Improving Generalization with Cross-State Behavior Matching in Deep Reinforcement Learning.
International Joint Conference on Autonomous Agents and Multi-agent Systems(2022)
摘要
Representation learning on visualized input is an essential yet challenging task for deep reinforcement learning (RL). To help the RL agent learn more general and discriminative representation among various states, we present cross-state self-constraint (CSSC). This novel technique regularizes representation learning by comparing state embedding similarities across different state-action pairs. We test our proposed method on the OpenAI Procgen benchmark with Rainbow and PPO and demonstrate significant improvement across most Procgen environments.
更多查看译文
AI 理解论文
溯源树
样例
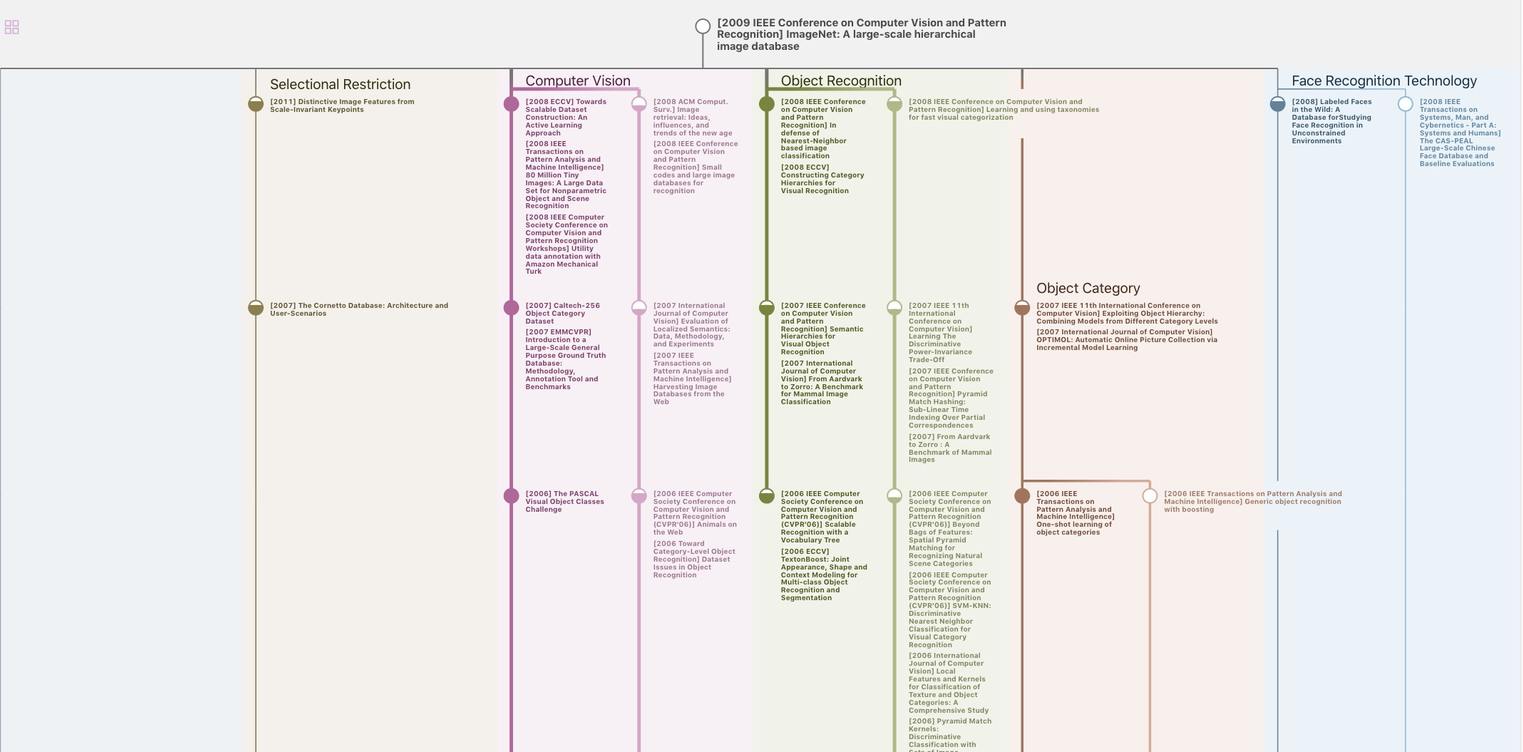
生成溯源树,研究论文发展脉络
Chat Paper
正在生成论文摘要