GMR-RRT*: Sampling-Based Path Planning Using Gaussian Mixture Regression
IEEE Transactions on Intelligent Vehicles(2022)
摘要
Mobile robot autonomous path planning is an essential factor for its wide deployment in real-world applications. Conventional sampling-based algorithms have gained tremendous success in the path planning field, but they usually take much time to find the optimal solution so that the planning quality (evaluated with time cost and path length) cannot be guaranteed. In this paper, based on Gaussian Mixture Regression (GMR) and the family of Rapidly-exploring Random Tree (RRT) schemes, we propose the GMR-RRT* algorithm to achieve fast path planning for mobile robots. The proposed GMR-RRT* consists of learning navigation behaviors from human demonstrations and planning a high-quality path for the robot. Using the GMR, the key features of human demonstrations are captured to form a probability density distribution of the human trajectory in the current environment. This distribution is further utilized to guide the RRT scheme’s sampling process to generate a feasible path in the current environment quickly. We test the proposed GMR-RRT* in different environments, comparing it with three state-of-the-art sampling-based algorithms. The experimental results demonstrate that the GMR-RRT* algorithm can achieve better performance in terms of time cost, memory usage, and path length.
更多查看译文
关键词
Mobile robot,learning from human demonstrations,sampling-based path planning
AI 理解论文
溯源树
样例
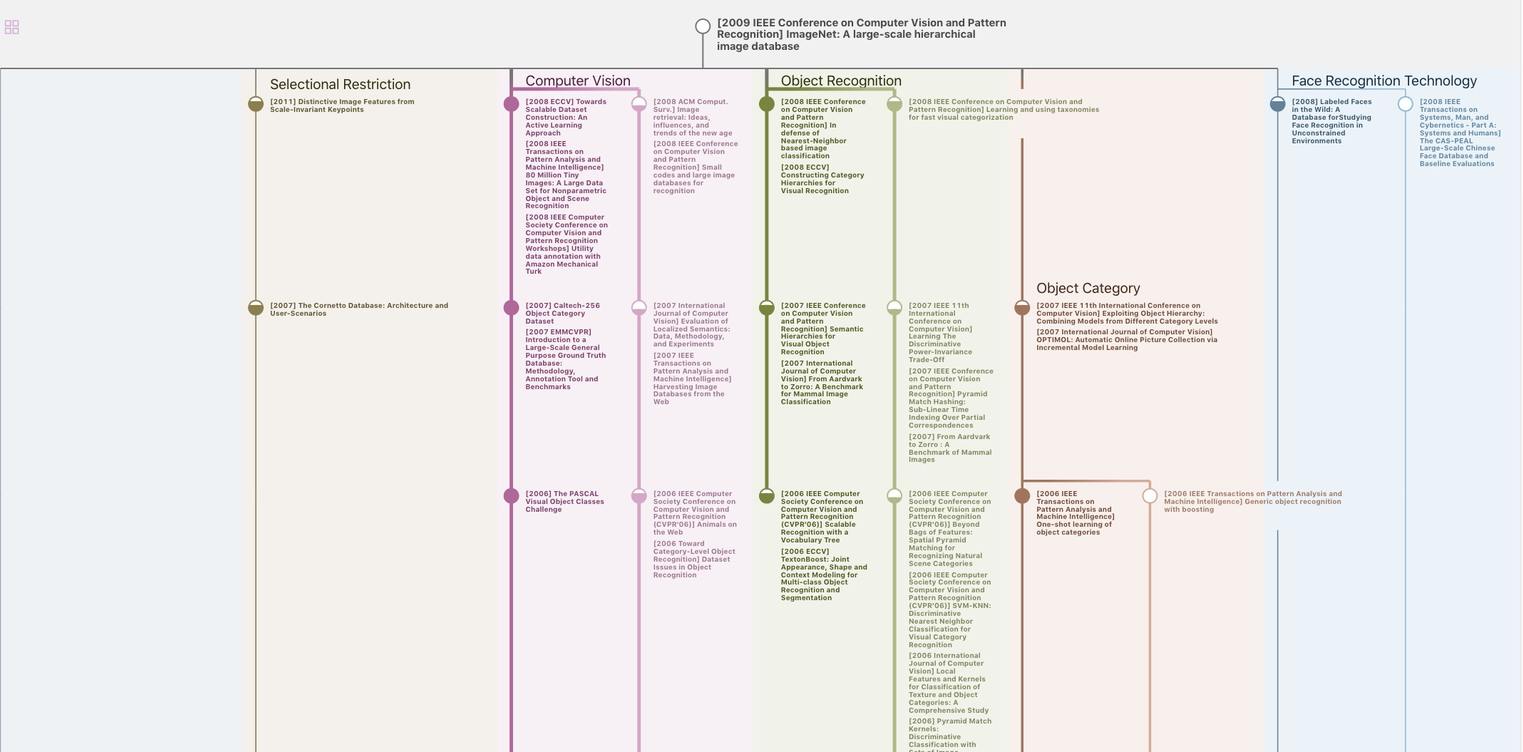
生成溯源树,研究论文发展脉络
Chat Paper
正在生成论文摘要