SBSim: A Sentence-BERT Similarity-Based Evaluation Metric for Indian Language Neural Machine Translation Systems
IEEE-ACM TRANSACTIONS ON AUDIO SPEECH AND LANGUAGE PROCESSING(2022)
摘要
Machine translation (MT) outputs are widely scored using automatic evaluation metrics and human evaluation scores. The automatic evaluation metrics are expected to be easily computable and a reflection of human evaluation. Traditional string-based metrics such as BLEU, ChrF++ scores, are widely used to evaluate MT systems, but fail to account for synonyms that appear in the state-of-the-art neural machine translation (NMT) systems, owing to their inability to evaluate paraphrases. While similarity-based metrics such as Yisi, BERTScore address this issue, these metrics need to be modified to better evaluate morphologically rich Indian languages such as, Tamil and Hindi. The current work proposes a novel and individual sentence-BERT based similarity (SBSim) metric, that makes use of a paraphrase-BERT model and sentence-level embedding to evaluate NMT outputs. The effectiveness of the BLEU, ChrF++, Yisi, BERTScore, and the proposed SBSim are evaluated on English-to-Tamil and English-to-Hindi NMT outputs. The sentence-level metric correlation of the proposed SBSim metric with respect to human scores is observed to outperform the existing metrics with a correlation of 0.9123 and 0.9052 for English-to-Tamil and English-to-Hindi NMT systems, respectively. Further, the average metric correlation of the SBSim metric is also observed to be the highest with a value of 0.9801 and 0.9836 for these NMT systems, respectively. The proposed metric is also evaluated on WMT2020 dataset and reports the highest correlation of 0.7129 with the human scores.
更多查看译文
关键词
Measurement, Speech processing, Correlation, Computational modeling, Surface morphology, Machine translation, Linguistics, Automatic evaluation, human correlation, individual metric, paraphrase-BERT, sentence-BERT
AI 理解论文
溯源树
样例
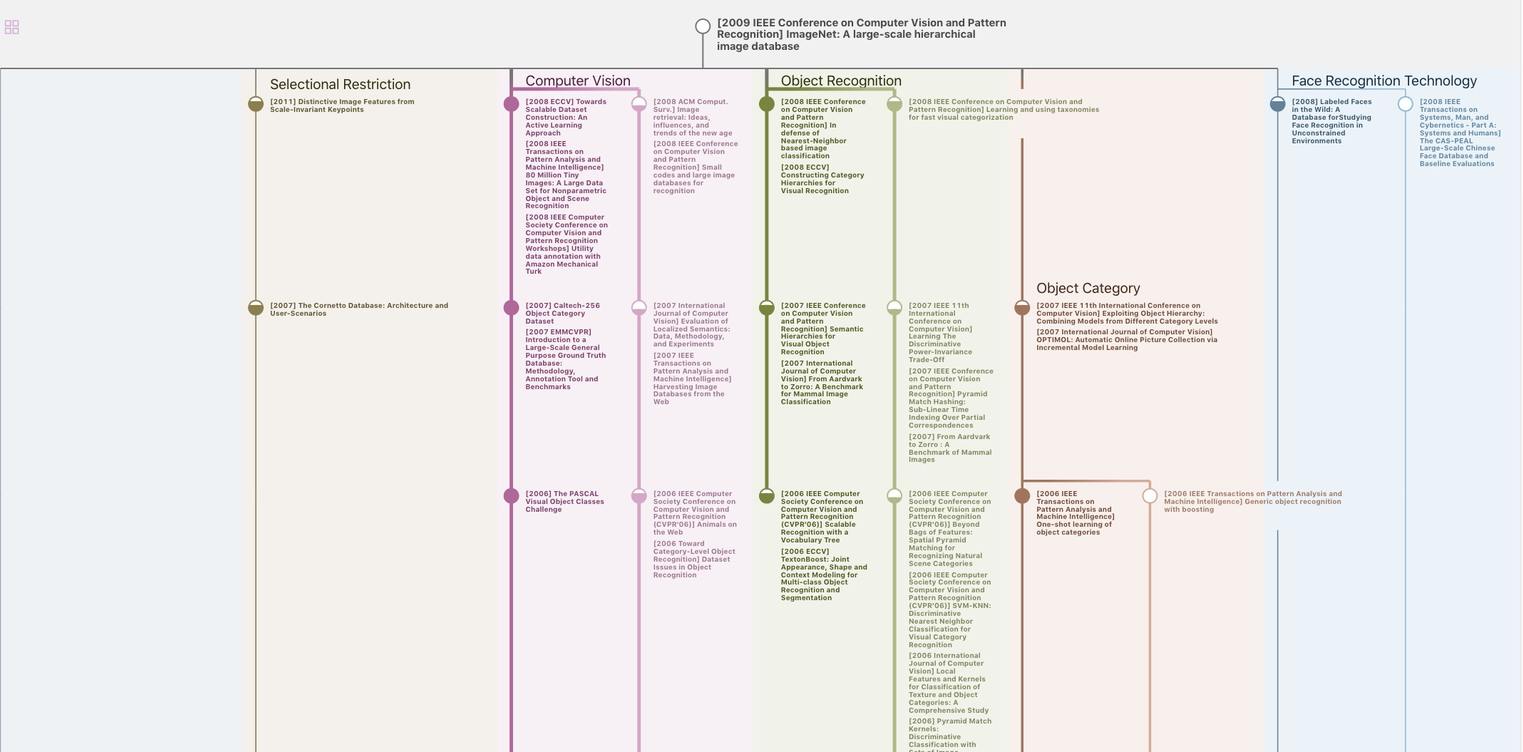
生成溯源树,研究论文发展脉络
Chat Paper
正在生成论文摘要