FedILC: Weighted Geometric Mean and Invariant Gradient Covariance for Federated Learning on Non-IID Data
CoRR(2022)
摘要
Federated learning is a distributed machine learning approach which enables a shared server model to learn by aggregating the locally-computed parameter updates with the training data from spatially-distributed client silos. Though successfully possessing advantages in both scale and privacy, federated learning is hurt by domain shift problems, where the learning models are unable to generalize to unseen domains whose data distribution is non-i.i.d. with respect to the training domains. In this study, we propose the Federated Invariant Learning Consistency (FedILC) approach, which leverages the gradient covariance and the geometric mean of Hessians to capture both inter-silo and intra-silo consistencies of environments and unravel the domain shift problems in federated networks. The benchmark and real-world dataset experiments bring evidence that our proposed algorithm outperforms conventional baselines and similar federated learning algorithms. This is relevant to various fields such as medical healthcare, computer vision, and the Internet of Things (IoT). The code is released at https://github.com/mikemikezhu/FedILC.
更多查看译文
关键词
federated learning,invariant gradient covariance,weighted geometric mean,non-iid
AI 理解论文
溯源树
样例
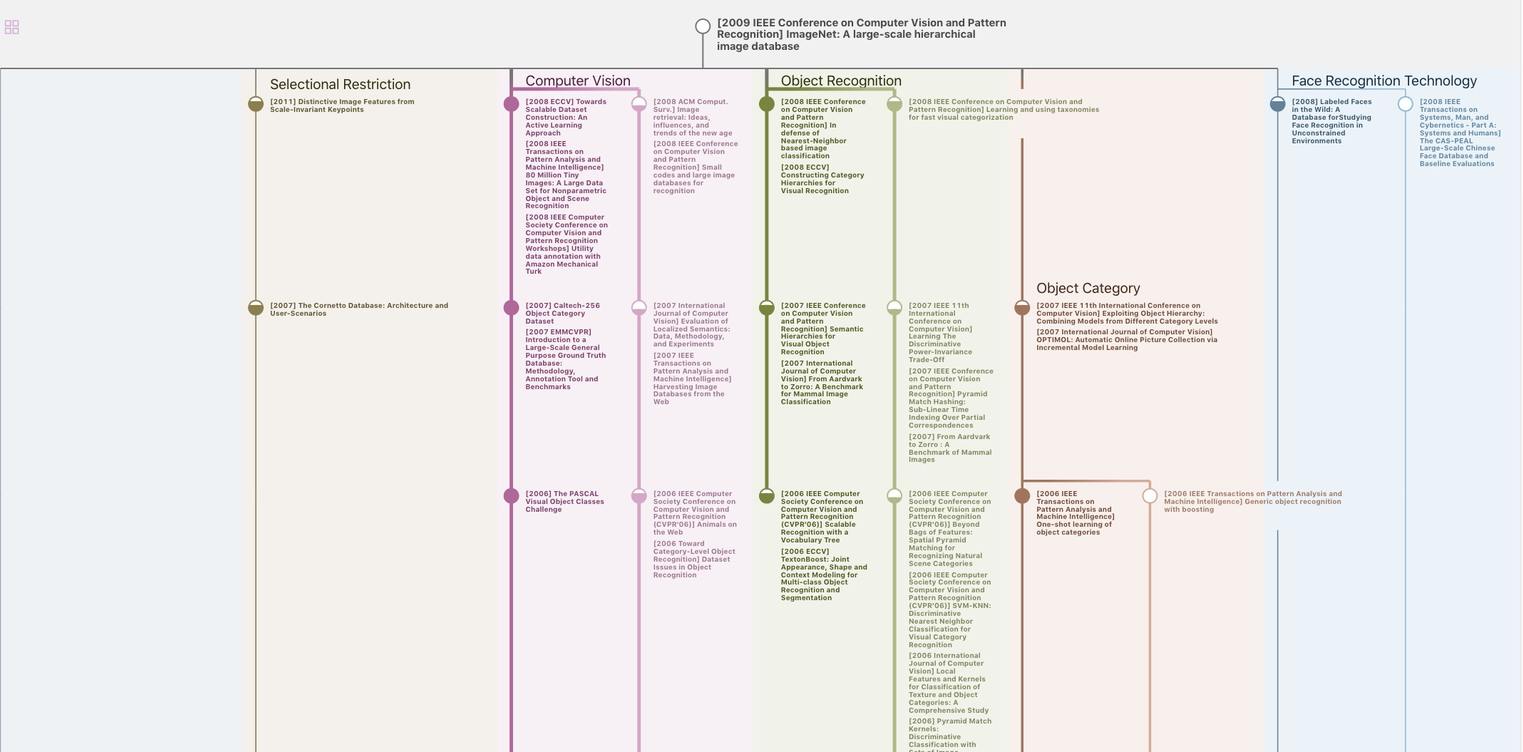
生成溯源树,研究论文发展脉络
Chat Paper
正在生成论文摘要