Consistent Discrepancy Learning for Intra-Camera Supervised Person Re-Identification
IEEE Transactions on Multimedia(2023)
摘要
Since annotating pedestrians across different views is extremely costly, intra-camera supervised person re-identification (ReID) aims to learn a ReID model from the intra-view labeled data. Under this setting, the most challenge lies in learning a view-invariant feature embedding in the absence of the cross-view annotations. Previous works focus on assigning a pseudo identity label for each image based on the feature similarity and learn view-invariant features by classification loss. However, because of the cross-view variations in lighting, background, etc., the pseudo labels are often noisy, and therefore not reliable for classification. In this paper, we explore learning a consistent discrepancy for pairwise images. Our main idea is that the discrepancy between pedestrian images should be consistent across different views regardless of view change so that it mainly depicts the identity difference. Due to the lack of cross-view annotations, we project images into different views and obtain likelihood prototypes for cross-view learning. These likelihood prototypes are used to measure the discrepancies between pairwise images under different views. And then, we propose an intra-view discrepancy preservation module to enforce the discrepancy to be view-consistent so as to encourage the model to distinguish the images based on the identities regardless of view change. Extensive experiments on multiple datasets show that our method outperforms existing related methods by clear margins and our method is comparable to supervised counterparts. Code will be made publicly available.
更多查看译文
关键词
Intra-camera supervised,likelihood prototypes,person re-identification
AI 理解论文
溯源树
样例
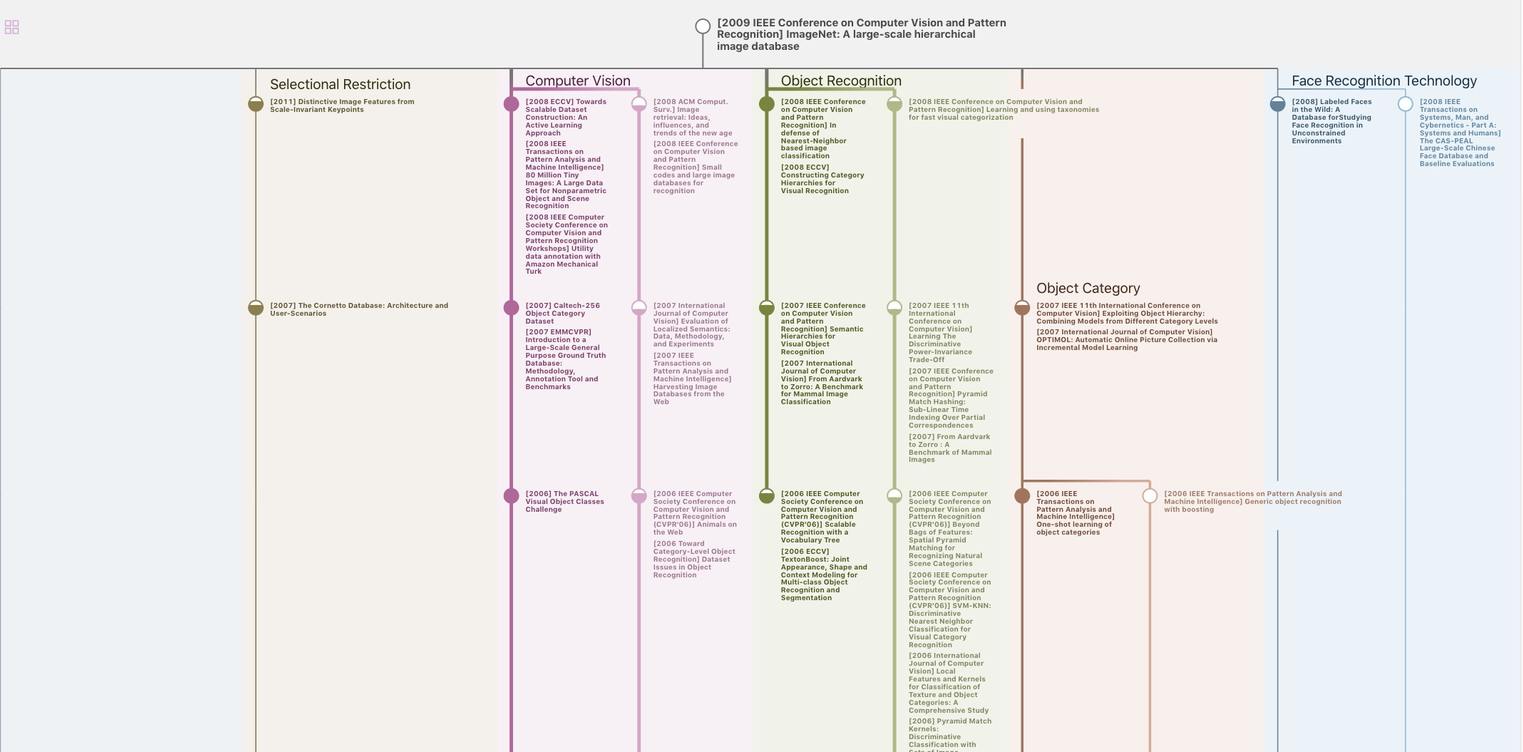
生成溯源树,研究论文发展脉络
Chat Paper
正在生成论文摘要