Video Language Co-Attention with Multimodal Fast-Learning Feature Fusion for VideoQA
PROCEEDINGS OF THE 7TH WORKSHOP ON REPRESENTATION LEARNING FOR NLP(2022)
摘要
We propose the Video Language Co-Attention Network (VLCN) - a novel memory-enhanced model for Video Question Answering (VideoQA). Our model combines two original contributions: A multimodal fast-learning feature fusion (FLF) block and a mechanism that uses self-attended language features to separately guide neural attention on both static and dynamic visual features extracted from individual video frames and short video clips. When trained from scratch, VLCN achieves competitive results with the state of the art on both MSVD-QA and MSRVTT-QA with 38.06% and 36.01% test accuracies, respectively. Through an ablation study, we further show that FLF improves generalization across different VideoQA datasets and performance for question types that are notoriously challenging in current datasets, such as long questions that require deeper reasoning as well as questions with rare answers(1).
更多查看译文
AI 理解论文
溯源树
样例
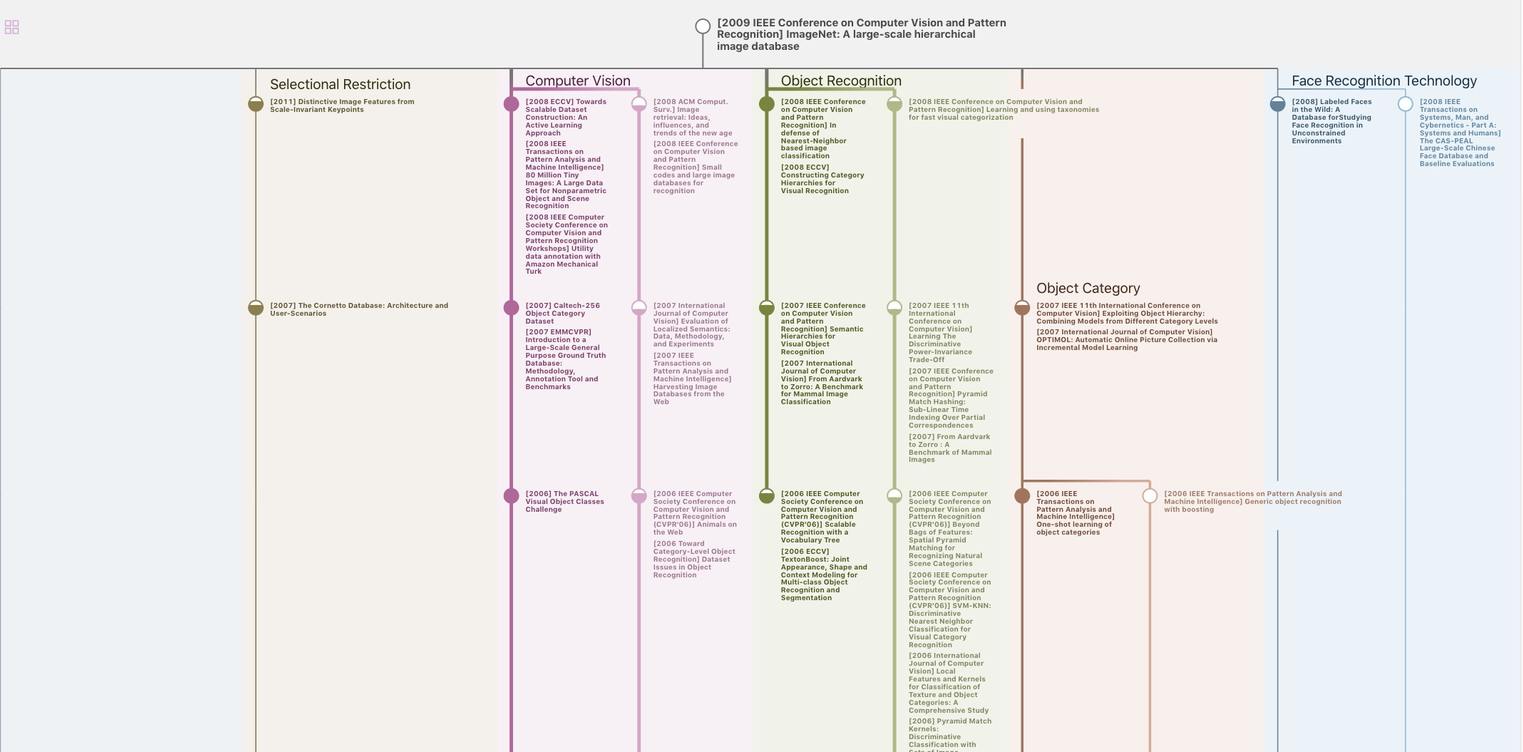
生成溯源树,研究论文发展脉络
Chat Paper
正在生成论文摘要