How catastrophic can catastrophic forgetting be in linear regression?
Annual Conference on Computational Learning Theory(2022)
摘要
To better understand catastrophic forgetting, we study fitting an overparameterized linear model to a sequence of tasks with different input distributions. We analyze how much the model forgets the true labels of earlier tasks after training on subsequent tasks, obtaining exact expressions and bounds. We establish connections between continual learning in the linear setting and two other research areas – alternating projections and the Kaczmarz method. In specific settings, we highlight differences between forgetting and convergence to the offline solution as studied in those areas. In particular, when $T$ tasks in $d$ dimensions are presented cyclically for $k$ iterations, we prove an upper bound of $T^2\min\{1/\sqrt{k},d/k\}$ on the forgetting. This stands in contrast to the convergence to the offline solution, which can be arbitrarily slow according to existing alternating projection results. We further show that the $T^2$ factor can be lifted when tasks are presented in a random ordering.
更多查看译文
关键词
catastrophic forgetting,linear regression
AI 理解论文
溯源树
样例
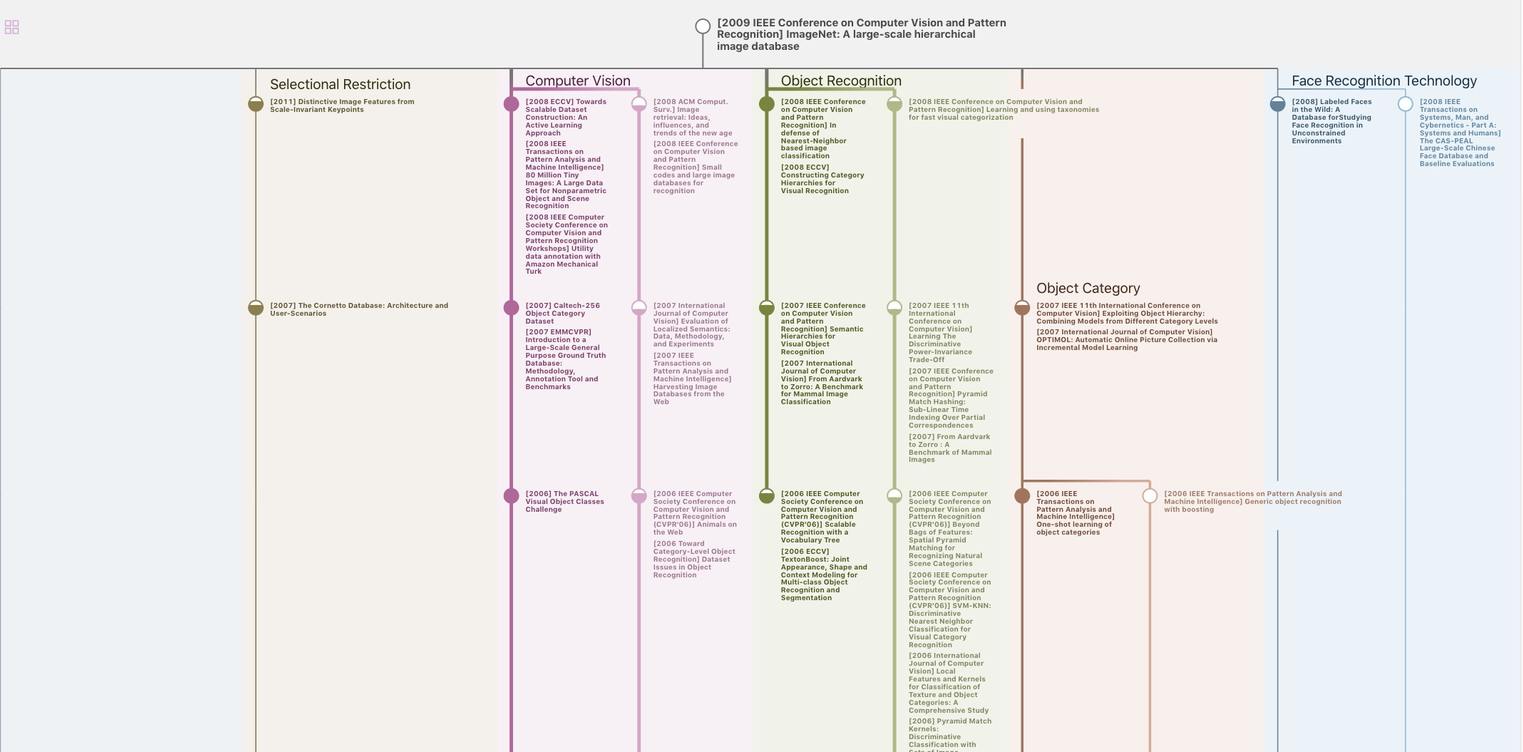
生成溯源树,研究论文发展脉络
Chat Paper
正在生成论文摘要