Energy Efficient Deep Learning Inference Embedded on FPGA for Sleep Apnea Detection
Journal of Signal Processing Systems(2022)
摘要
Sleep apnea is a type of disorder caused by the absence of breathing for a specific period of time coupled with a significant decrease in the blood oxygen saturation level. The monitoring process of sleep apnea is challenging due to the requirement of overnight expensive sleep study, hand-crafted feature extraction from breathing signals, and manual annotations by the sleep experts. Therefore, a low-cost, energy-efficient, portable, and automated biomedical system is necessary to improve early detection, frequent monitoring, and clinical decision-making. In this paper, a digital hardware design of a trained deep feedforward neural network (FNN) is implemented on a Field Programmable Gate-Array (FPGA) for the detection of sleep apnea. The model was trained and evaluated with hyperparameters obtained from a three-step optimization process which ensures compact design solution in low-power miniaturized CMOS circuits. A three-layer FNN trained with ADAM optimizer and mean square error (MSE) loss minimization shows an accuracy of around 88%. An application-specific deep learning inference module realized in FPGA hardware platform confirms a power consumption below 34 W which is 5 × lower than that of commercially available machine learning accelerators. The outcome of this research can be integrated into a system-on-a-chip (SoC) platform for developing a smart automated sleep apnea detection device.
更多查看译文
关键词
Deep learning,Feedforward neural network,FPGA,Sleep apnea,ECG,Oxygen saturation,System-on-a-chip
AI 理解论文
溯源树
样例
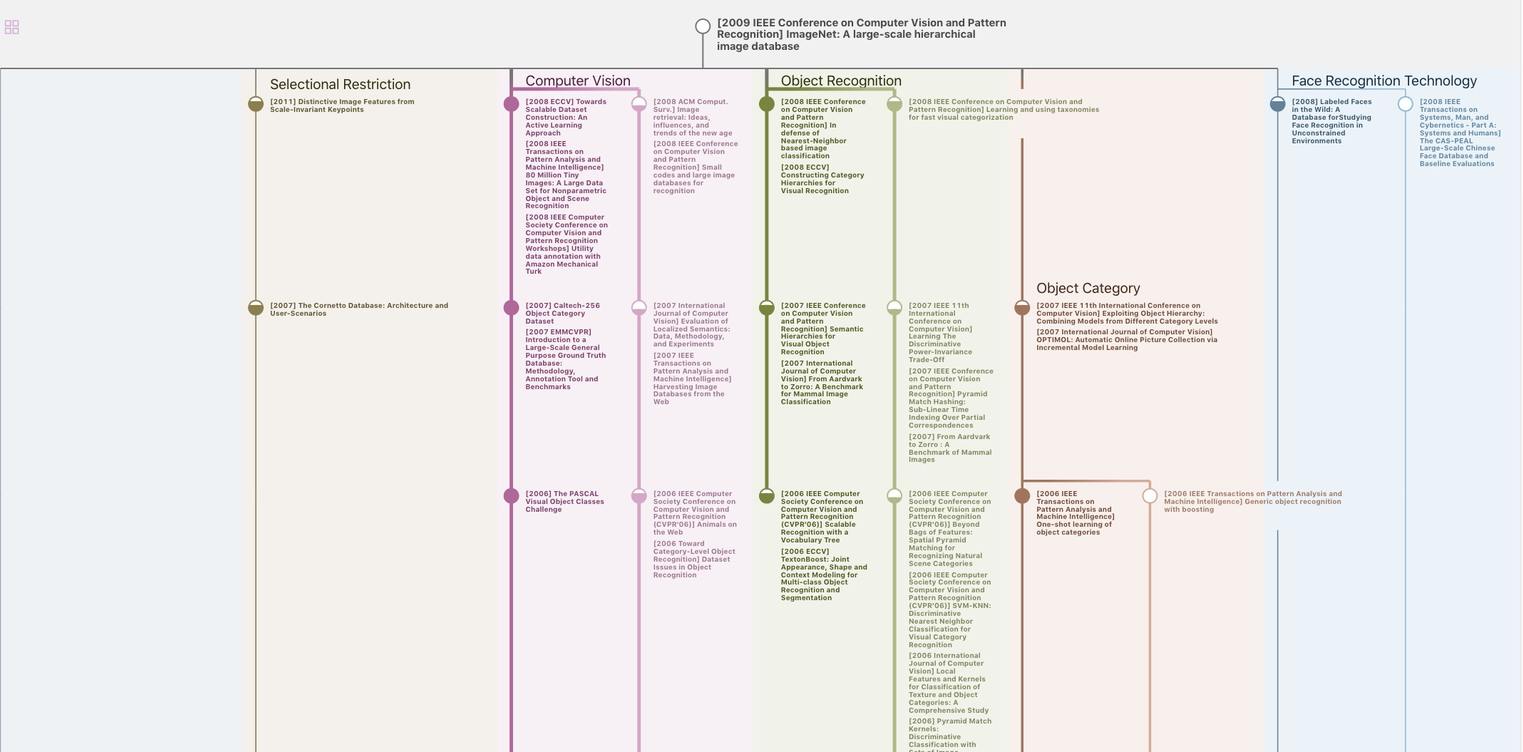
生成溯源树,研究论文发展脉络
Chat Paper
正在生成论文摘要