Multi-task manifold learning for partial label learning
Information Sciences(2022)
摘要
In partial label learning (PLL), each instance is associated with a candidate label set, and only one label is ground-truth. PLL aims to identify the ground-truth label out of these candidate labels. Most of the existing PLL approaches focus on single-task PLL, and ignore the auxiliary information of the related tasks. This paper puts forward a novel multi-task manifold learning method for partial label learning (MT-PLL), which learns multiple PLL tasks jointly, and incorporates the auxiliary information of the related tasks to improve the performance of PLL classifiers. MT-PLL assumes that the graph manifold structure guides the generation of labeling confidence for instances in each task. In addition, the information of related tasks can be used to boost the performance of the overall classification model. Then, a heuristic framework is used to optimize the objective function. Numerical experiments have demonstrated that MT-PLL can deliver better performance than state-of-the-art single-task PLL methods.
更多查看译文
关键词
Partial label learning,Multi-task learning,Manifold learning
AI 理解论文
溯源树
样例
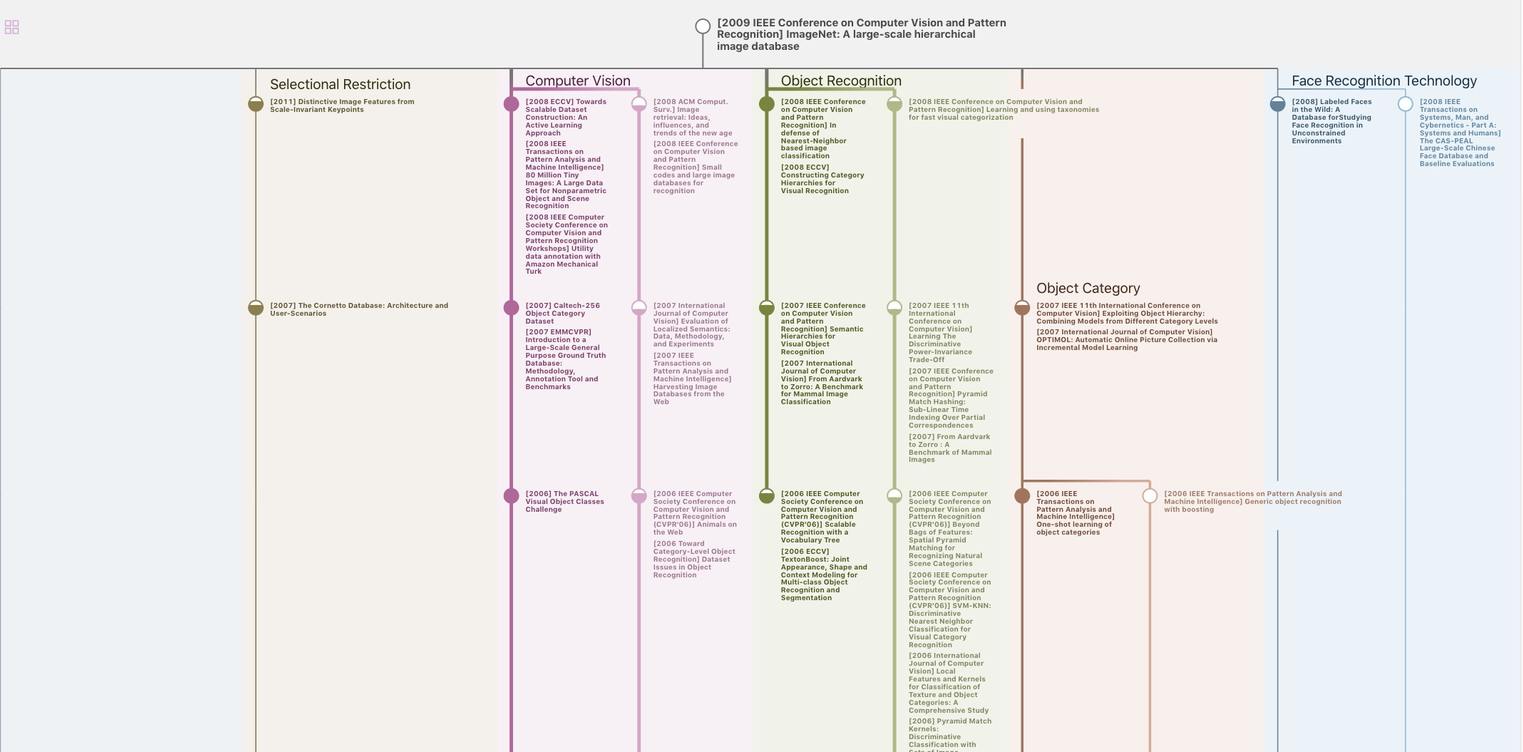
生成溯源树,研究论文发展脉络
Chat Paper
正在生成论文摘要