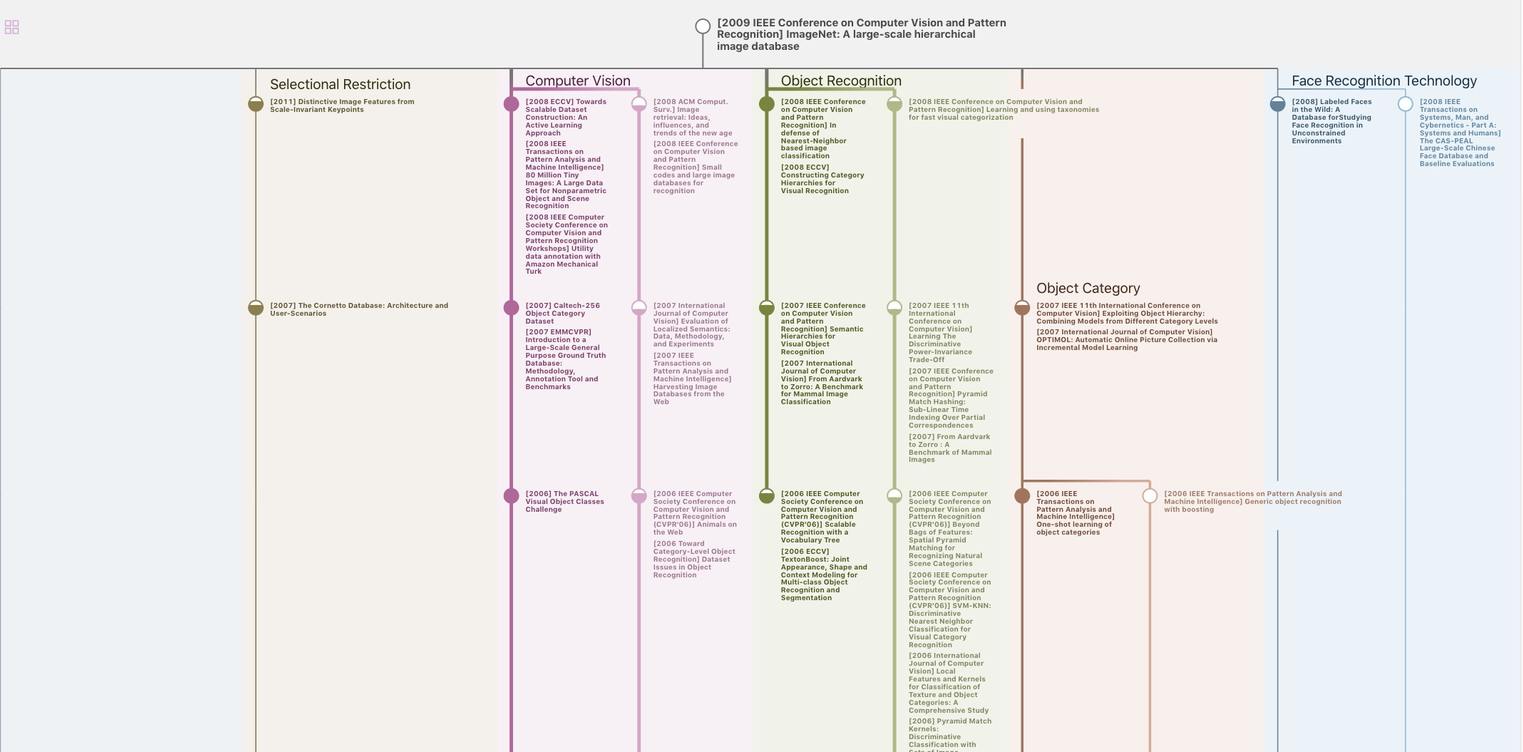
生成溯源树,研究论文发展脉络
Chat Paper
正在生成论文摘要