Ultra-Short-Term Prediction of EV Aggregator’s Demond Response Flexibility Using ARIMA, Gaussian-ARIMA, LSTM and Gaussian-LSTM
2021 3rd International Academic Exchange Conference on Science and Technology Innovation (IAECST)(2021)
摘要
The rapid growth of electric vehicles and their controllability bring the electric vehicle aggregator (EVA) more and more opportunities to participate in the real-time energy and regulation markets. The EVA needs to guarantee the prediction accuracy of its base power rate and demand response flexibility to benefit from energy arbitrage and ancillary services. The prediction of EVA’s demand response flexibility is based on the accurate power load prediction. Due to the high volatility and uncertainty, it is pretty challenging to make the ultra-short-term prediction of EVA’s power consumption. The purpose of this paper is to design a feasible and accurate method for an actual EVA to predict its base power and flexibility one hour and 15 minutes ahead considering the necessity of a Gaussian filter. In this paper, the comparative study of the two main prediction methods ARIMA and LSTM is carried out to find out the more appropriate solution for the ultra-short-term prediction with the step of one hour and 15 minutes. Considering the non-linear parts involved in the historical data, the traditional ARIMA and LSTM are combined with a Gaussian filter for comparison. Therefore, the ARIMA, Gaussian-ARIMA, LSTM and Gaussian-LSTM approaches are analyzed and compared by backtesting procedure from the historical load data of an existing EVA integrating 15577 EV charging piles participates in a specific province in China. As a result, the LSTM approach outperforms the ARIMA according to the backtesting results. The Gaussian filter can improve the prediction performance under the condition of low data density or obvious noises.
更多查看译文
关键词
utra-short-term prediction,EVA,demand response,flexibility,ARIMA,LSTM,Gaussian filter
AI 理解论文
溯源树
样例
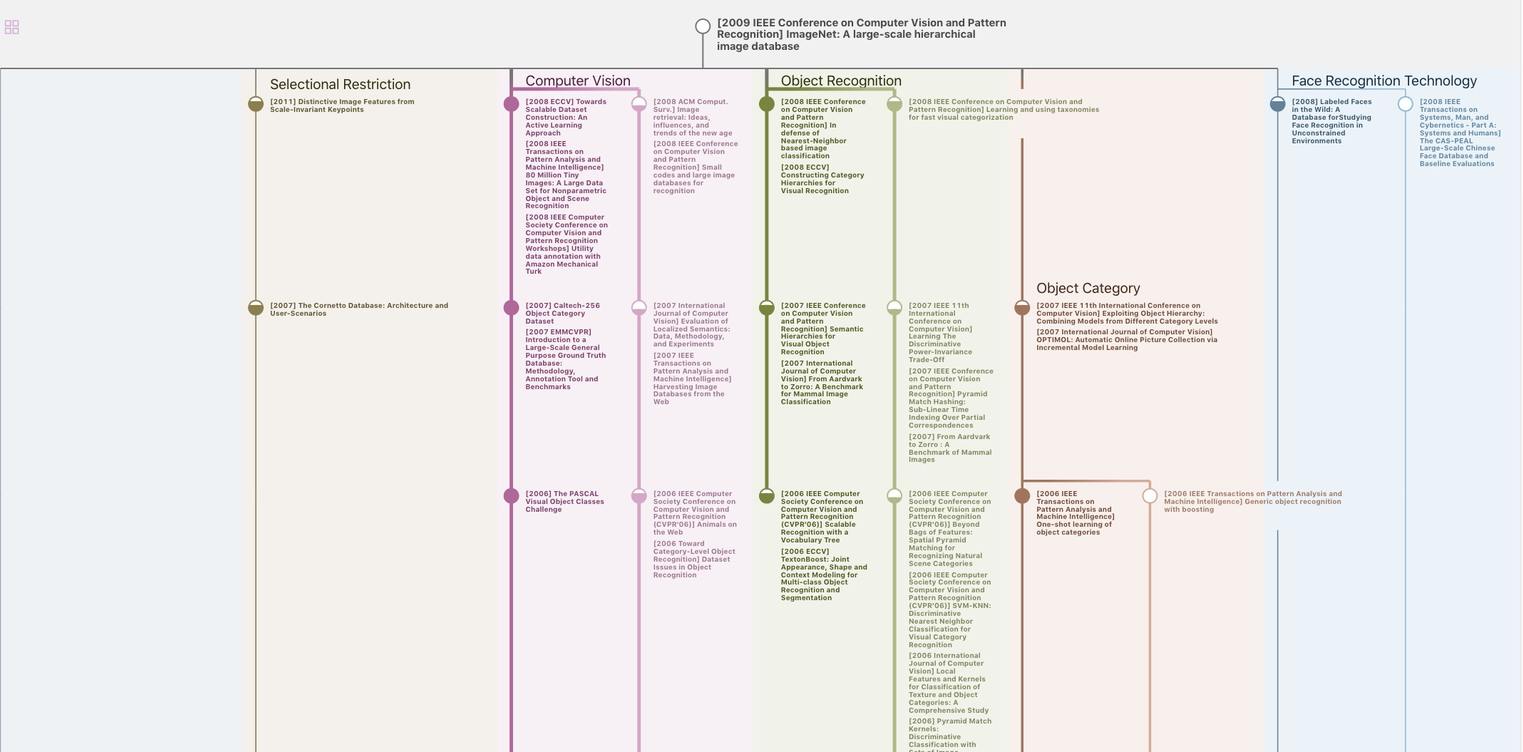
生成溯源树,研究论文发展脉络
Chat Paper
正在生成论文摘要