Variational learning algorithms for quantum query complexity
NEW JOURNAL OF PHYSICS(2024)
摘要
Quantum query complexity is pivotal in the analysis of quantum algorithms, encompassing well-known examples like search and period-finding algorithms. These algorithms typically involve a sequence of unitary operations and oracle calls dependent on an input variable. In this study, we introduce a variational learning approach to explore quantum query complexity. Our method employs an efficient parameterization of the unitary operations and utilizes a loss function derived from the algorithm's error probability. We apply this technique to various quantum query complexities, notably devising a new algorithm that resolves the 5-bit Hamming modulo problem with four queries, addressing an open question from Cornelissen et al (2021 arXiv:2112.14682). This finding is corroborated by a semidefinite programming (SDP) approach. Our numerical method exhibits superior memory efficiency compared to SDP and can identify quantum query algorithms (QQAs) that require a smaller workspace register dimension, an aspect not optimized by SDP. These advancements present a significant step forward in the practical application and understanding of QQAs.
更多查看译文
关键词
quantum query complexity,variational algorithm,semidefinite programming
AI 理解论文
溯源树
样例
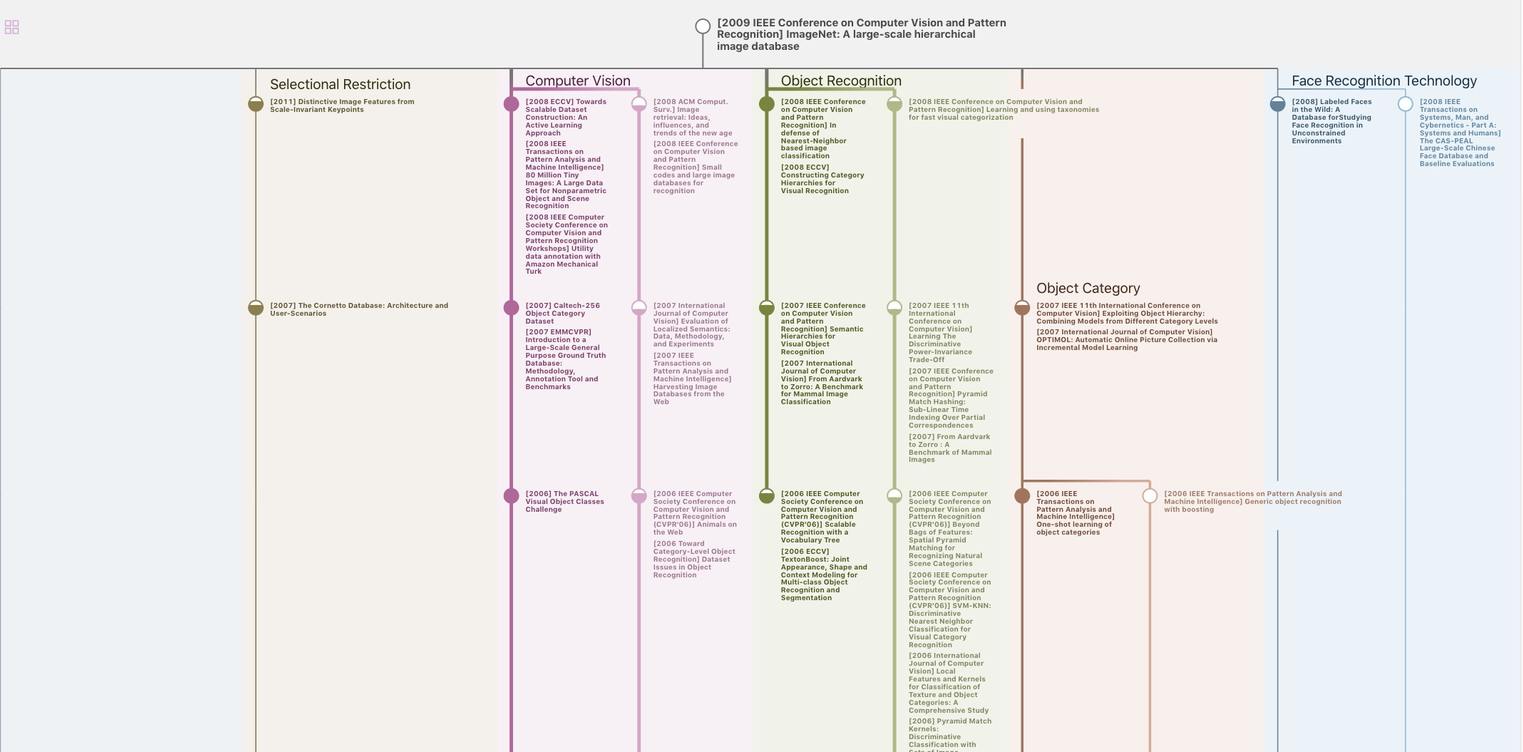
生成溯源树,研究论文发展脉络
Chat Paper
正在生成论文摘要