Differentially private Riemannian optimization
Machine Learning(2024)
摘要
In this paper, we study the differentially private empirical risk minimization problem where the parameter is constrained to a Riemannian manifold. We introduce a framework for performing differentially private Riemannian optimization by adding noise to the Riemannian gradient on the tangent space. The noise follows a Gaussian distribution intrinsically defined with respect to the Riemannian metric on the tangent space. We adapt the Gaussian mechanism from the Euclidean space to the tangent space compatible to such generalized Gaussian distribution. This approach presents a novel analysis as compared to directly adding noise on the manifold. We further prove privacy guarantees of the proposed differentially private Riemannian (stochastic) gradient descent using an extension of the moments accountant technique. Overall, we provide utility guarantees under geodesic (strongly) convex, general nonconvex objectives as well as under the Riemannian Polyak-Łojasiewicz condition. Empirical results illustrate the versatility and efficacy of the proposed framework in several applications.
更多查看译文
关键词
Riemannian manifold,Riemannian optimization,Stochastic optimization,Differential privacy,Empirical risk minimization,Tangent Gaussian
AI 理解论文
溯源树
样例
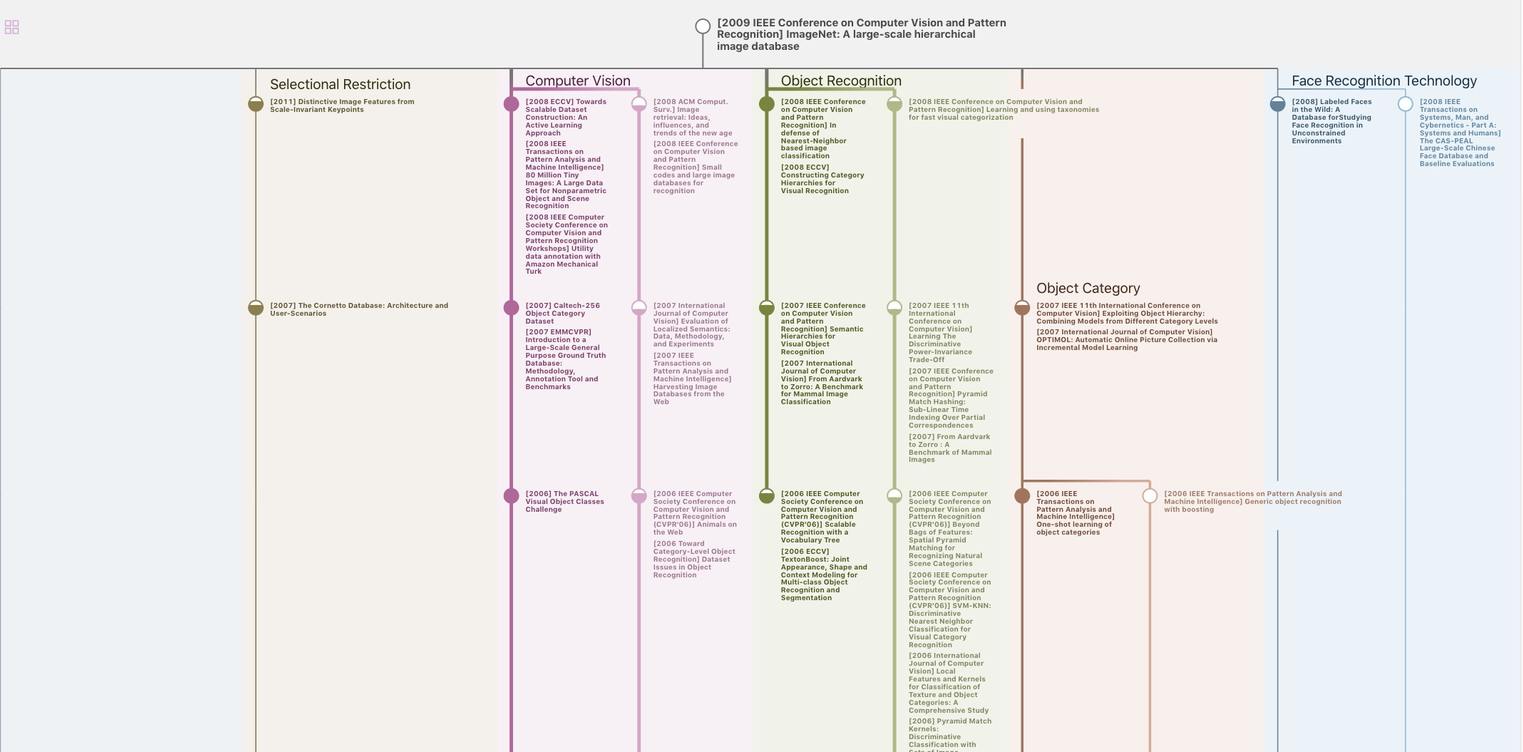
生成溯源树,研究论文发展脉络
Chat Paper
正在生成论文摘要