Internal Calibration of Transient Kinetic Data via Machine Learning
Catalysis Today(2022)
摘要
The temporal analysis of products (TAP) reactor provides a vast amount of transient kinetic information that may be used to describe a variety of chemical features including residence time distributions, kinetic coefficients, number of active sites, reaction mechanism, etc. However, as with any measurement device, the TAP reactor signal is convoluted with noise and drift is common. To reduce the uncertainty of the kinetic measurement and any derived parameters or mechanisms, proper preprocessing must be performed prior to any advanced type of analysis. This preprocessing includes baseline correction, i.e., a shift in the voltage response, and calibration, i.e., a scaling of the flux response based on prior experiments. The traditional methodology of preprocessing requires significant user discretion and reliance on separate calibration experiments that may drift over time. Herein we use machine learning techniques combined with physical constraints to understand the noise and drift that is being generated within and between experiments for enhancement of the chemical kinetic signal. As such, the proposed methodology demonstrates clear benefits over the traditional preprocessing approach by eliminating the need for separate calibration experiments or heuristic input from the user. • A new analysis method eliminates the need for separate calibration experiments. • The Gamma distribution, machine learning and physical constraints isolate the kinetic signal. • Noise and drift over a series of experiments are accounted for. • The uncertainty of kinetic measurements is reduced.
更多查看译文
关键词
Transient Kinetics,TAP,Cheminformatics,Calibration,Convex optimization
AI 理解论文
溯源树
样例
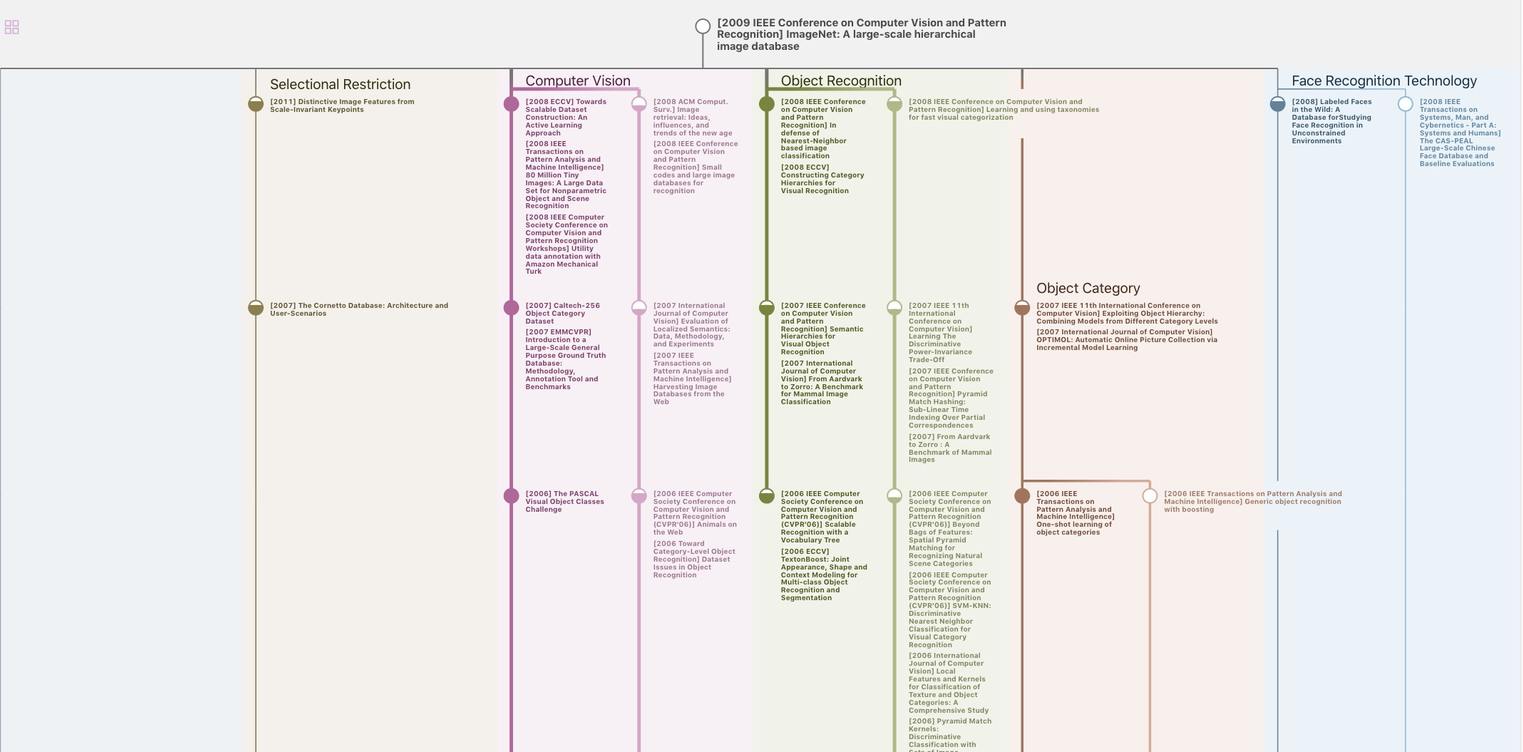
生成溯源树,研究论文发展脉络
Chat Paper
正在生成论文摘要