Investigation of Two Neural Mass Models for DCM-based Effective Connectivity Inference in Temporal Epilepsy
Computer methods and programs in biomedicine(2022)
摘要
Background and objectiveRecently, spectral Dynamic Causal Modelling (DCM) has been used increasingly to infer effective connectivity from epileptic intracranial electroencephalographic (iEEG) signals. In this context, the Physiology-Based Model (PBM), a neural mass model, is used as a generative model. However, previous studies have highlighted out the inability of PBM to properly describe iEEG signals with specific power spectral densities (PSDs). More precisely, PSDs that have multiple peaks around β and γ rhythms (i.e. spectral characteristics at seizure onset) are concerned.MethodsTo cope with this limitation, an alternative neural mass model, called the complete PBM (cPBM), is investigated. The spectral DCM and two recent variants are used to evaluate the relevance of cPBM over PBM.ResultsThe study is conducted on both simulated signals and real epileptic iEEG recordings. Our results confirm that, compared to PBM, cPBM shows (i) more ability to model the desired PSDs and (ii) lower numerical complexity whatever the method.ConclusionsThanks to its intrinsic and extrinsic connectivity parameters as well as the input coming into the fast inhibitory subpopulation, the cPBM provides a more expressive model of PSDs, leading to a better understanding of epileptic patterns and DCM-based effective connectivity inference.
更多查看译文
关键词
Epilepsy,Neural mass model,Effective connectivity,Power spectral density,Spectral dynamic causal modelling
AI 理解论文
溯源树
样例
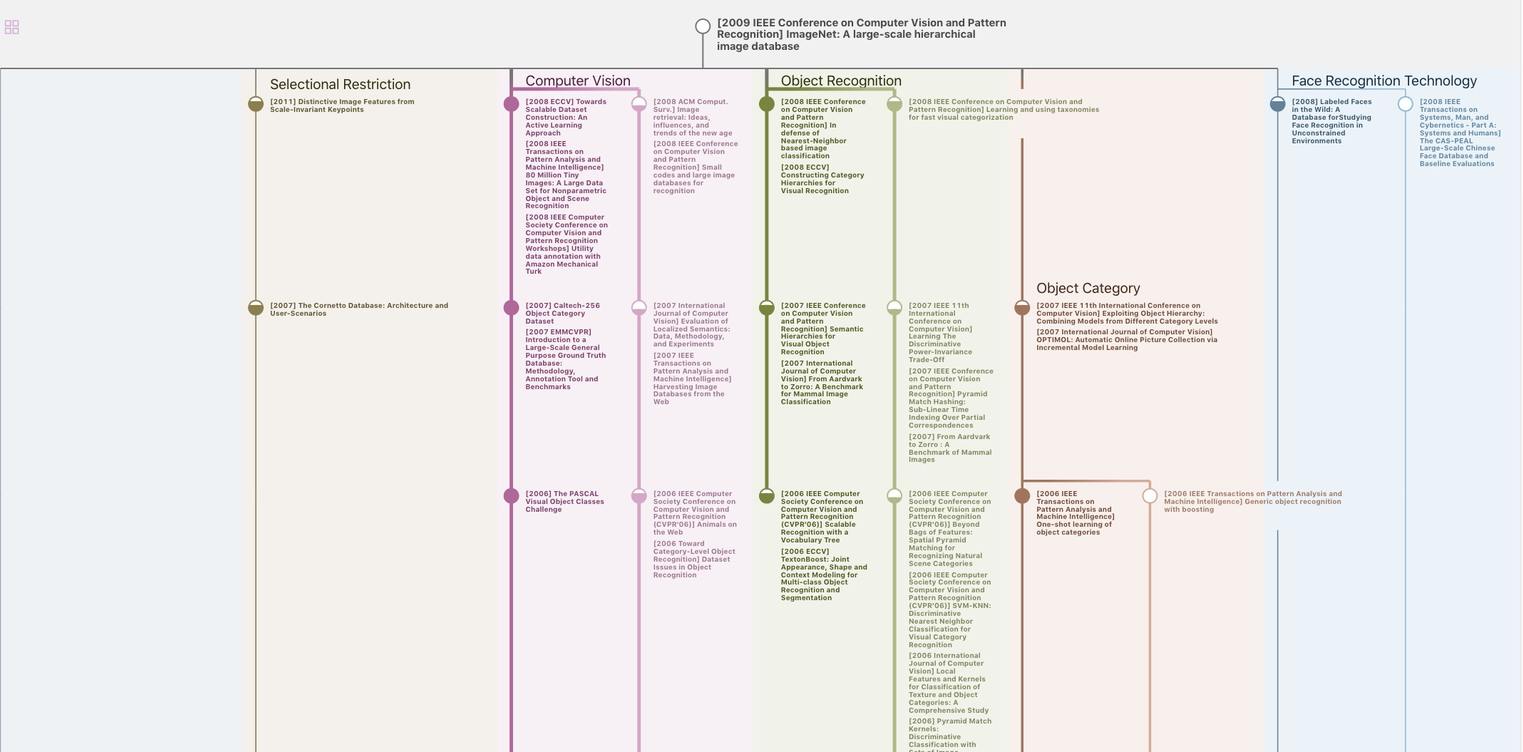
生成溯源树,研究论文发展脉络
Chat Paper
正在生成论文摘要