Literary and Colloquial Tamil Dialect Identification
Circuits, Systems, and Signal Processing(2022)
摘要
Culture and language evolve together. With respect to Tamil, the form of the language that people use nowadays has come a long way from its origins. These days, the old literary form of Tamil is used commonly for writing and the contemporary colloquial Tamil is used for speaking. Human–computer interaction applications require colloquial Tamil (CT) to make it more accessible and easy for the everyday user and, it requires literary Tamil (LT) when information is needed in a formal written format. Continuing the use of LT alongside CT in computer-aided language learning applications will both preserve LT, and provide ease of use via CT, at the same time. Hence, there is a need for the conversion between LT and CT dialects, which demands as a first step, dialect identification. Dialect identification (DID) of LT and CT is an unexplored area of research. There is a considerable research potential in this area, because, (i) LT is standardized while CT is not fully standardized and, (ii) they have only subtle differences. Five methods are explored in our work, which originated from the preliminary work using Gaussian mixture model (GMM) for dialect identification of LT and CT, which offered a motivation with an identification accuracy of 87%. In the current work, keeping the nuances of both these dialects in mind, one other implicit method—convolutional neural network (CNN); two explicit methods—Parallel Phone Recognition (PPR) and Parallel Large Vocabulary Continuous Speech Recognition (P-LVCSR); two versions of the proposed explicit unified phone recognition method (UPR-1 and UPR-2), are explored. These methods vary based on: the need for annotated data, the size of the unit, the way in which modelling is carried out, and the way in which the final decision is made. Even though the average duration of the test utterances is less—4.9 s for LT and 2.5 s for CT—the systems performed well, offering the following identification accuracies: 87.72% (GMM), 93.97% (CNN), 89.24% (PPR), 94.21% (P-LVCSR), 88.57 (UPR-1), 93.53% (UPR-1 with P-LVCSR), 94.55 (UPR-2) and 95.61% (UPR-2 with P-LVCSR).
更多查看译文
关键词
Dialect identification, Literary and colloquial Tamil, Implicit and explicit methods, Statistical machine learning, Neural network
AI 理解论文
溯源树
样例
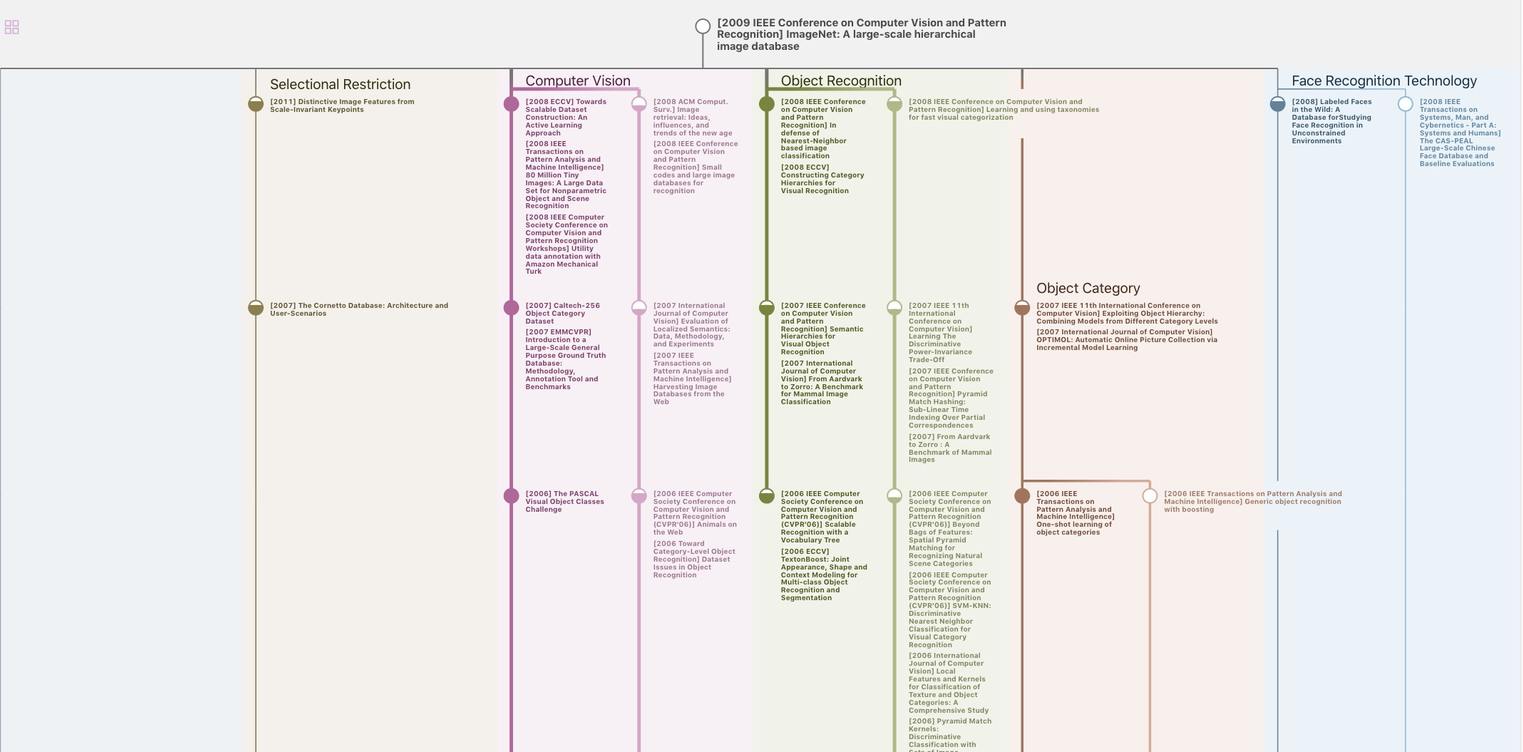
生成溯源树,研究论文发展脉络
Chat Paper
正在生成论文摘要