Improving Target-driven Visual Navigation with Attention on 3D Spatial Relationships
NEURAL PROCESSING LETTERS(2022)
摘要
Embodied Artificial Intelligence has become popular in recent years. Its task shifts from focusing on internet images to active settings, involving an embodied agent to perceive and act within 3D environments. In this paper, we study the Target-driven Visual Navigation (TDVN) in 3D indoor scenes using deep reinforcement learning techniques. The generalization of TDVN is a long-standing ill-posed issue, where the agent is expected to transfer intelligent knowledge from training domains to unseen domains. To address this issue, we propose a model that combines visual and relational graph features to learn the navigation policy. Graph convolutional networks are used to obtain graph features, which encodes spatial relations between objects. We also adopt a Target Skill Extension module to generate sub-targets, in order to allow the agent to learn from its failures. For evaluation, we perform experiments in the AI2-THOR. Experimental results show that our proposed model outperforms baselines under various metrics.
更多查看译文
关键词
Deep reinforcement learning,Graph convolutional networks,Visual navigation,3D scenes
AI 理解论文
溯源树
样例
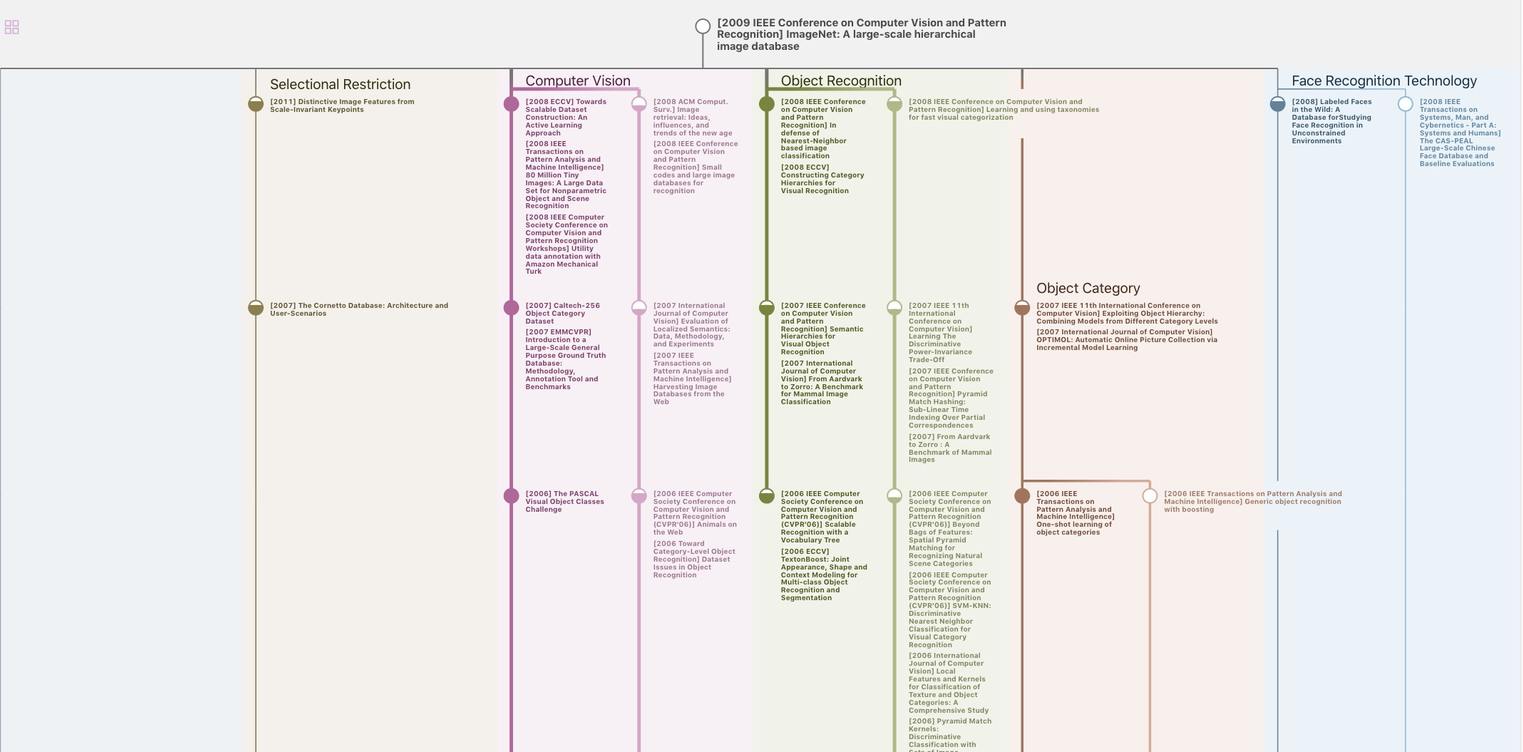
生成溯源树,研究论文发展脉络
Chat Paper
正在生成论文摘要