Anomaly Guided Policy Learning from Imperfect Demonstrations.
International Joint Conference on Autonomous Agents and Multi-agent Systems(2022)
摘要
Learning from Demonstrations (LfD) refers to using expert demonstrations combined with the reward information given by the environment to jointly guide the learning of policy in Reinforcement Learning. Previous LfD methods usually assume that provided demonstrations are perfect., while in real-world applications, demonstrations are often collected from multiple sources, which may contain imperfect ones. In this work, we aim to deal with the latter situation, i.e., Learning from Imperfect Demonstrations (LfID), where demonstrations only include trajectories with state-action pairs. To this end, two challenges need to be solved: evaluation for the demonstrations and calibration for the bonus model. Both challenges can be more severe in sparse reward environments, since the exploration problem will appear while learning. In this work, we focus on bridging the exploration and LfID problems in view of anomaly detection, and further proposing AGPO method to deal with these problems. Compared with state-of-the-art methods, empirical studies on some challenging continuous control benchmarks show the superiority of AGPO in this scenario.
更多查看译文
AI 理解论文
溯源树
样例
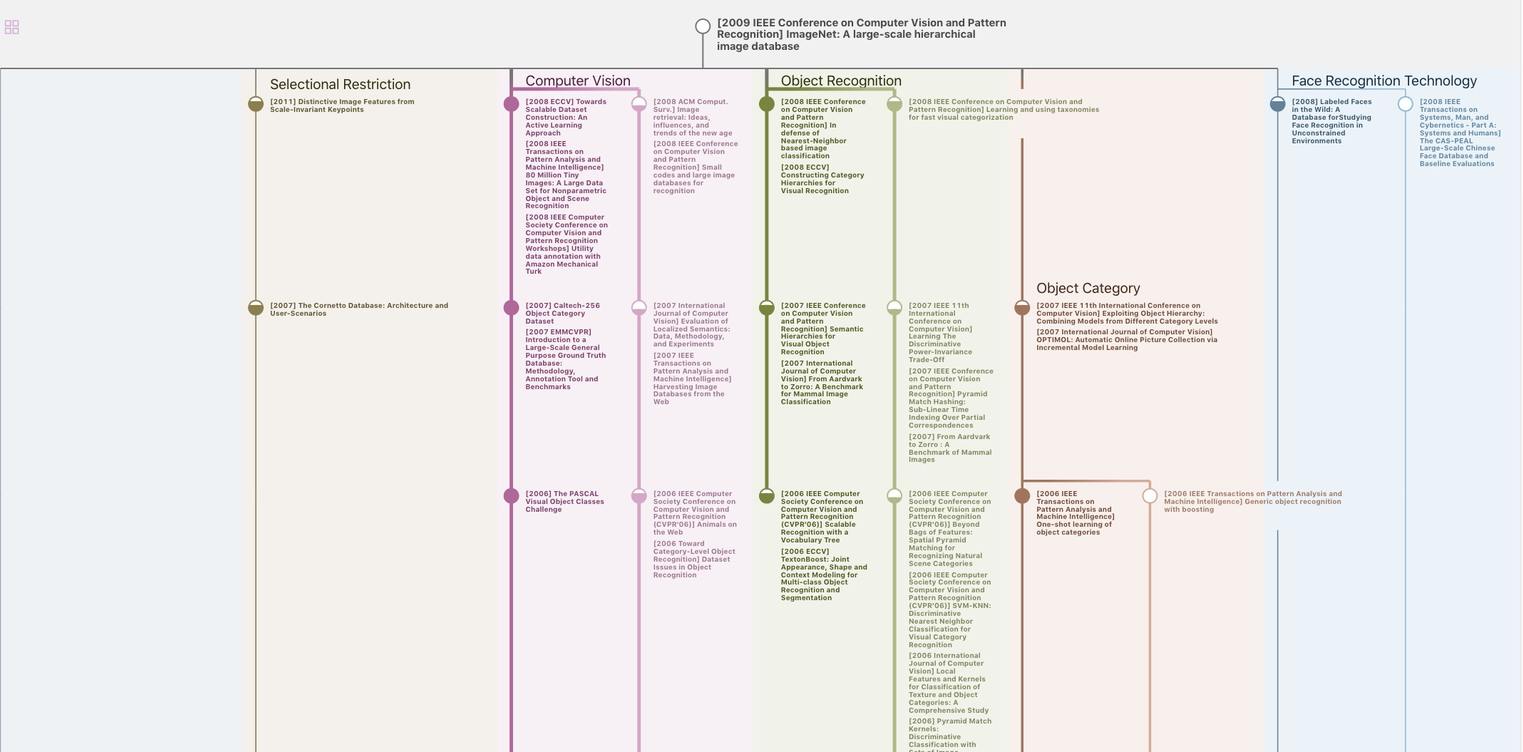
生成溯源树,研究论文发展脉络
Chat Paper
正在生成论文摘要