Multi-Armed Bandits in Brain-Computer Interfaces
FRONTIERS IN HUMAN NEUROSCIENCE(2022)
摘要
The multi-armed bandit (MAB) problem models a decision-maker that optimizes its actions based on current and acquired new knowledge to maximize its reward. This type of online decision is prominent in many procedures of Brain-Computer Interfaces (BCIs) and MAB has previously been used to investigate, e.g., what mental commands to use to optimize BCI performance. However, MAB optimization in the context of BCI is still relatively unexplored, even though it has the potential to improve BCI performance during both calibration and real-time implementation. Therefore, this review aims to further describe the fruitful area of MABs to the BCI community. The review includes a background on MAB problems and standard solution methods, and interpretations related to BCI systems. Moreover, it includes state-of-the-art concepts of MAB in BCI and suggestions for future research.
更多查看译文
关键词
multi-armed bandit (MAB), Brain-Computer Interface (BCI), reinforcement learning, calibration, real-time optimization
AI 理解论文
溯源树
样例
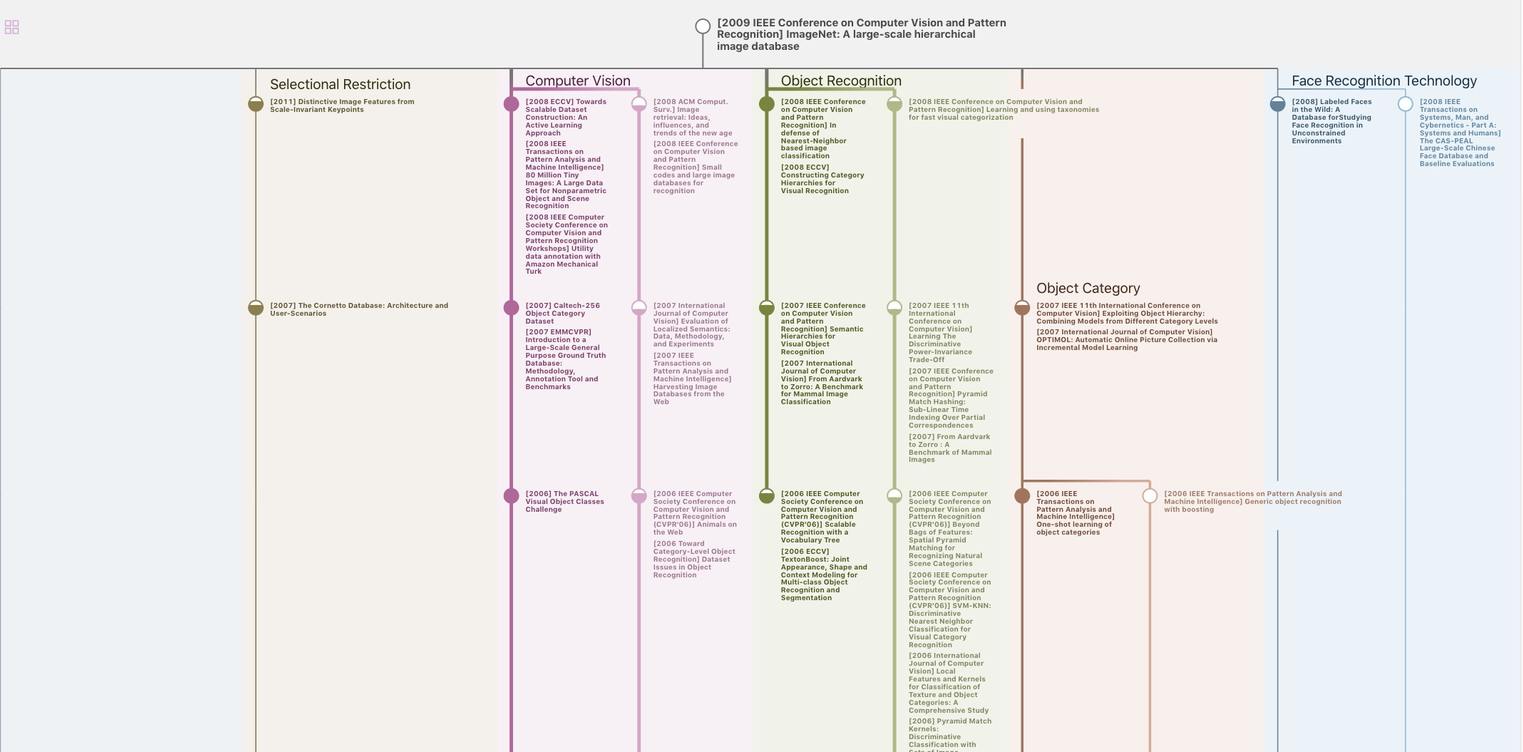
生成溯源树,研究论文发展脉络
Chat Paper
正在生成论文摘要