Massive MIMO for Serving Federated Learning and Non-Federated Learning Users
IEEE TRANSACTIONS ON WIRELESS COMMUNICATIONS(2024)
摘要
With its privacy preservation and communication efficiency, federated learning (FL) has emerged as a promising learning framework for beyond 5G wireless networks. It is anticipated that future wireless networks will jointly serve both FL and downlink non-FL user groups in the same time-frequency resource. While in the downlink of each FL iteration, both groups simultaneously receive data from the base station in the same time-frequency resource, the uplink of each FL iteration requires bidirectional communication to support uplink transmission for FL users and downlink transmission for non-FL users. To overcome this challenge, we present half-duplex (HD) and full-duplex (FD) communication schemes to serve both groups. More specifically, we adopt the massive multiple-input multiple-output technology and aim to maximize the minimum effective rate of non-FL users under a quality of service (QoS) latency constraint for FL users. Since the formulated problem is nonconvex, we propose a power control algorithm based on successive convex approximation to find a stationary solution. Numerical results show that the proposed solutions perform significantly better than the considered baselines schemes. Moreover, the FD-based scheme outperforms the HD-based counterpart in scenarios where the self-interference is small or moderate and/or the size of FL model updates is large.
更多查看译文
关键词
Massive multiple-input multiple-output (MIMO),federated learning (FL),resource allocation,successive convex approximation (SCA)
AI 理解论文
溯源树
样例
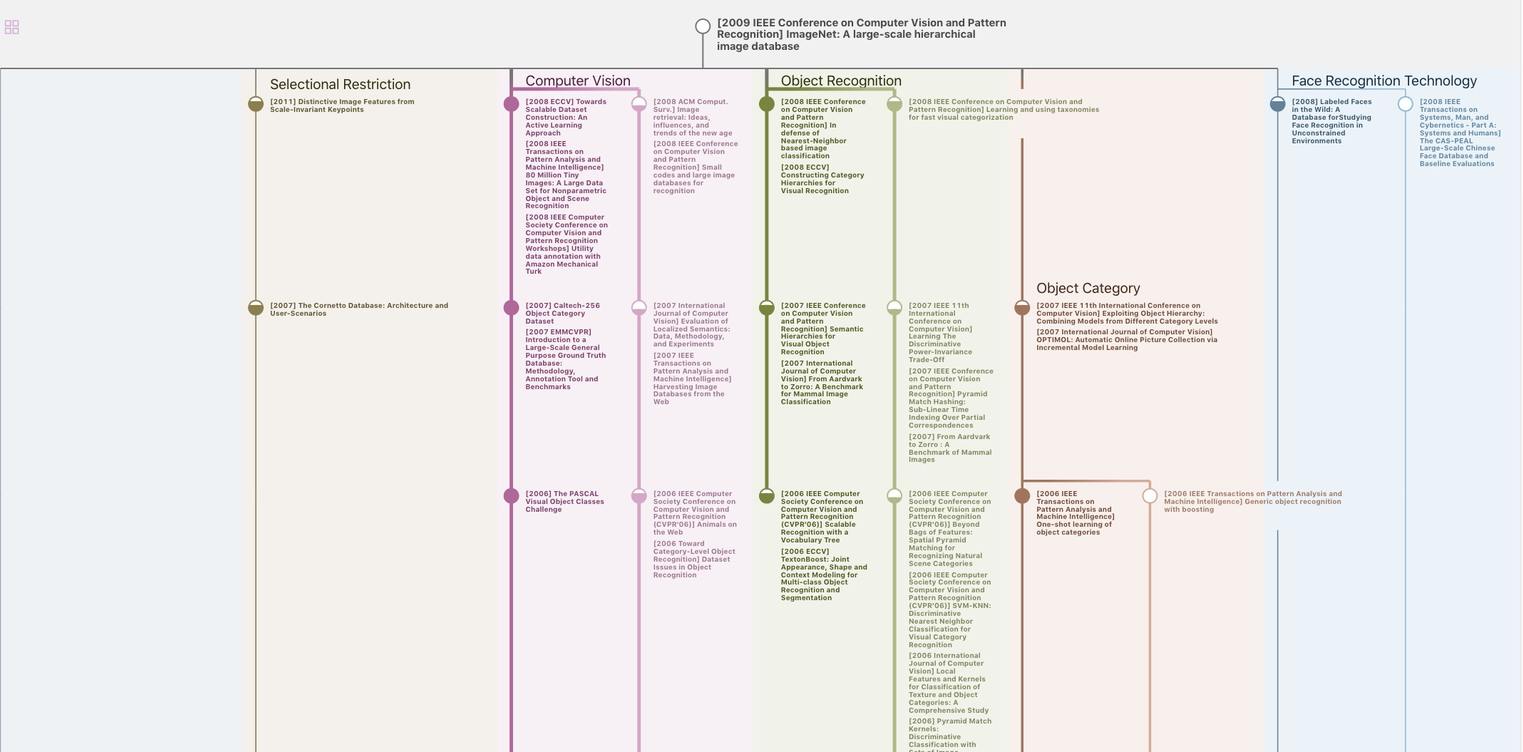
生成溯源树,研究论文发展脉络
Chat Paper
正在生成论文摘要