Hybrid anomaly detection and prioritization for network logs at cloud scale
European Conference on Computer Systems(2022)
摘要
ABSTRACTMonitoring the health of large-scale systems requires significant manual effort, usually through the continuous curation of alerting rules based on keywords, thresholds and regular expressions, which might generate a flood of mostly irrelevant alerts and obscure the actual information operators would like to see. Existing approaches try to improve the observability of systems by intelligently detecting anomalous situations. Such solutions surface anomalies that are statistically significant, but may not represent events that reliability engineers consider relevant. We propose ADEPTUS, a practical approach for detection of relevant health issues in an established system. ADEPTUS combines statistics and unsupervised learning to detect anomalies with supervised learning and heuristics to determine which of the detected anomalies are likely to be relevant to the Site Reliability Engineers (SREs). ADEPTUS overcomes the labor-intensive prerequisite of obtaining anomaly labels for supervised learning by automatically extracting information from historic alerts and incident tickets. We leverage ADEPTUS for observability in the network infrastructure of IBM Cloud. We perform an extensive real-world evaluation on 10 months of logs generated by tens of thousands of network devices across 11 data centers and demonstrate that ADEPTUS achieves higher alerting accuracy than the rule-based log alerting solution, curated by domain experts, used by SREs daily.
更多查看译文
关键词
Anomaly Detection, Log Analysis, Reliability, Machine Learning, Deep Learning, Cloud Computing, AIOps
AI 理解论文
溯源树
样例
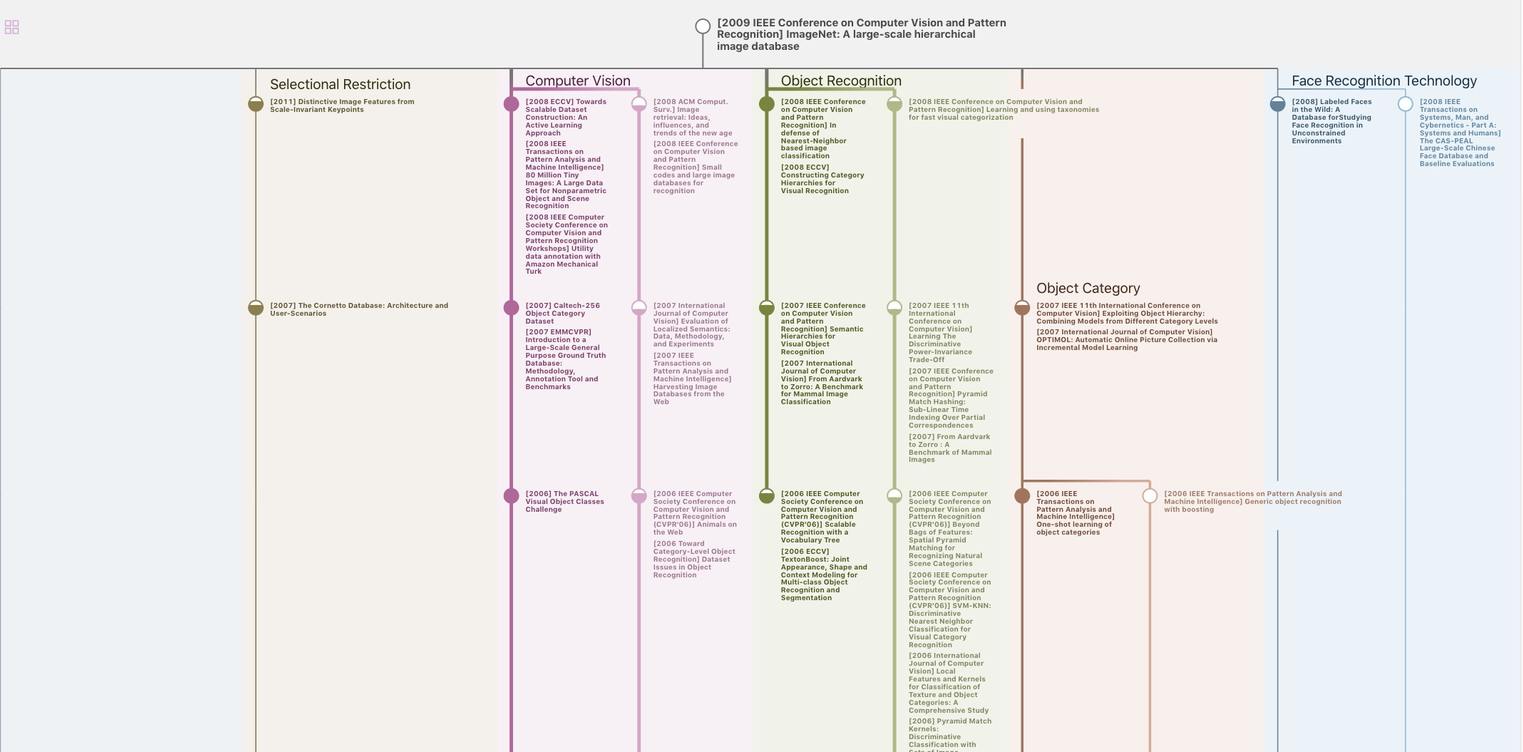
生成溯源树,研究论文发展脉络
Chat Paper
正在生成论文摘要