Task model-specific operator skill assessment in routine fetal ultrasound scanning
International Journal of Computer Assisted Radiology and Surgery(2022)
摘要
Purpose For highly operator-dependent ultrasound scanning, skill assessment approaches evaluate operator competence given available data, such as acquired images and tracked probe movement. Operator skill level can be quantified by the completeness, speed, and precision of performing a clinical task, such as biometry. Such clinical tasks are increasingly becoming assisted or even replaced by automated machine learning models. In addition to measurement, operators need to be competent at the upstream task of acquiring images of sufficient quality. To provide computer assistance for this task requires a new definition of skill. Methods This paper focuses on the task of selecting ultrasound frames for biometry, for which operator skill is assessed by quantifying how well the tasks are performed with neural network-based frame classifiers. We first develop a frame classification model for each biometry task, using a novel label-efficient training strategy. Once these task models are trained, we propose a second task model-specific network to predict two skill assessment scores, based on the probability of identifying positive frames and accuracy of model classification. Results We present comprehensive results to demonstrate the efficacy of both the frame-classification and skill-assessment networks, using clinically acquired data from two biometry tasks for a total of 139 subjects, and compare the proposed skill assessment with metrics of operator experience. Conclusion Task model-specific skill assessment is feasible and can be predicted by the proposed neural networks, which provide objective assessment that is a stronger indicator of task model performance, compared to existing skill assessment methods.
更多查看译文
关键词
Skill assessment,Ultrasound,Fetal ultrasound,Deep learning
AI 理解论文
溯源树
样例
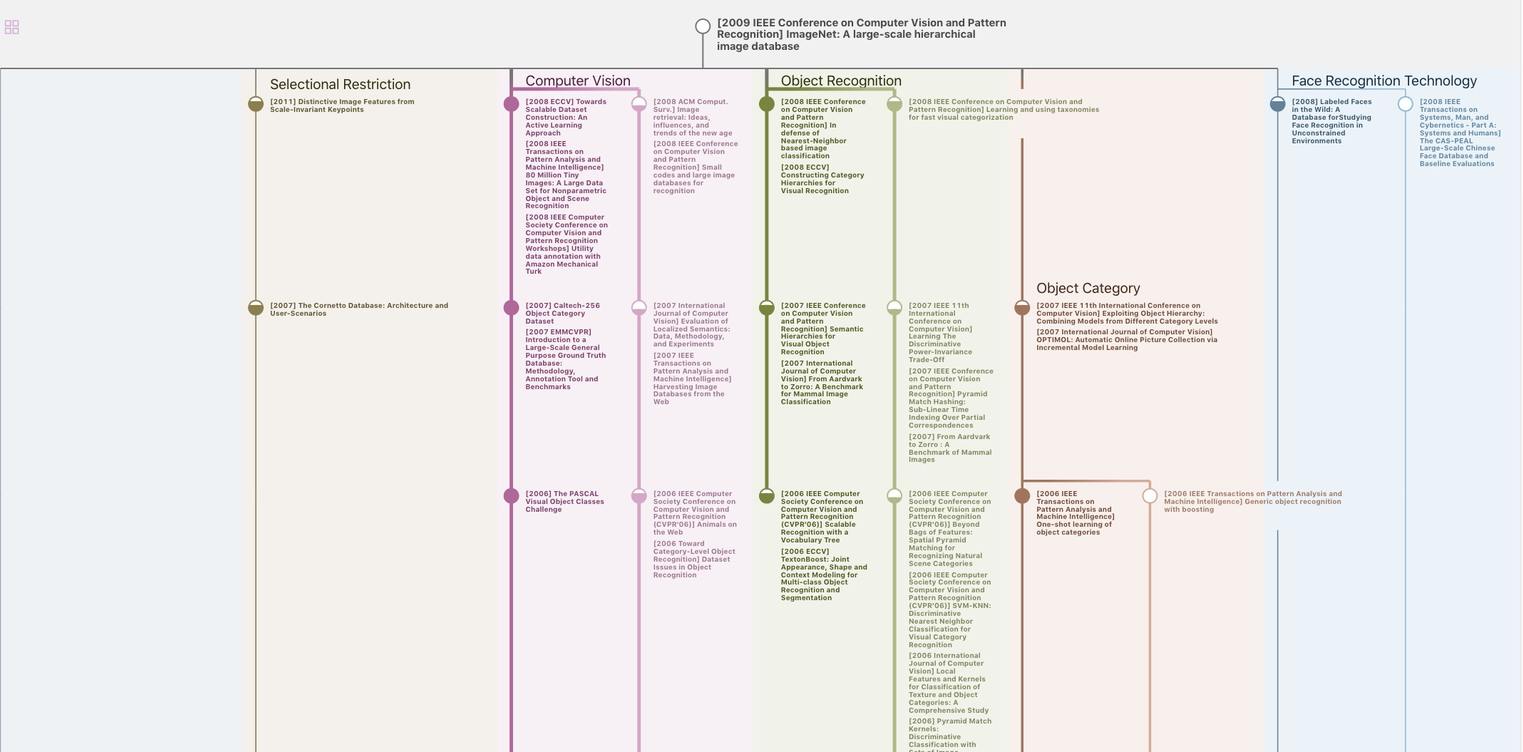
生成溯源树,研究论文发展脉络
Chat Paper
正在生成论文摘要