JointCL: A Joint Contrastive Learning Framework for Zero-Shot Stance Detection
PROCEEDINGS OF THE 60TH ANNUAL MEETING OF THE ASSOCIATION FOR COMPUTATIONAL LINGUISTICS (ACL 2022), VOL 1: (LONG PAPERS)(2022)
摘要
Zero-shot stance detection (ZSSD) aims to detect the stance for an unseen target during the inference stage. In this paper, we propose a joint contrastive learning (JointCL) framework, which consists of stance contrastive learning and target-aware prototypical graph contrastive learning. Specifically, a stance contrastive learning strategy is employed to better generalize stance features for unseen targets. Further, we build a prototypical graph for each instance to learn the target-based representation, in which the prototypes are deployed as a bridge to share the graph structures between the known targets and the unseen ones. Then a novel target-aware prototypical graph contrastive learning strategy is devised to generalize the reasoning ability of target-based stance representations to the unseen targets. Extensive experiments on three benchmark datasets show that the proposed approach achieves state-of-the-art performance in the ZSSD task(1).
更多查看译文
关键词
stance,jointcl contrastive learning framework,detection,zero-shot
AI 理解论文
溯源树
样例
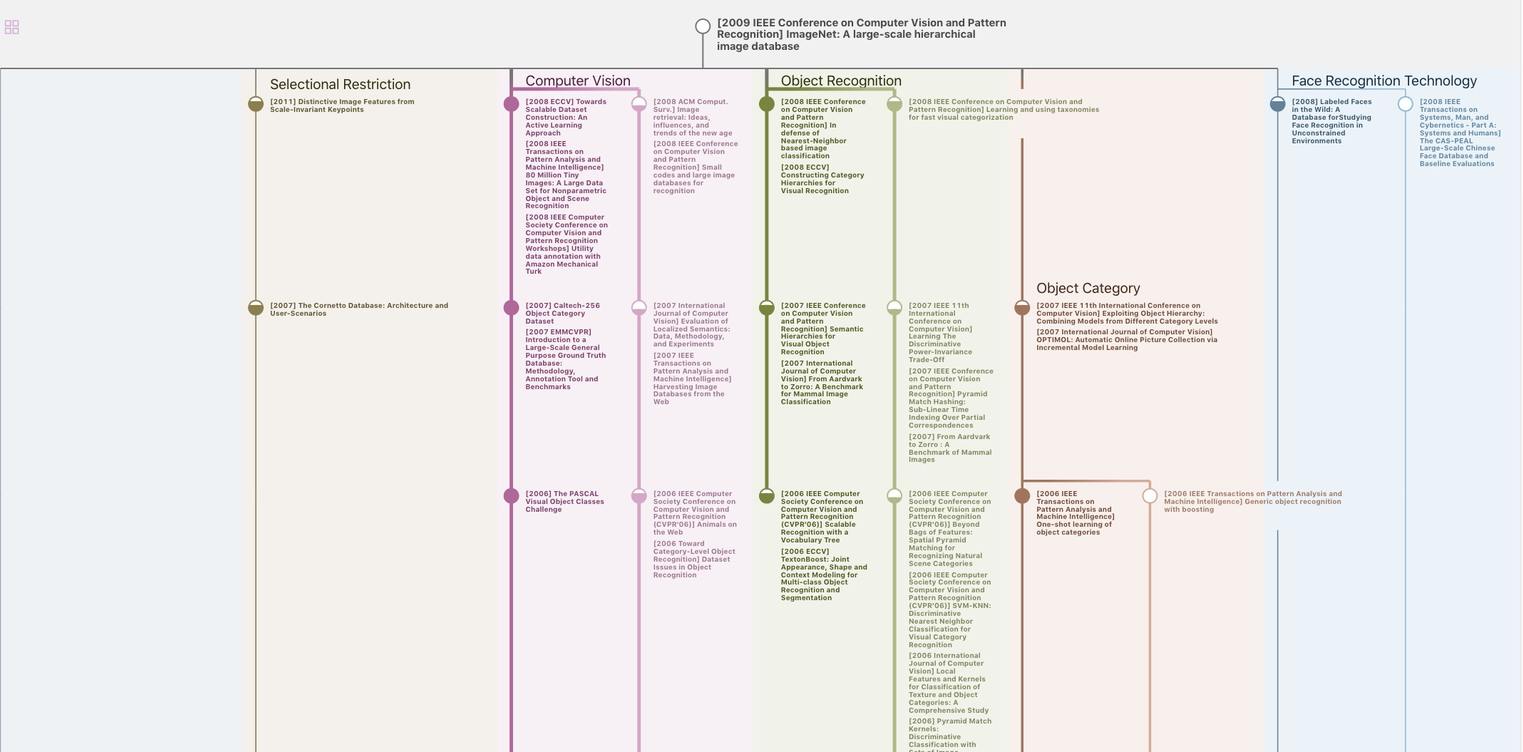
生成溯源树,研究论文发展脉络
Chat Paper
正在生成论文摘要