Convergence of Deep Neural Networks with General Activation Functions and Pooling
arxiv(2022)
摘要
Deep neural networks, as a powerful system to represent high dimensional complex functions, play a key role in deep learning. Convergence of deep neural networks is a fundamental issue in building the mathematical foundation for deep learning. We investigated the convergence of deep ReLU networks and deep convolutional neural networks in two recent researches (arXiv:2107.12530, 2109.13542). Only the Rectified Linear Unit (ReLU) activation was studied therein, and the important pooling strategy was not considered. In this current work, we study the convergence of deep neural networks as the depth tends to infinity for two other important activation functions: the leaky ReLU and the sigmoid function. Pooling will also be studied. As a result, we prove that the sufficient condition established in arXiv:2107.12530, 2109.13542 is still sufficient for the leaky ReLU networks. For contractive activation functions such as the sigmoid function, we establish a weaker sufficient condition for uniform convergence of deep neural networks.
更多查看译文
关键词
deep neural networks,neural networks,general activation functions
AI 理解论文
溯源树
样例
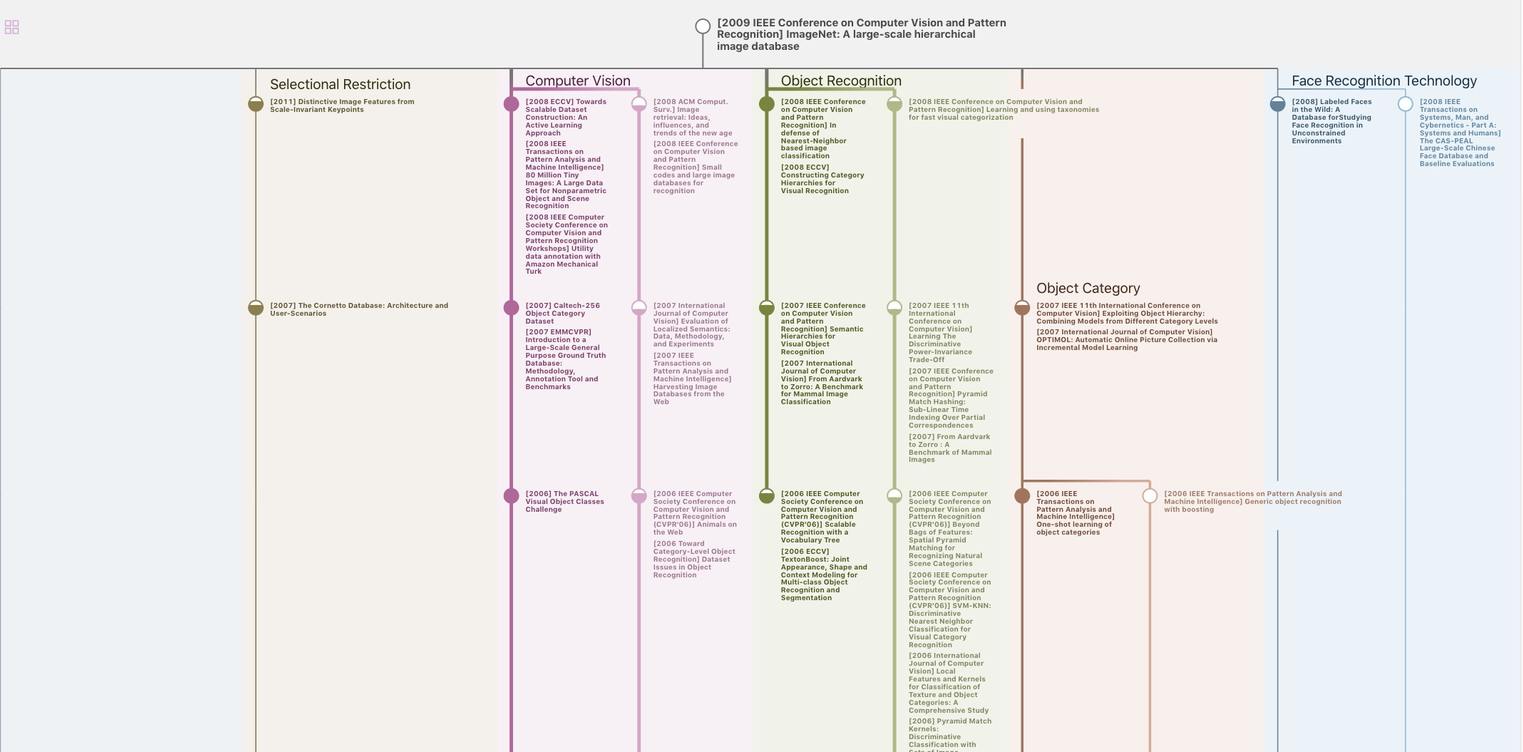
生成溯源树,研究论文发展脉络
Chat Paper
正在生成论文摘要