Correct Me If I'm Wrong: Using Non-Experts to Repair Reinforcement Learning Policies
ACM/IEEE International Conference on Human-Robot Interaction(2022)
摘要
ABSTRACTReinforcement learning has shown great potential for learning sequential decision-making tasks. Yet, it is difficult to anticipate all possible real-world scenarios during training, causing robots to inevitably fail in the long run. Many of these failures are due to variations in the robot's environment. Usually experts are called to correct the robot's behavior; however, some of these failures do not necessarily require an expert to solve them. In this work, we query non-experts online for help and explore 1) if/how non-experts can provide feedback to the robot after a failure and 2) how the robot can use this feedback to avoid such failures in the future by generating shields that restrict or correct its high-level actions. We demonstrate our approach on common daily scenarios of a simulated kitchen robot. The results indicate that non-experts can indeed understand and repair robot failures. Our generated shields accelerate learning and improve data-efficiency during retraining.
更多查看译文
关键词
robot failure,policy repair,non-experts,shielded reinforcement learning
AI 理解论文
溯源树
样例
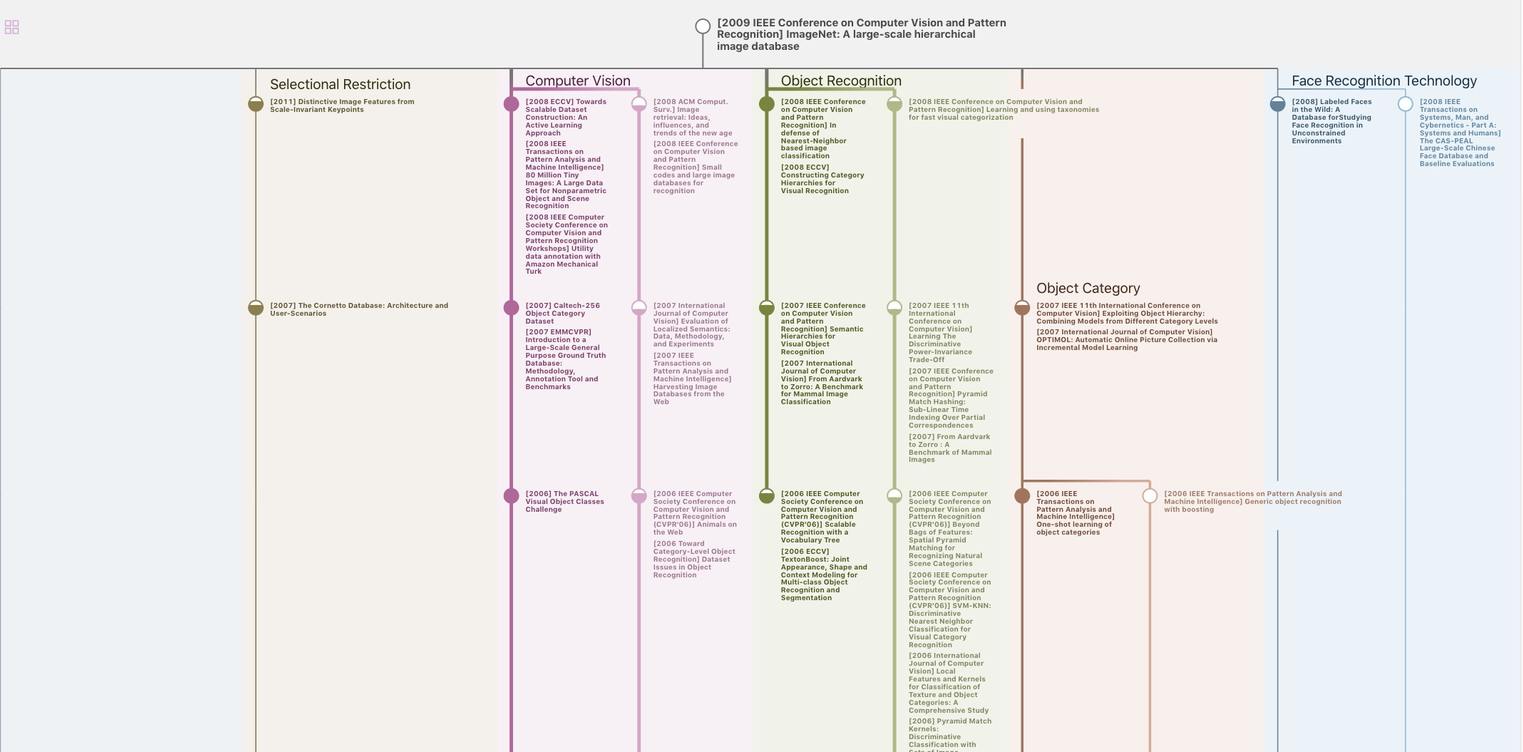
生成溯源树,研究论文发展脉络
Chat Paper
正在生成论文摘要