Multi-task deep learning seismic impedance inversion optimization based on homoscedastic uncertainty
Applied sciences(2022)
摘要
Seismic inversion is a process to obtain the spatial structure and physical properties of underground rock formations by using surface acquired seismic data, constrained by known geological laws, drilling and logging data. The principle of seismic inversion based on deep learning is to learn the mapping between seismic data and rock properties by training a neural network using logging data as labels. However, due to high cost, the number of logging curves are often limited, leading to a trained model with poor generalization. Multi-task learning (MTL) provides an effective way to mitigate this problem. Learning multiple related tasks at the same time can improve the generalization ability of the model, thereby improving the performance of the main task on the same amount of labeled data. However, the performance of multi-task learning is highly dependent on the relative weights for the loss of each task, and manual tuning of the weights is often time-consuming and laborious. In this paper, a method based on homoscedastic uncertainty of the Bayesian model is used to balance the weights of the loss function for multiple tasks, and a Fully convolutional residual network (FCRN) is used to achieve seismic impedance inversion and seismic data reconstruction simultaneously. The test results on the synthetic dataset of Marmousi2 model show that the proposed method can automatically determine the approximate optimal weight of the two tasks, and predicts impedance with higher accuracy than single-task FCRN model.
更多查看译文
关键词
seismic impedance inversion,fully convolutional residual network,multi-task learning,homoscedastic uncertainty
AI 理解论文
溯源树
样例
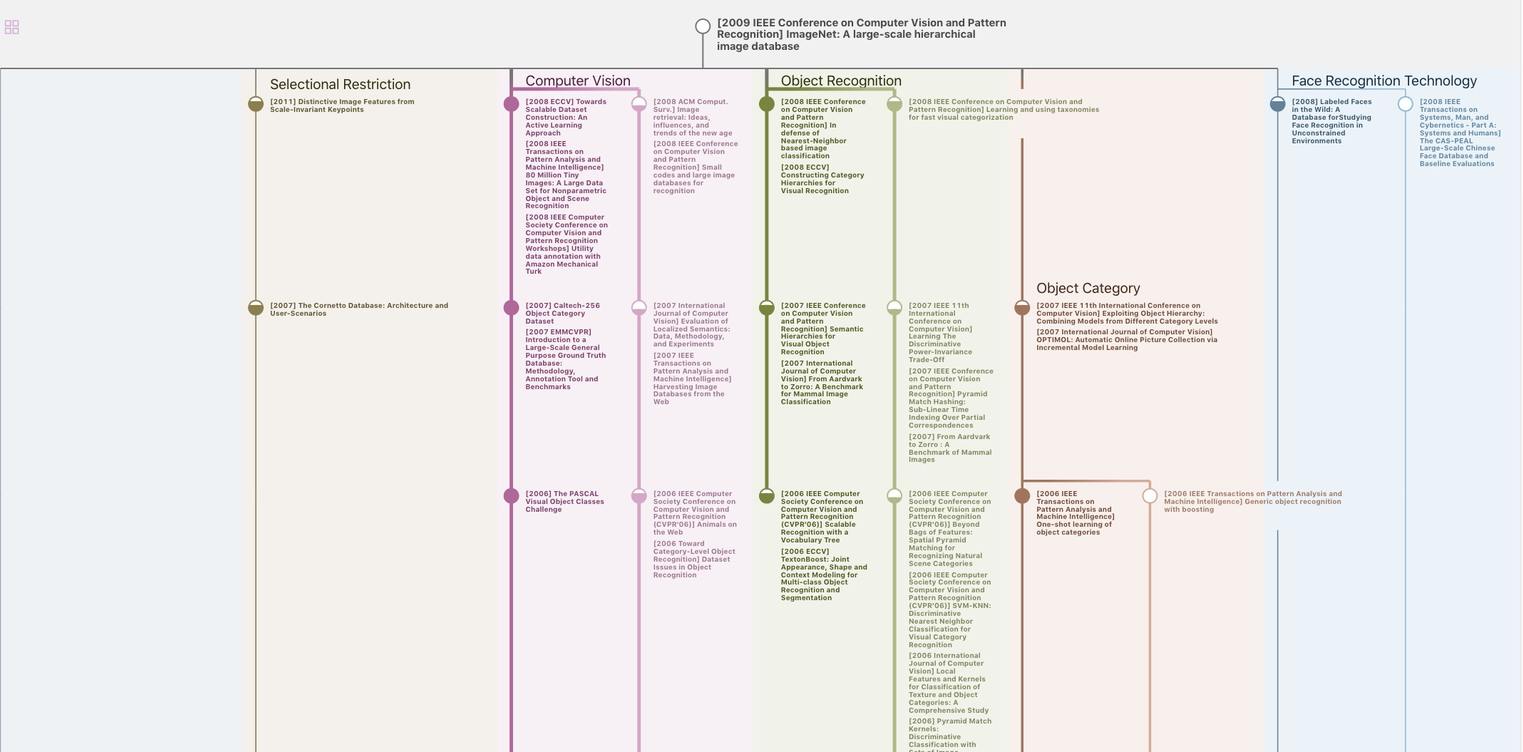
生成溯源树,研究论文发展脉络
Chat Paper
正在生成论文摘要