Machine Learning Based Analysis of Relations between Antigen Expression and Genetic Aberrations in Childhood B-Cell Precursor Acute Lymphoblastic Leukaemia
JOURNAL OF CLINICAL MEDICINE(2022)
摘要
Flow cytometry technique (FC) is a standard diagnostic tool for diagnostics of B-cell precursor acute lymphoblastic leukemia (BCP-ALL) assessing the immunophenotype of blast cells. BCP-ALL is often associated with underlying genetic aberrations, that have evidenced prognostic significance and can impact the disease outcome. Since the determination of patient prognosis is already important at the initial phase of BCP-ALL diagnostics, we aimed to reveal specific genetic aberrations by finding specific multiple antigen expression patterns with FC immunophenotyping. The FC immunophenotype data were analysed using machine learning methods (gradient boosting, decision trees, classification rules). The obtained results were verified with the use of repeated cross-validation. The t(12;21)/ETV6-RUNX1 aberration occurs more often when blasts present high expression of CD10, CD38, low CD34, CD45 and specific low expression of CD81. The t(v;11q23)/KMT2A is associated with positive NG2 expression and low CD10, CD34, TdT and CD24. Hyperdiploidy is associated with CD123, CD66c and CD34 expression on blast cells. In turn, high expression of CD81, low expression of CD45, CD22 and lack of CD123 and NG2 indicates that none of the studied aberrations is present. Detecting aberrations in pediatric BCP-ALL, based on the expression of multiple markers, can be done with decent efficiency.
更多查看译文
关键词
acute lymphoblastic leukaemia, flow cytometry, cytogenetics, machine learning, knowledge discovery, classification
AI 理解论文
溯源树
样例
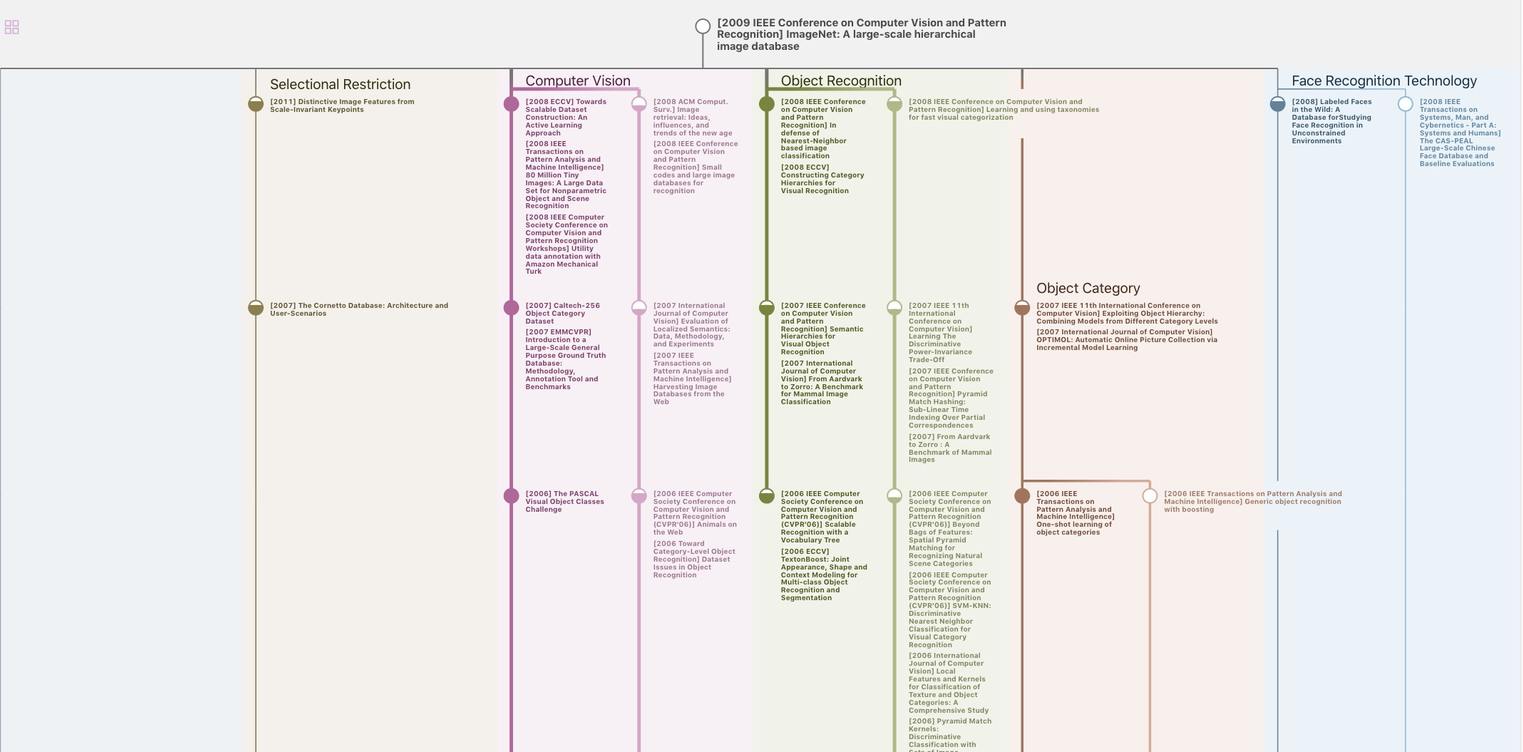
生成溯源树,研究论文发展脉络
Chat Paper
正在生成论文摘要