Graph neural network modeling of grain-scale anisotropic elastic behavior using simulated and measured microscale data
arxiv(2022)
摘要
Here we assess the applicability of graph neural networks (GNNs) for predicting the grain-scale elastic response of polycrystalline metallic alloys. Using GNN surrogate models, grain-averaged stresses during uniaxial elastic tension in low solvus high-refractory (LSHR) Ni Superalloy and Ti 7 wt%Al (Ti-7Al) are predicted as example face-centered cubic and hexagonal closed packed alloys, respectively. A transfer learning approach is taken in which GNN surrogate models are trained using crystal elasticity finite element method (CEFEM) simulations and then the trained surrogate models are used to predict the mechanical response of microstructures measured using high-energy X-ray diffraction microscopy (HEDM). The performance of using various microstructural and micromechanical descriptors for input nodal features to the GNNs is explored through comparisons to traditional mean-field theory predictions, reserved full-field CEFEM data, and measured far-field HEDM data. The effects of elastic anisotropy on GNN model performance and outlooks for the extension of the framework are discussed.
更多查看译文
关键词
graph neural network modeling,elastic behavior,measured microscale data,grain-scale
AI 理解论文
溯源树
样例
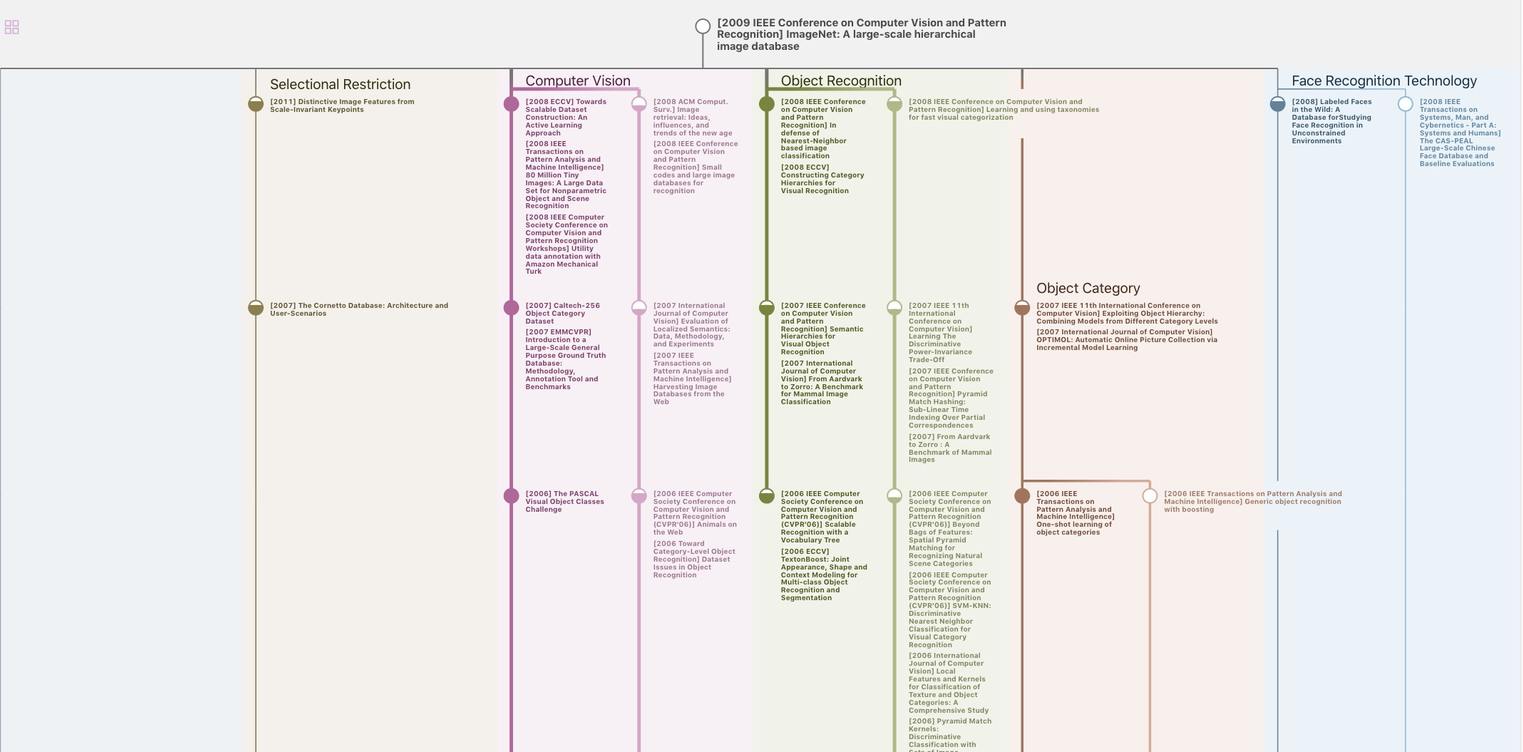
生成溯源树,研究论文发展脉络
Chat Paper
正在生成论文摘要