Testing Predictive Automated Driving Systems: Lessons Learned and Future Recommendations
IEEE Intelligent Transportation Systems Magazine(2022)
摘要
Conventional vehicles are certified through classical approaches, where different physical certification tests are set up on test tracks to assess the required safety levels. These approaches are well suited for vehicles with limited complexity and limited interactions with other entities as last-second resources. However, these approaches do not allow the evaluation of safety with real behaviors for critical and edge cases nor the evaluation of the ability to anticipate them in the mid or long term. This is particularly relevant for automated and autonomous driving functions that make use of advanced predictive systems to anticipate future actions and motions to be considered in the path planning layer. In this article, we present and analyze the results of physical tests on the proving grounds of several predictive systems in automated driving functions developed within the framework of the BRidging Gaps for the Adoption of Automated VEhicles (BRAVE) project. Based on our experience in testing predictive automated driving functions, we identify the main limitations of current physical testing approaches when dealing with predictive systems, analyze the main challenges ahead, and provide a set of practical actions and recommendations to consider in future physical testing procedures for automated and autonomous driving functions.
更多查看译文
关键词
Testing,Safety,Roads,Complexity theory,Behavioral sciences,Certification,Autonomous vehicles
AI 理解论文
溯源树
样例
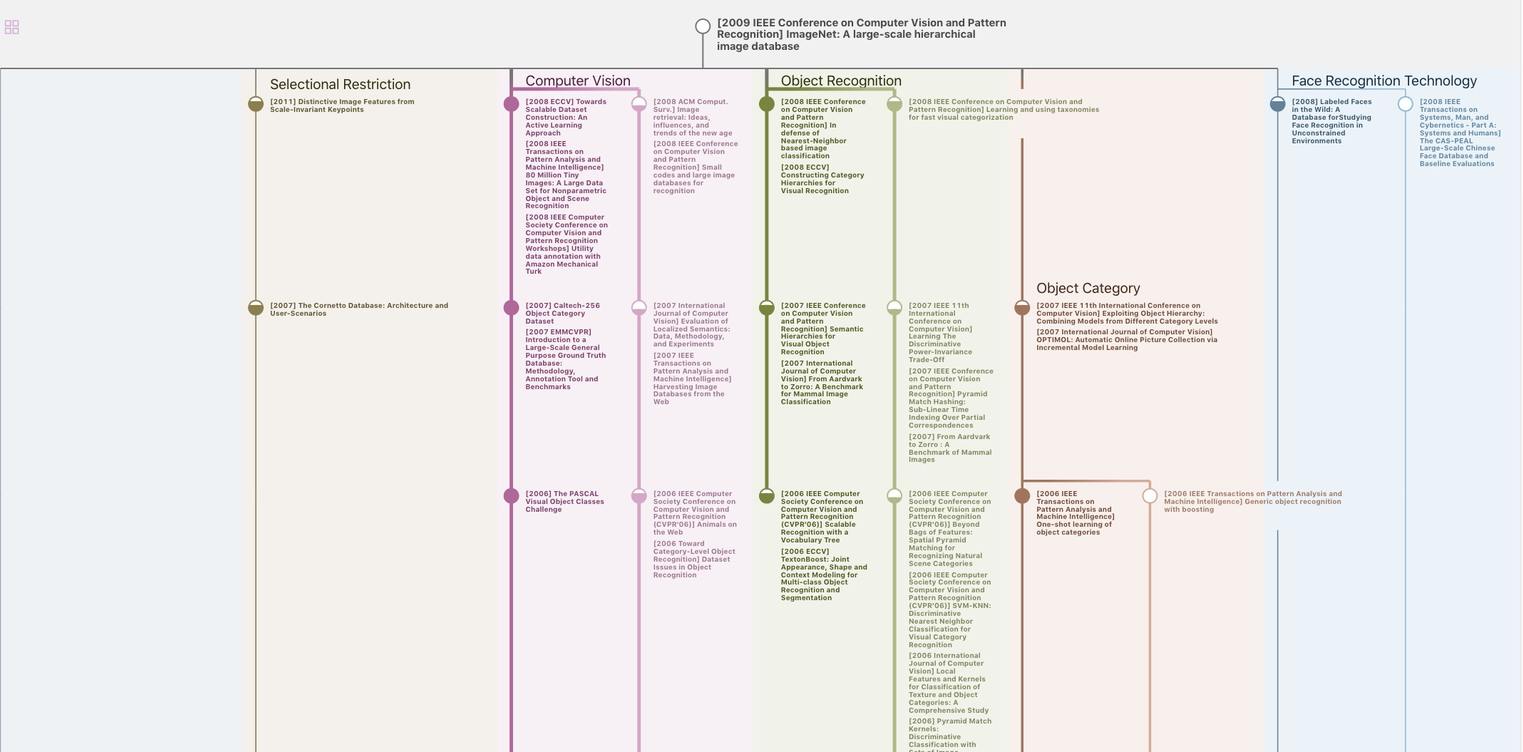
生成溯源树,研究论文发展脉络
Chat Paper
正在生成论文摘要