Detecting the critical states during disease development based on temporal network flow entropy
Briefings in Bioinformatics(2022)
摘要
Complex diseases progression can be generally divided into three states, which are normal state, predisease/critical state and disease state. The sudden deterioration of diseases can be viewed as a bifurcation or a critical transition. Therefore, hunting for the tipping point or critical state is of great importance to prevent the disease deterioration. However, it is still a challenging task to detect the critical states of complex diseases with high-dimensional data, especially based on an individual. In this study, we develop a new method based on network fluctuation of molecules, temporal network flow entropy (TNFE) or temporal differential network flow entropy, to detect the critical states of complex diseases on the basis of each individual. By applying this method to a simulated dataset and six real diseases, including respiratory viral infections and tumors with four time-course and two stage-course high-dimensional omics datasets, the critical states before deterioration were detected and their dynamic network biomarkers were identified successfully. The results on the simulated dataset indicate that the TNFE method is robust under different noise strengths, and is also superior to the existing methods on detecting the critical states. Moreover, the analysis on the real datasets demonstrated the effectiveness of TNFE for providing early-warning signals on various diseases. In addition, we also predicted disease deterioration risk and identified drug targets for cancers based on stage-wise data.
更多查看译文
关键词
critical state,dynamic network biomarker (DNB),high dimensional omics data,network flow entropy (NFE)
AI 理解论文
溯源树
样例
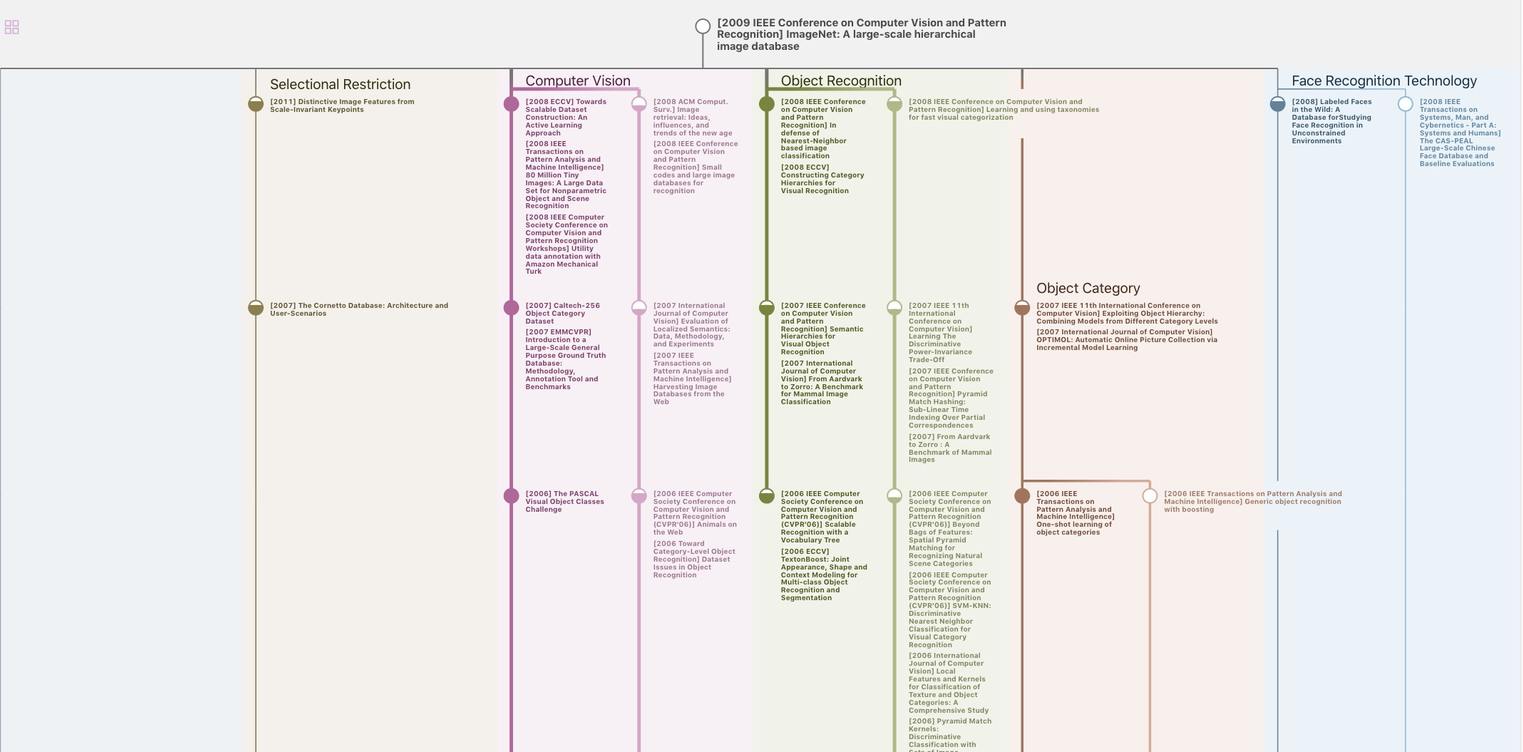
生成溯源树,研究论文发展脉络
Chat Paper
正在生成论文摘要