Transformer Scale Gate for Semantic Segmentation
CVPR 2023(2023)
Abstract
Effectively encoding multi-scale contextual information is crucial for accurate semantic segmentation. Most of the existing transformer-based segmentation models combine features across scales without any selection, where features on sub-optimal scales may degrade segmentation outcomes. Leveraging from the inherent properties of Vision Transformers, we propose a simple yet effective module, Transformer Scale Gate (TSG), to optimally combine multi-scale features. TSG exploits cues in self and cross attentions in Vision Transformers for the scale selection. TSG is a highly flexible plug-and-play module, and can easily be incorporated with any encoder-decoder-based hierarchical vision Transformer architecture. Extensive experiments on the Pascal Context, ADE20K and Cityscapes datasets demonstrate that our feature selection strategy achieves consistent gains.
MoreTranslated text
Key words
Segmentation,grouping and shape analysis
AI Read Science
Must-Reading Tree
Example
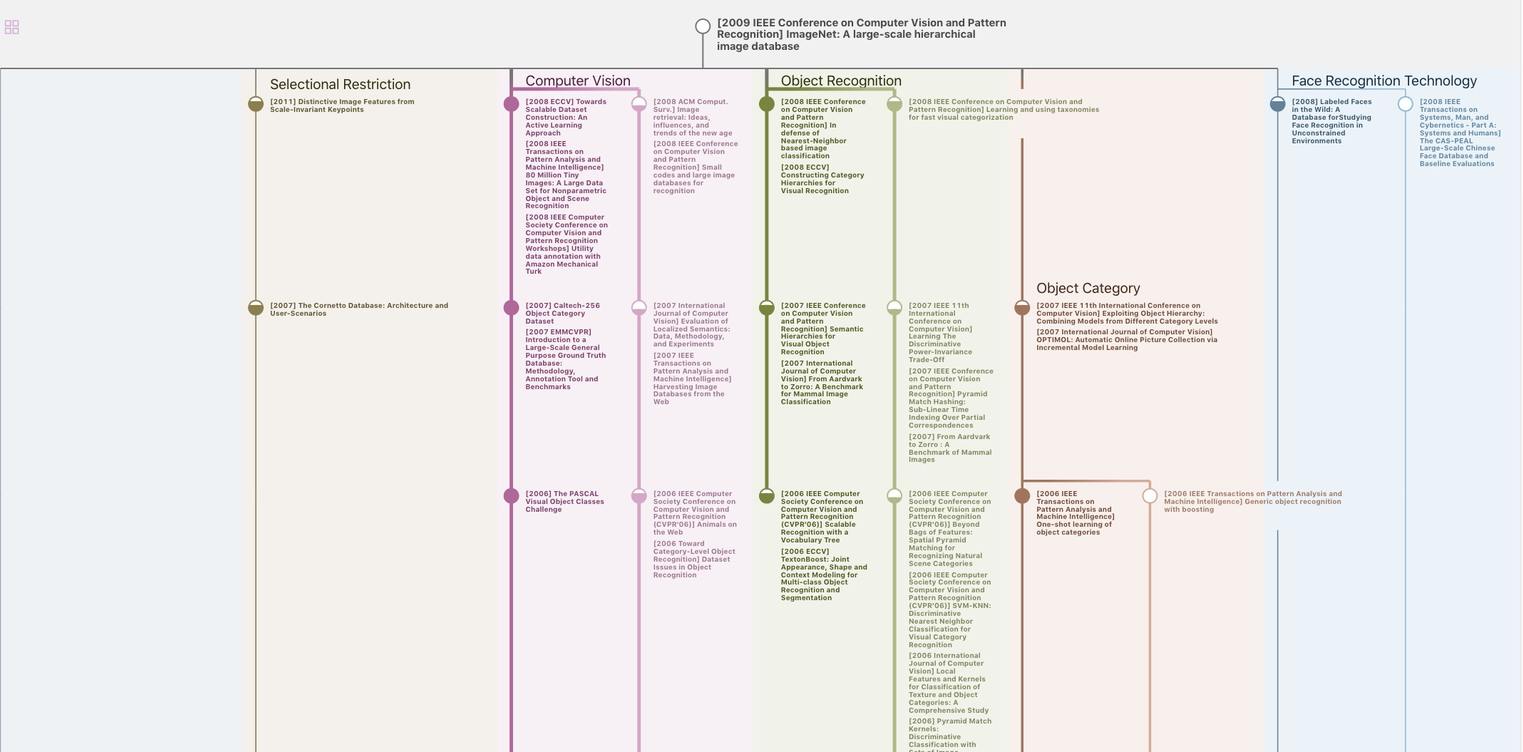
Generate MRT to find the research sequence of this paper
Chat Paper
Summary is being generated by the instructions you defined