Collar-Aware Training for Streaming Speaker Change Detection in Broadcast Speech.
The Speaker and Language Recognition Workshop (Odyssey)(2022)
摘要
In this paper, we present a novel training method for speaker change detection models. Speaker change detection is often viewed as a binary sequence labelling problem. The main challenges with this approach are the vagueness of annotated change points caused by the silences between speaker turns and imbalanced data due to the majority of frames not including a speaker change. Conventional training methods tackle these by artificially increasing the proportion of positive labels in the training data. Instead, the proposed method uses an objective function which encourages the model to predict a single positive label within a specified collar. This is done by marginalizing over all possible subsequences that have exactly one positive label within the collar. Experiments on English and Estonian datasets show large improvements over the conventional training method. Additionally, the model outputs have peaks concentrated to a single frame, removing the need for post-processing to find the exact predicted change point which is particularly useful for streaming applications.
更多查看译文
关键词
streaming speaker change detection,broadcast speech,collar-aware
AI 理解论文
溯源树
样例
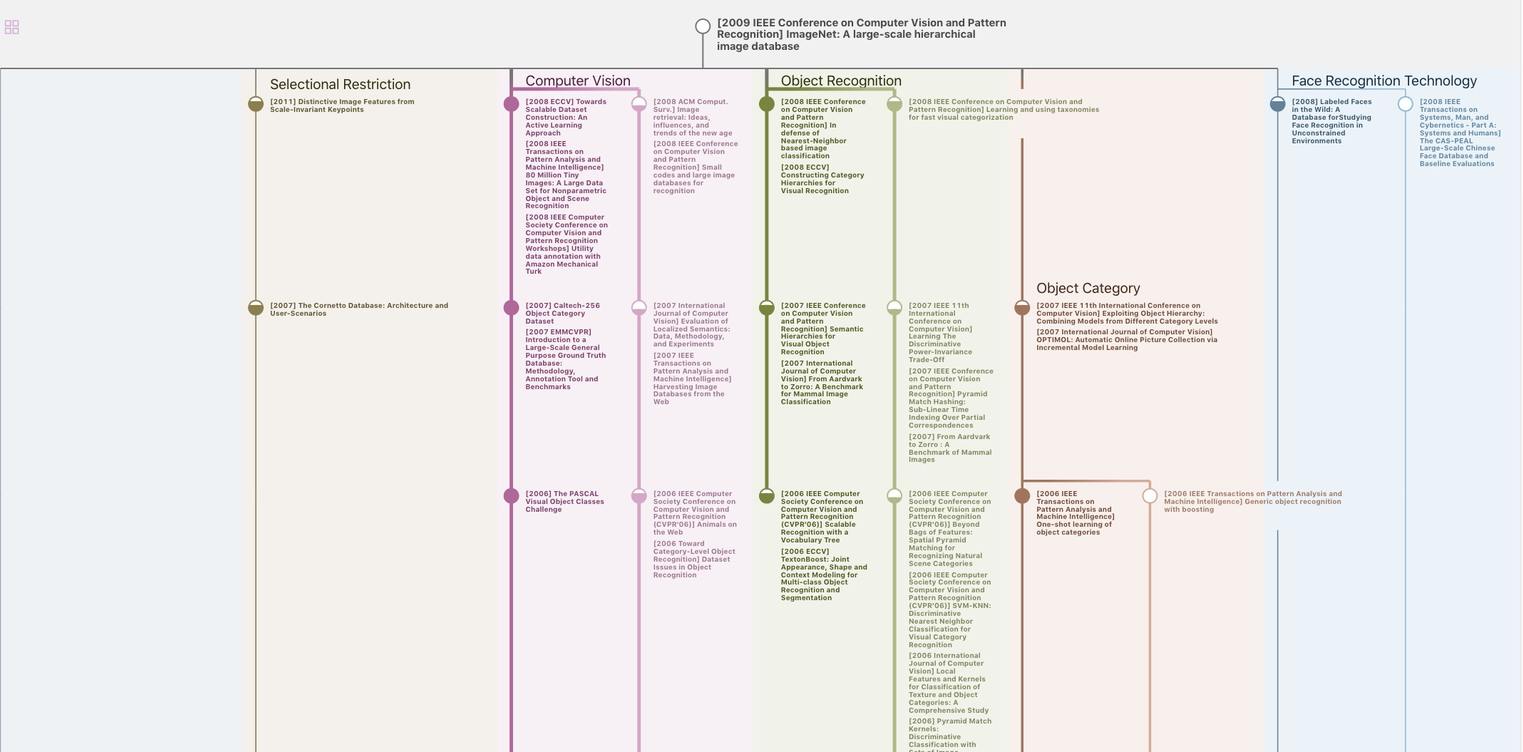
生成溯源树,研究论文发展脉络
Chat Paper
正在生成论文摘要