Functional2Structural: Cross-Modality Brain Networks Representation Learning
arxiv(2022)
摘要
MRI-based modeling of brain networks has been widely used to understand functional and structural interactions and connections among brain regions, and factors that affect them, such as brain development and disease. Graph mining on brain networks may facilitate the discovery of novel biomarkers for clinical phenotypes and neurodegenerative diseases. Since brain networks derived from functional and structural MRI describe the brain topology from different perspectives, exploring a representation that combines these cross-modality brain networks is non-trivial. Most current studies aim to extract a fused representation of the two types of brain network by projecting the structural network to the functional counterpart. Since the functional network is dynamic and the structural network is static, mapping a static object to a dynamic object is suboptimal. However, mapping in the opposite direction is not feasible due to the non-negativity requirement of current graph learning techniques. Here, we propose a novel graph learning framework, known as Deep Signed Brain Networks (DSBN), with a signed graph encoder that, from an opposite perspective, learns the cross-modality representations by projecting the functional network to the structural counterpart. We validate our framework on clinical phenotype and neurodegenerative disease prediction tasks using two independent, publicly available datasets (HCP and OASIS). The experimental results clearly demonstrate the advantages of our model compared to several state-of-the-art methods.
更多查看译文
关键词
brain,networks,representation
AI 理解论文
溯源树
样例
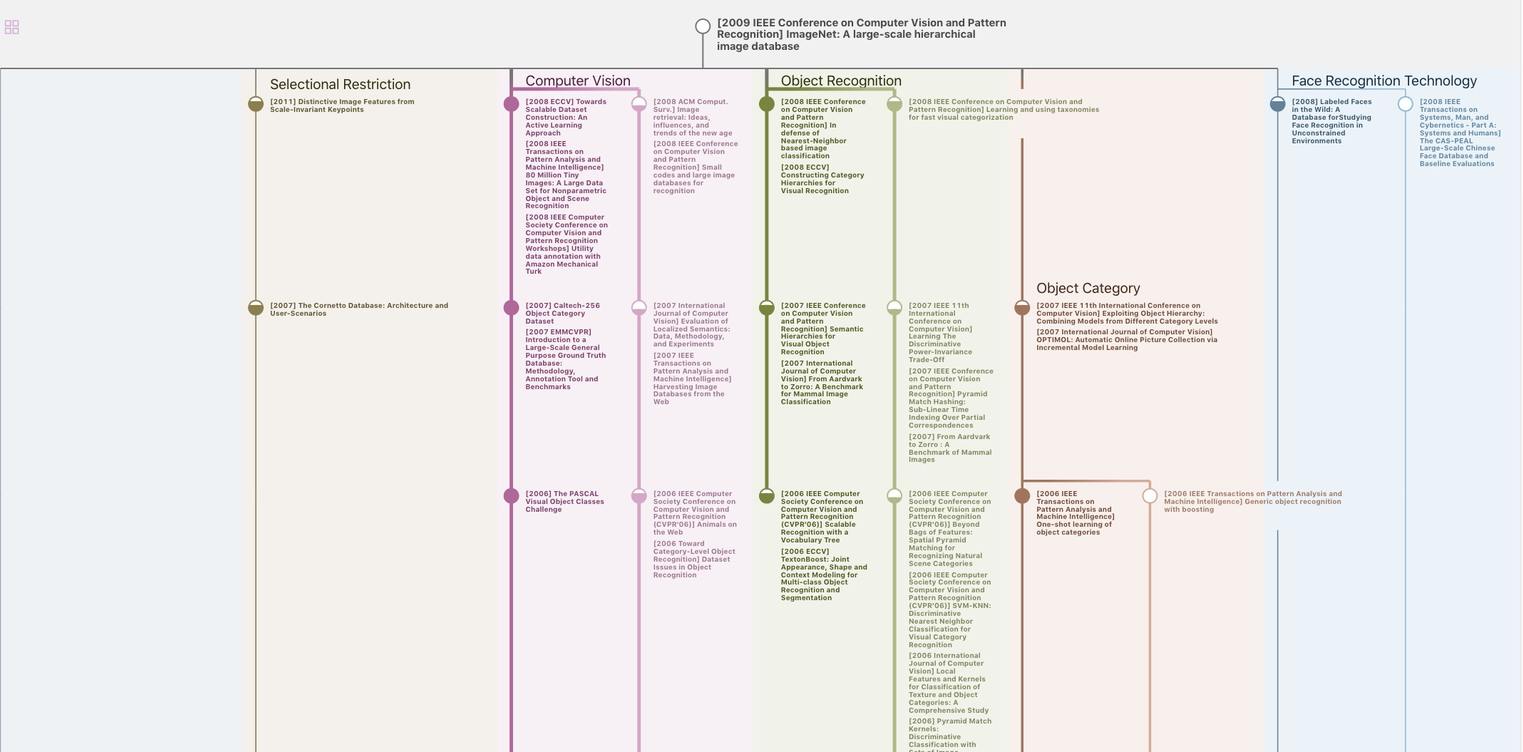
生成溯源树,研究论文发展脉络
Chat Paper
正在生成论文摘要