Simple and Effective Knowledge-Driven Query Expansion for QA-Based Product Attribute Extraction
PROCEEDINGS OF THE 60TH ANNUAL MEETING OF THE ASSOCIATION FOR COMPUTATIONAL LINGUISTICS (ACL 2022): (SHORT PAPERS), VOL 2(2022)
摘要
A key challenge in attribute value extraction (AVE) from e-commerce sites is how to handle a large number of attributes for diverse products. Although this challenge is partially addressed by a question answering (QA) approach which finds a value in product data for a given query (attribute), it does not work effectively for rare and ambiguous queries. We thus propose simple knowledge-driven query expansion based on possible answers (values) of a query (attribute) for QA-based AVE. We retrieve values of a query (attribute) from the training data to expand the query. We train a model with two tricks, knowledge dropout and knowledge token mixing, which mimic the imperfection of the value knowledge in testing. Experimental results on our cleaned version of AliExpress dataset show that our method improves the performance of AVE (+6.08 macro F-1), especially for rare and ambiguous attributes (+7.82 and +6.86 macro F-1, respectively).
更多查看译文
AI 理解论文
溯源树
样例
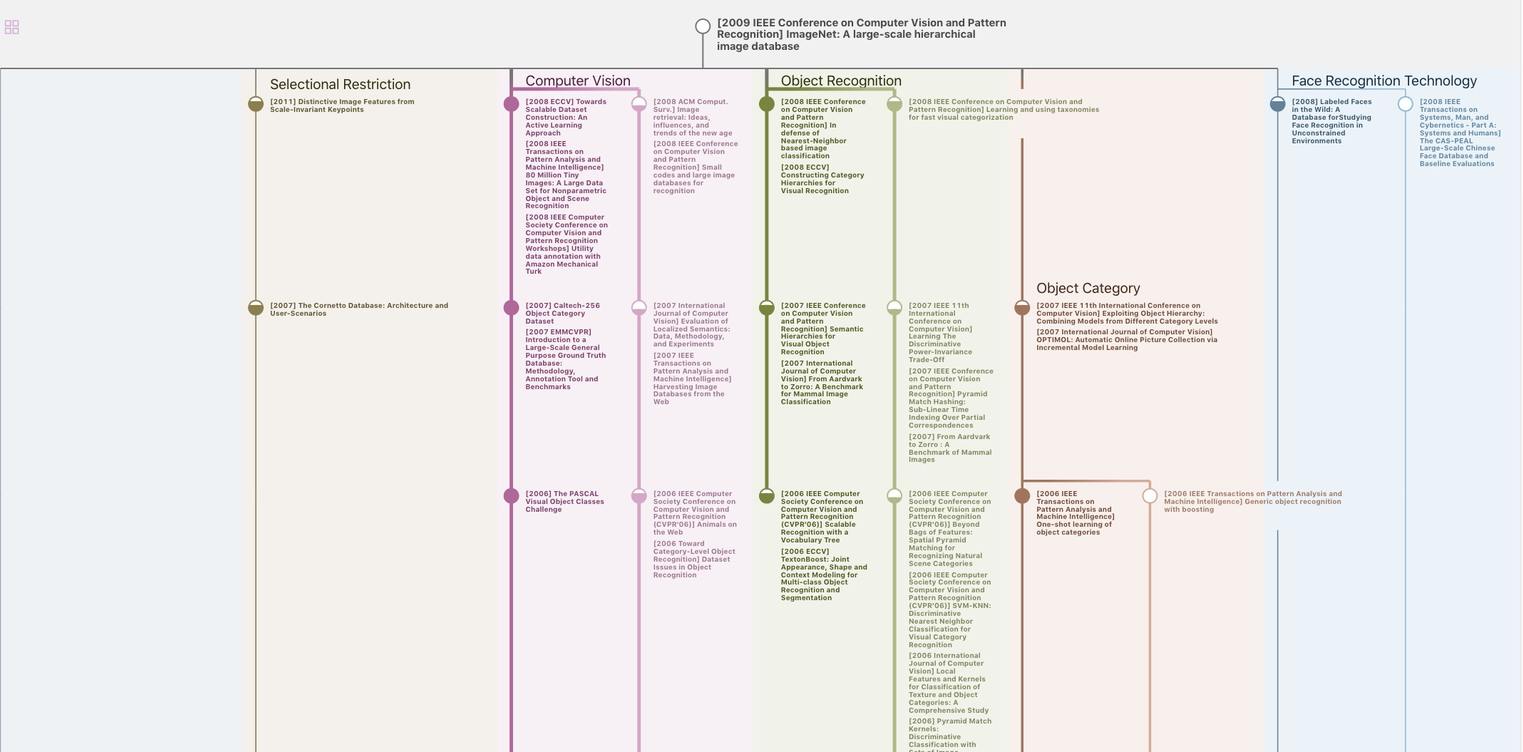
生成溯源树,研究论文发展脉络
Chat Paper
正在生成论文摘要