DDDM: A Brain-Inspired Framework for Robust Classification
European Conference on Artificial Intelligence(2022)
摘要
Despite their outstanding performance in a broad spectrum of real-world tasks, deep artificial neural networks are sensitive to input noises, particularly adversarial perturbations. On the contrary, human and animal brains are much less vulnerable. In contrast to the one-shot inference performed by most deep neural networks, the brain often solves decision-making with an evidence accumulation mechanism that may trade time for accuracy when facing noisy inputs. The mechanism is well described by the Drift-Diffusion Model (DDM). In the DDM, decision-making is modeled as a process in which noisy evidence is accumulated toward a threshold. Drawing inspiration from the DDM, we propose the Dropout-based Drift-Diffusion Model (DDDM) that combines test-phase dropout and the DDM for improving the robustness for arbitrary neural networks. The dropouts create temporally uncorrelated noises in the network that counter perturbations, while the evidence accumulation mechanism guarantees a reasonable decision accuracy. Neural networks enhanced with the DDDM tested in image, speech, and text classification tasks all significantly outperform their native counterparts, demonstrating the DDDM as a task-agnostic defense against adversarial attacks.
更多查看译文
关键词
Machine Learning: Robustness,Humans and AI: Brain Sciences
AI 理解论文
溯源树
样例
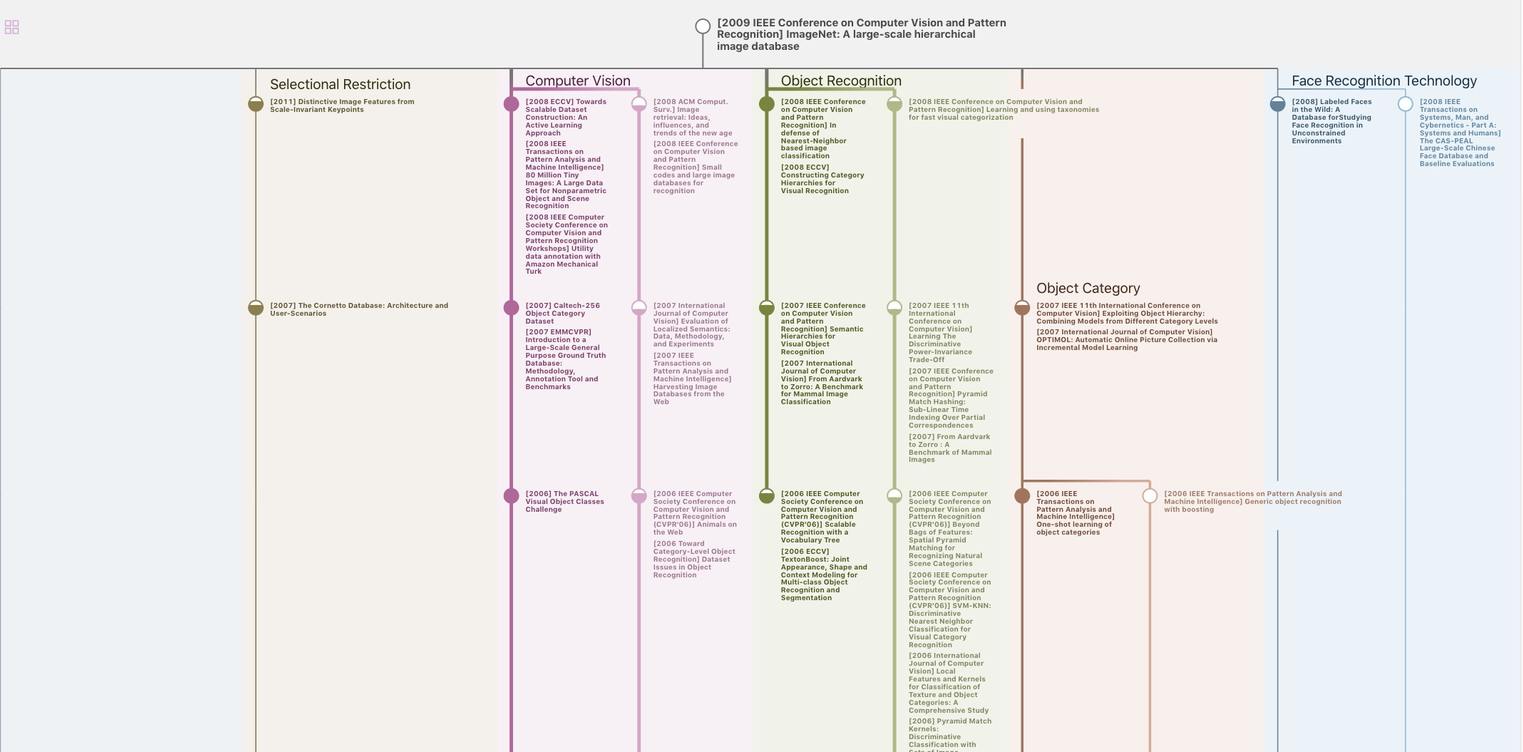
生成溯源树,研究论文发展脉络
Chat Paper
正在生成论文摘要