Focal Liver Lesion MRI Feature Identification Using Efficientnet and MONAI: A Feasibility Study
CELLS(2022)
摘要
Liver tumors constitute a major part of the global disease burden, often making regular imaging follow-up necessary. Recently, deep learning (DL) has increasingly been applied in this research area. How these methods could facilitate report writing is still a question, which our study aims to address by assessing multiple DL methods using the Medical Open Network for Artificial Intelligence (MONAI) framework, which may provide clinicians with preliminary information about a given liver lesion. For this purpose, we collected 2274 three-dimensional images of lesions, which we cropped from gadoxetate disodium enhanced T1w, native T1w, and T2w magnetic resonance imaging (MRI) scans. After we performed training and validation using 202 and 65 lesions, we selected the best performing model to predict features of lesions from our in-house test dataset containing 112 lesions. The model (EfficientNetB0) predicted 10 features in the test set with an average area under the receiver operating characteristic curve (standard deviation), sensitivity, specificity, negative predictive value, positive predictive value of 0.84 (0.1), 0.78 (0.14), 0.86 (0.08), 0.89 (0.08) and 0.71 (0.17), respectively. These results suggest that AI methods may assist less experienced residents or radiologists in liver MRI reporting of focal liver lesions.
更多查看译文
关键词
focal liver lesion, deep learning, radiological feature, hepatocellular carcinoma, liver metastasis, gadoxetate disodium, abdominal MRI, multidimensional imaging
AI 理解论文
溯源树
样例
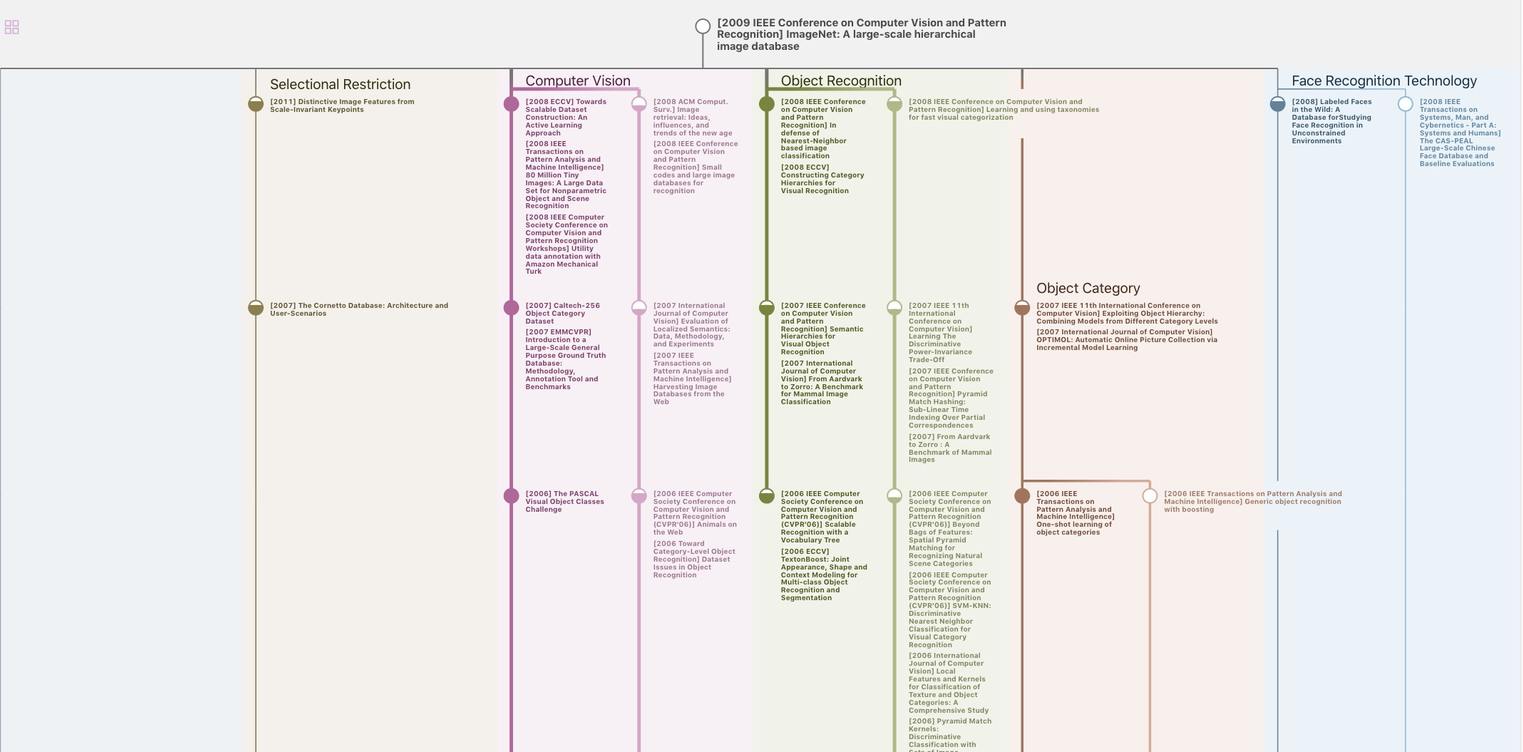
生成溯源树,研究论文发展脉络
Chat Paper
正在生成论文摘要