Complexity Is an Effective Observable to Tune Early Stopping in Scenario Optimization
IEEE Transactions on Automatic Control(2023)
摘要
Scenario optimization is a broad scheme for data-driven decision-making in which experimental observations act as constraints on the feasible domain for the optimization variables. The probability with which the solution is not feasible for a new, out-of-sample, observation is called the “risk.” Recent studies have unveiled the profound link that exists between the risk and a properly defined notion of “complexity” of the scenario solution. In the present article, we leverage these results to introduce a new scheme where the size of the sample of scenarios is iteratively tuned to the current complexity of the solution so as to eventually hit a desired level of risk. This new scheme implies a substantial saving of data as compared to previous approaches. This article presents the new method, offers a full theoretical study, and illustrates it on a control problem.
更多查看译文
关键词
Optimization under uncertainties,randomized methods,scenario optimization
AI 理解论文
溯源树
样例
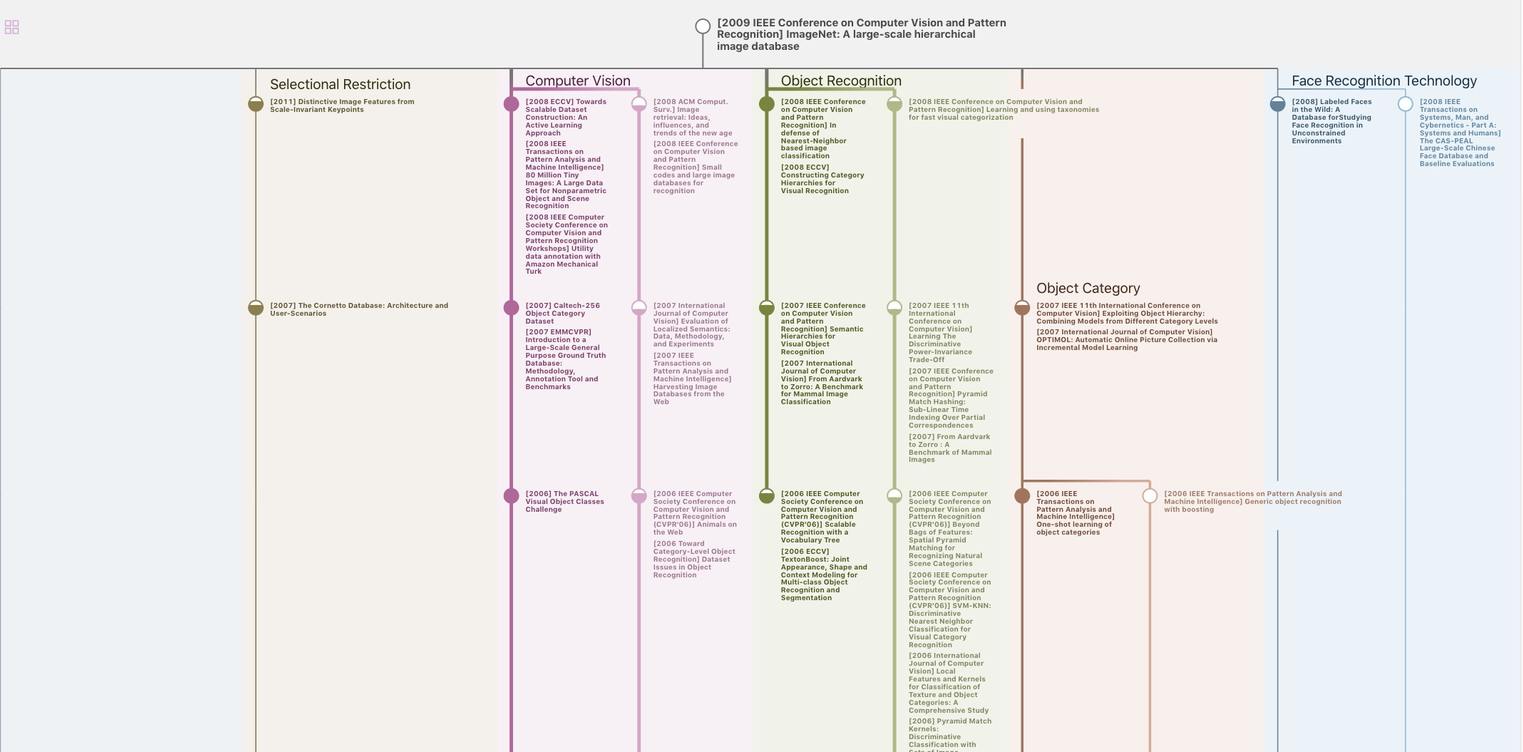
生成溯源树,研究论文发展脉络
Chat Paper
正在生成论文摘要