Automating In-Network Machine Learning
arxiv(2022)
摘要
Using programmable network devices to aid in-network machine learning has been the focus of significant research. However, most of the research was of a limited scope, providing a proof of concept or describing a closed-source algorithm. To date, no general solution has been provided for mapping machine learning algorithms to programmable network devices. In this paper, we present Planter, an open-source, modular framework for mapping trained machine learning models to programmable devices. Planter supports a wide range of machine learning models, multiple targets and can be easily extended. The evaluation of Planter compares different mapping approaches, and demonstrates the feasibility, performance, and resource efficiency for applications such as anomaly detection, financial transactions, and quality of experience. The results show that Planter-based in-network machine learning algorithms can run at line rate, have a negligible effect on latency, coexist with standard switching functionality, and have no or minor accuracy trade-offs.
更多查看译文
关键词
machine learning,in-network
AI 理解论文
溯源树
样例
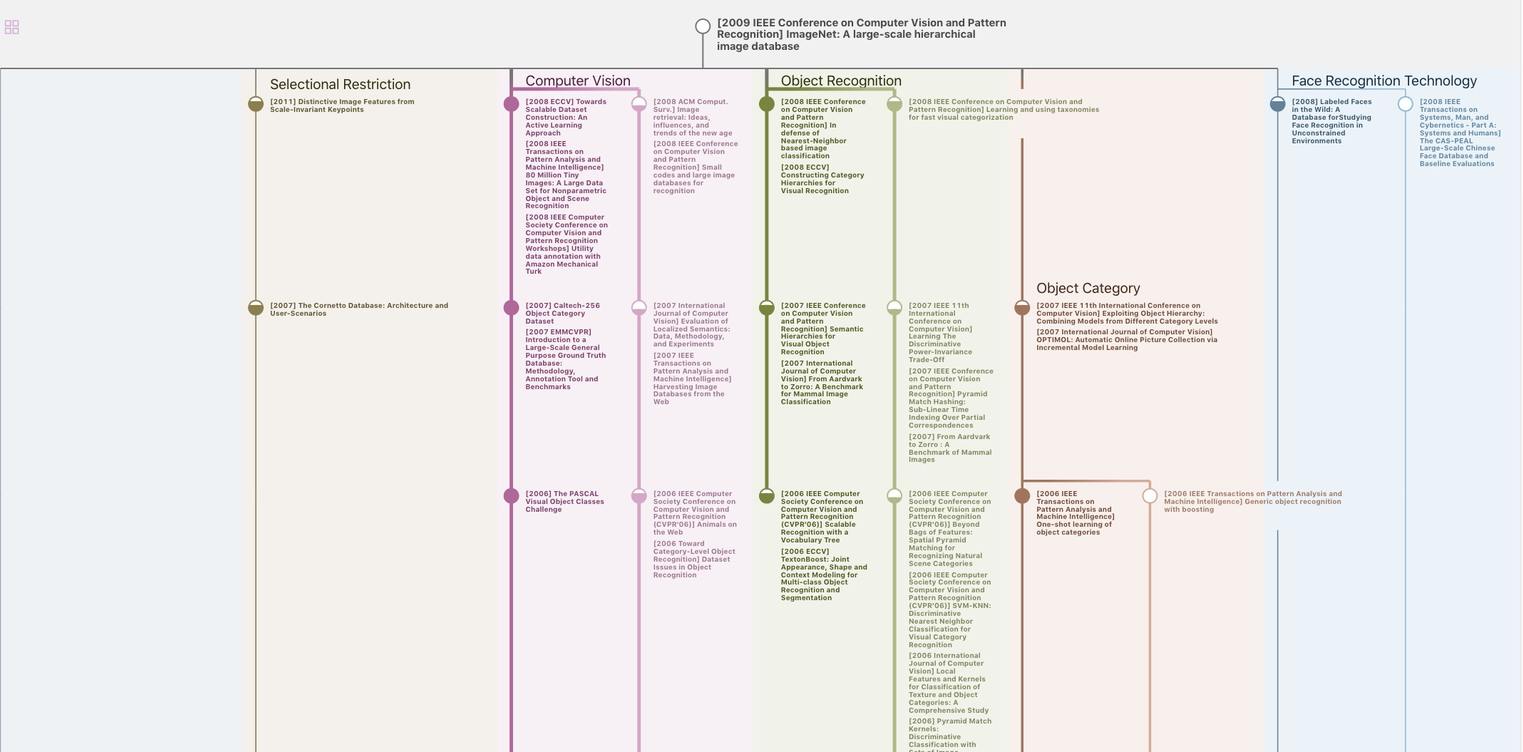
生成溯源树,研究论文发展脉络
Chat Paper
正在生成论文摘要