Segmenting quantitative phase images of neurons using a deep learning model trained on images generated from a neuronal growth model
Quantitative Phase Imaging VIII(2022)
摘要
Morphological changes in neurons are closely related to neurological disorders. Quantitative Phase Imaging (QPI) has been used to assess neuronal changes over time, using mass-sensitive contrast to quantitatively track network growth. QPI requires high quality segmentation of neurons in order to measure neuron cell body and neurite mass distributions. Neural networks are the state of the art for segmentation, but require thousands of images in order to generalize well. However, recent work on network functionality has shown that networks generalize by learning simple functions. Whether low data complexity hinders this has yet to be seen. Here we test this by simulating low complexity data, specifically, QPI images of neurons simulated using a neuronal growth model. We show segmentation results for feeding the network lab-acquired data.
更多查看译文
AI 理解论文
溯源树
样例
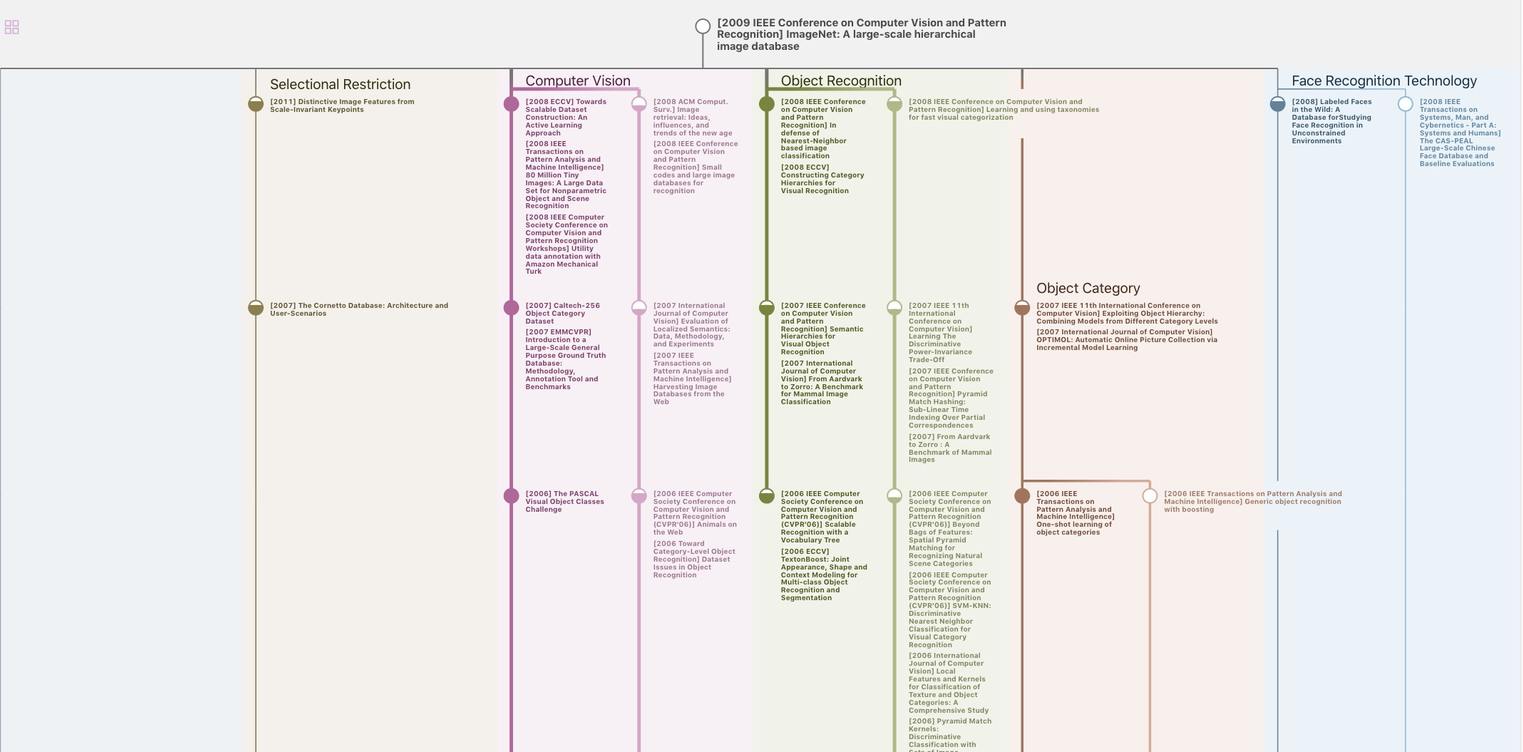
生成溯源树,研究论文发展脉络
Chat Paper
正在生成论文摘要