New Lower Bounds for Private Estimation and\\a Generalized Fingerprinting Lemma
NeurIPS 2022(2022)
摘要
We prove new lower bounds for statistical estimation tasks under the constraint of $\paren{\eps, \delta}$-differential privacy. First, we provide tight lower bounds for private covariance estimation of Gaussian distributions. We show that estimating the covariance matrix in Frobenius norm requires $\Omega\paren{d^2}$ samples, and in spectral norm requires $\Omega\paren{d^{\frac{3}{2}}}$ samples, both matching upper bounds up to logarithmic factors. We prove these bounds via our main technical contribution, a broad generalization of the fingerprinting method~\cite{BunUV14} to exponential families. Additionally, using the private Assouad method of Acharya, Sun, and Zhang~\cite{AcharyaSZ21}, we show a tight $\Omega\paren{\frac{d}{\alpha^2 \eps}}$ lower bound for estimating the mean of a distribution with bounded covariance to $\alpha$-error in $\ell_2$-distance. Prior known lower bounds for all these problems were either polynomially weaker or held under the stricter condition of $\paren{\eps,0}$-differential privacy.
更多查看译文
关键词
Differential Privacy,Learning,Machine Learning,Data Privacy,Statistics,Gaussians,Covariance Estimation,Lower Bounds,Mean Estimation
AI 理解论文
溯源树
样例
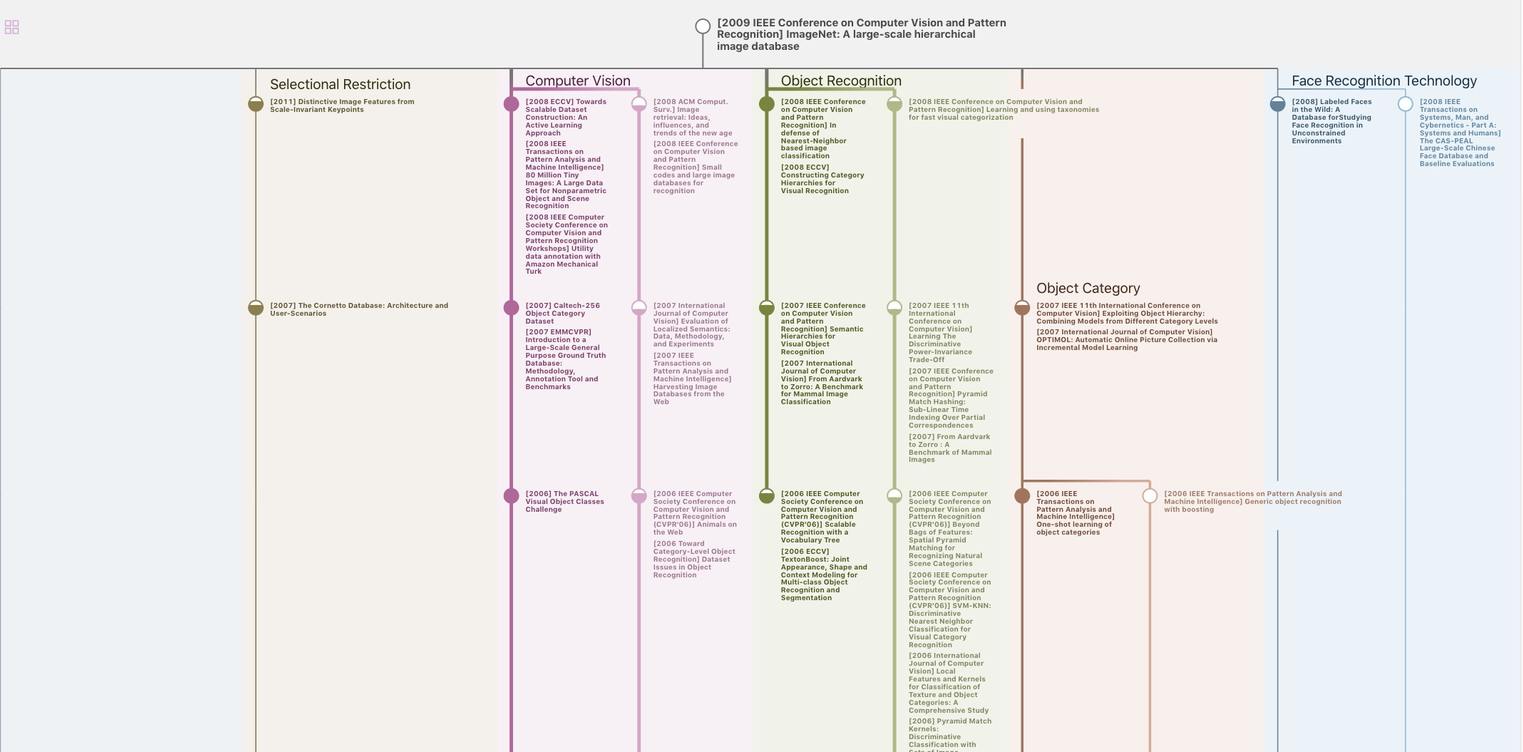
生成溯源树,研究论文发展脉络
Chat Paper
正在生成论文摘要