Gradient Descent Optimizes Infinite-Depth ReLU Implicit Networks with Linear Widths
arxiv(2022)
摘要
Implicit deep learning has recently become popular in the machine learning community since these implicit models can achieve competitive performance with state-of-the-art deep networks while using significantly less memory and computational resources. However, our theoretical understanding of when and how first-order methods such as gradient descent (GD) converge on \textit{nonlinear} implicit networks is limited. Although this type of problem has been studied in standard feed-forward networks, the case of implicit models is still intriguing because implicit networks have \textit{infinitely} many layers. The corresponding equilibrium equation probably admits no or multiple solutions during training. This paper studies the convergence of both gradient flow (GF) and gradient descent for nonlinear ReLU activated implicit networks. To deal with the well-posedness problem, we introduce a fixed scalar to scale the weight matrix of the implicit layer and show that there exists a small enough scaling constant, keeping the equilibrium equation well-posed throughout training. As a result, we prove that both GF and GD converge to a global minimum at a linear rate if the width $m$ of the implicit network is \textit{linear} in the sample size $N$, i.e., $m=\Omega(N)$.
更多查看译文
关键词
gradient,networks,linear widths,infinite-depth
AI 理解论文
溯源树
样例
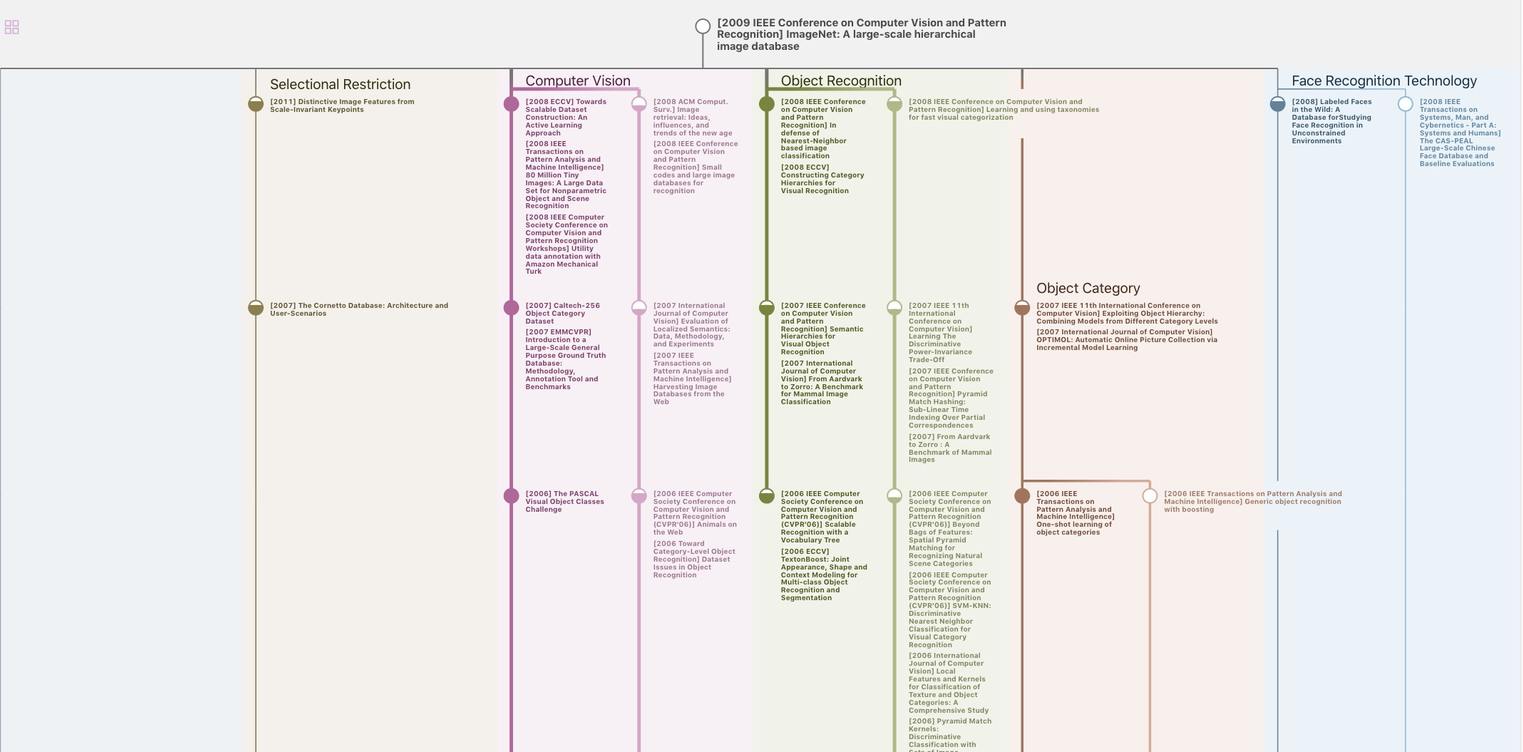
生成溯源树,研究论文发展脉络
Chat Paper
正在生成论文摘要