KIQA: Knowledge-Infused Question Answering Model for Financial Table-Text Data
PROCEEDINGS OF DEEP LEARNING INSIDE OUT (DEELIO 2022): THE 3RD WORKSHOP ON KNOWLEDGE EXTRACTION AND INTEGRATION FOR DEEP LEARNING ARCHITECTURES(2022)
摘要
While entity retrieval models continue to advance their capabilities, our understanding of their wide-ranging applications is limited, especially in domain-specific settings. We highlighted this issue by using recent general domain entity-linking models, LUKE and GENRE, to inject external knowledge into a question-answering (QA) model for a financial QA task with a hybrid tabular-textual dataset. We found that both models improved the baseline model by 1.57% overall and 8.86% on textual data. Nonetheless, the challenge remains as they still struggle to handle tabular inputs. We subsequently conducted a comprehensive attention-weight analysis, revealing how LUKE utilizes external knowledge supplied by GENRE. The analysis also elaborates how the injection of symbolic knowledge can be helpful and what needs further improvement, paving the way for future research on this challenging QA task and advancing our understanding of how a language model incorporates external knowledge.
更多查看译文
AI 理解论文
溯源树
样例
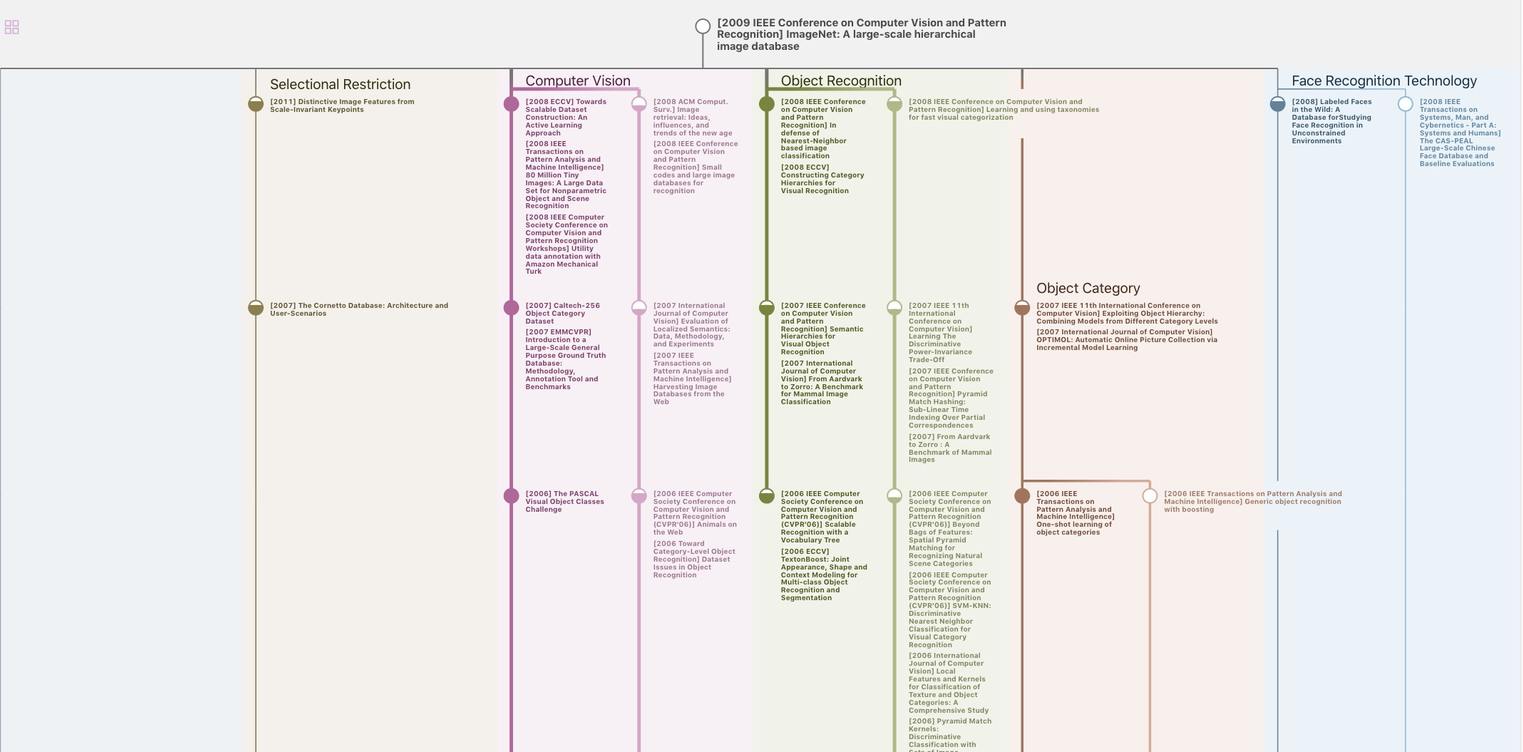
生成溯源树,研究论文发展脉络
Chat Paper
正在生成论文摘要