Empirical Advocacy of Bio-inspired Models for Robust Image Recognition
arxiv(2022)
摘要
Deep convolutional neural networks (DCNNs) have revolutionized computer vision and are often advocated as good models of the human visual system. However, there are currently many shortcomings of DCNNs, which preclude them as a model of human vision. There are continuous attempts to use features of the human visual system to improve the robustness of neural networks to data perturbations. We provide a detailed analysis of such bio-inspired models and their properties. To this end, we benchmark the robustness of several bio-inspired models against their most comparable baseline DCNN models. We find that bio-inspired models tend to be adversarially robust without requiring any special data augmentation. Additionally, we find that bio-inspired models beat adversarially trained models in the presence of more real-world common corruptions. Interestingly, we also find that bio-inspired models tend to use both low and mid-frequency information, in contrast to other DCNN models. We find that this mix of frequency information makes them robust to both adversarial perturbations and common corruptions.
更多查看译文
关键词
recognition,models,image,bio-inspired
AI 理解论文
溯源树
样例
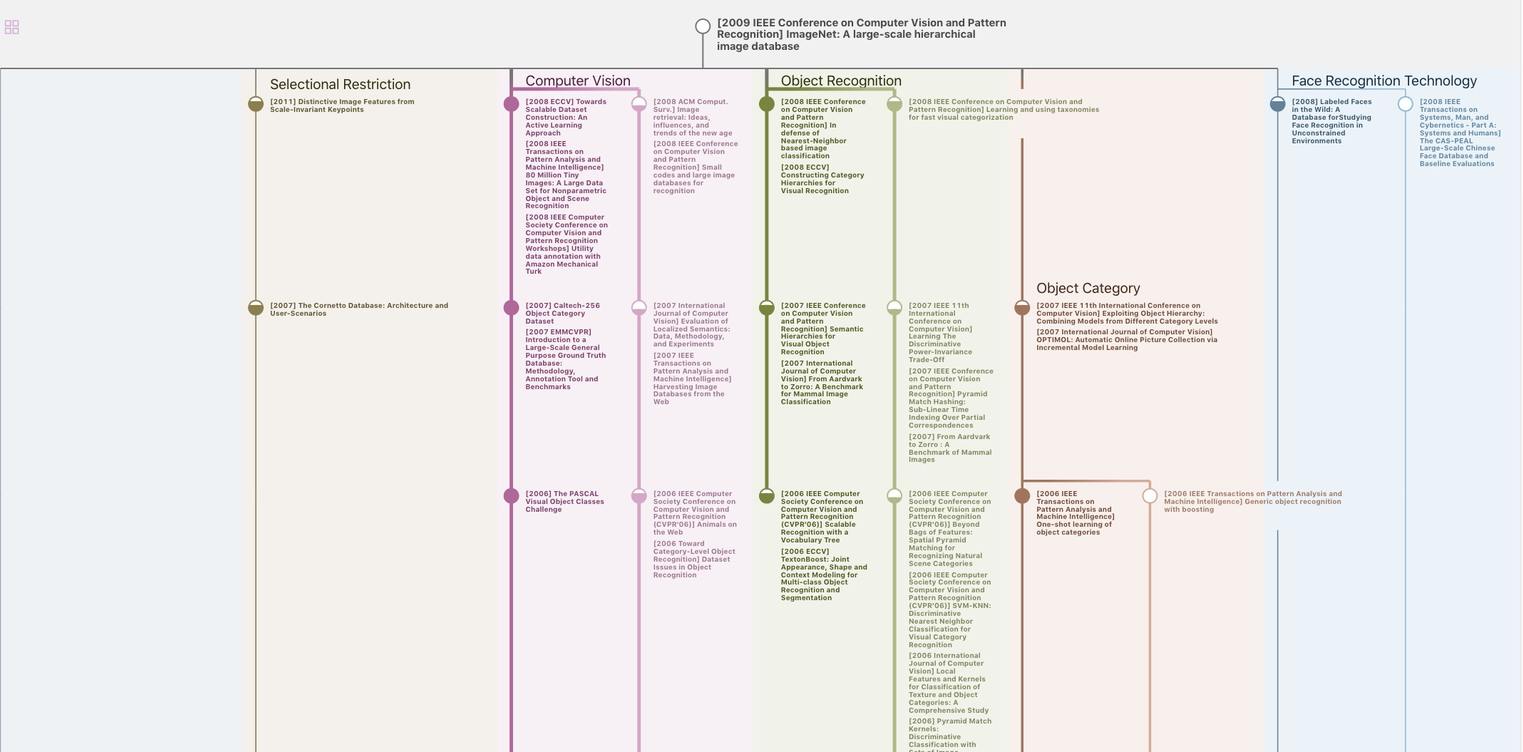
生成溯源树,研究论文发展脉络
Chat Paper
正在生成论文摘要