Distribution modelling of deep-sea elasmobranchs on the Mid-Atlantic Ridge for spatial management
Deep-sea Research Part I-oceanographic Research Papers(2022)
摘要
Elasmobranchs inhabiting depths beyond 200 m are extremely susceptible to overexploitation but are extracted by fisheries around the world either as target species or as bycatch. There is little information available to formulate management strategies to reduce elasmobranch-fishery interactions in the deep sea. In European Union waters, prohibiting the catches of deep-sea elasmobranchs has provided the necessary impetus to study by-catch avoidance of these threatened species. We used over 20 years of fisheries-independent and fisheries-dependent data to model the spatial distribution of 15 species of deep-sea elasmobranchs (12 sharks and 3 rays) captured frequently in the Exclusive Economic Zone of the Azores Archipelago (Mid-Atlantic Ridge) to explore spatial management to reduce unwanted catches of these species. We applied Generalised Additive Models to predict the probability of presence of 15 species, as well as the abundance of 6 of those species, within the Azores EEZ and neighbouring seamounts (up to 2000 m depth), using environmental and operational variables as predictors. Our results identified that depth is most influential in determining the distribution of these sharks and rays, in addition to seafloor topography. Distinctive bathymetric features such as seamounts and ridges were highlighted as areas where the probability of presence of the greatest number of species overlapped. Although not related to habitat, gear type influenced the capture probability of certain species, with the artisanal handline, gorazeira , having lower captures than bottom longline. Our results support using depth-based, area-based, and gear-based tactics to design management measures to reduce elasmobranch bycatch, for more sustainable deep-sea fisheries. • Most frequently captured deep-sea elasmobranchs modelled in the Azores using delta GAMs. • Spatial distribution predictions can be used to reduce bycatch of 15 sharks and rays. • Depth and seafloor topography important predictors. Lower catch on handline than longline. • Maximum species richness predicted around seamounts and ridges. • Inform area and gear-based management that can balance species conservation and fisheries.
更多查看译文
AI 理解论文
溯源树
样例
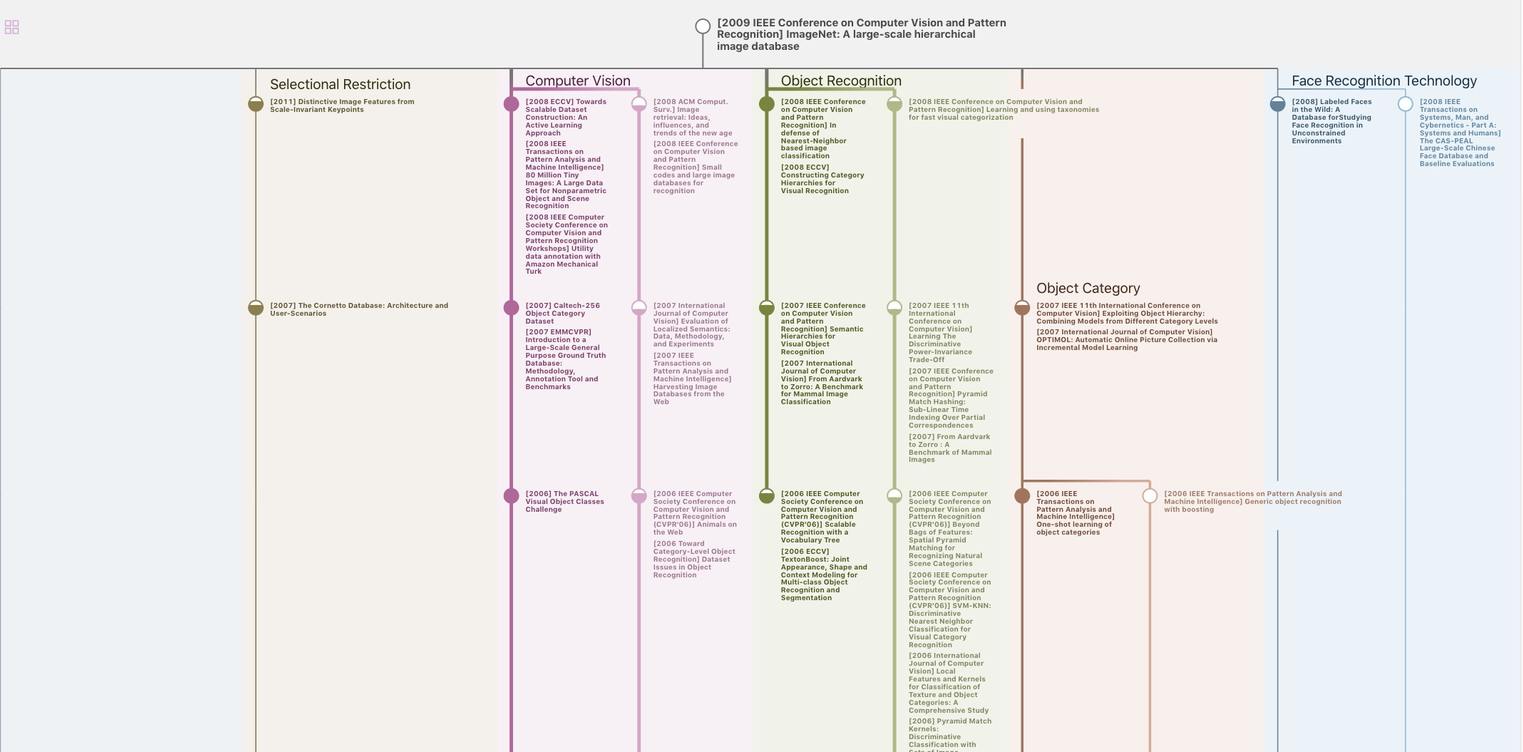
生成溯源树,研究论文发展脉络
Chat Paper
正在生成论文摘要