PathologyBERT -- Pre-trained Vs. A New Transformer Language Model for Pathology Domain
arxiv(2022)
摘要
Pathology text mining is a challenging task given the reporting variability and constant new findings in cancer sub-type definitions. However, successful text mining of a large pathology database can play a critical role to advance 'big data' cancer research like similarity-based treatment selection, case identification, prognostication, surveillance, clinical trial screening, risk stratification, and many others. While there is a growing interest in developing language models for more specific clinical domains, no pathology-specific language space exist to support the rapid data-mining development in pathology space. In literature, a few approaches fine-tuned general transformer models on specialized corpora while maintaining the original tokenizer, but in fields requiring specialized terminology, these models often fail to perform adequately. We propose PathologyBERT - a pre-trained masked language model which was trained on 347,173 histopathology specimen reports and publicly released in the Huggingface repository. Our comprehensive experiments demonstrate that pre-training of transformer model on pathology corpora yields performance improvements on Natural Language Understanding (NLU) and Breast Cancer Diagnose Classification when compared to nonspecific language models.
更多查看译文
关键词
pathologybert domain,new transformer language model,pre-trained
AI 理解论文
溯源树
样例
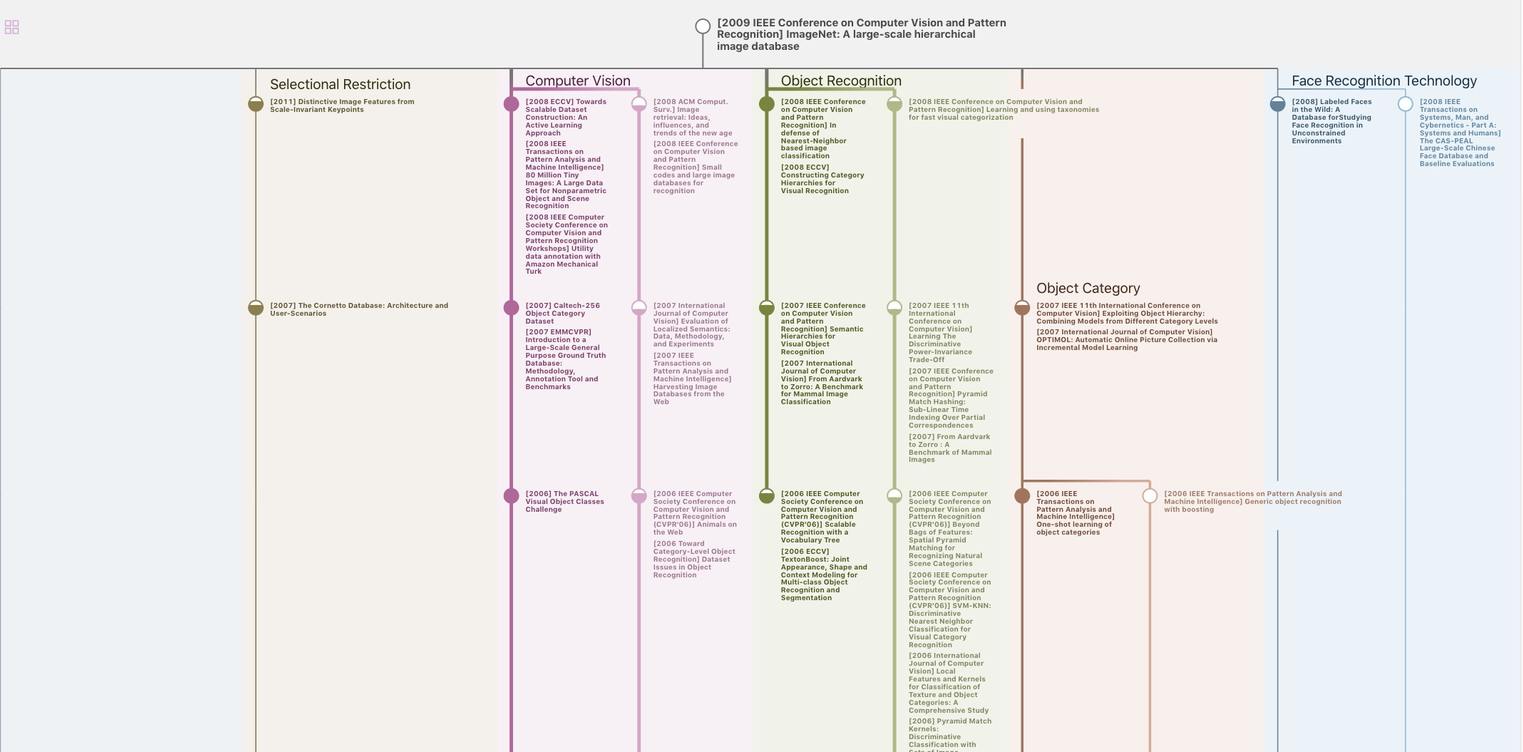
生成溯源树,研究论文发展脉络
Chat Paper
正在生成论文摘要