Archard model guided feature engineering improved support vector regression for rail wear analysis
Engineering Failure Analysis(2022)
摘要
•A physics model is applied to generate new features for the SVR model improvement when the raw data contains outliers.•A long-term rail wear degradation modelling and prediction approach is realized based on a 14-year in-field dataset.•A two-peak characteristic of rail wear was observed across the cant height value range.•Rail service time length is the most important factor for rail wear prediction.
更多查看译文
关键词
Rail wear analysis,Measurement data outliers,Physics model guided feature engineering,Support Vector Regression
AI 理解论文
溯源树
样例
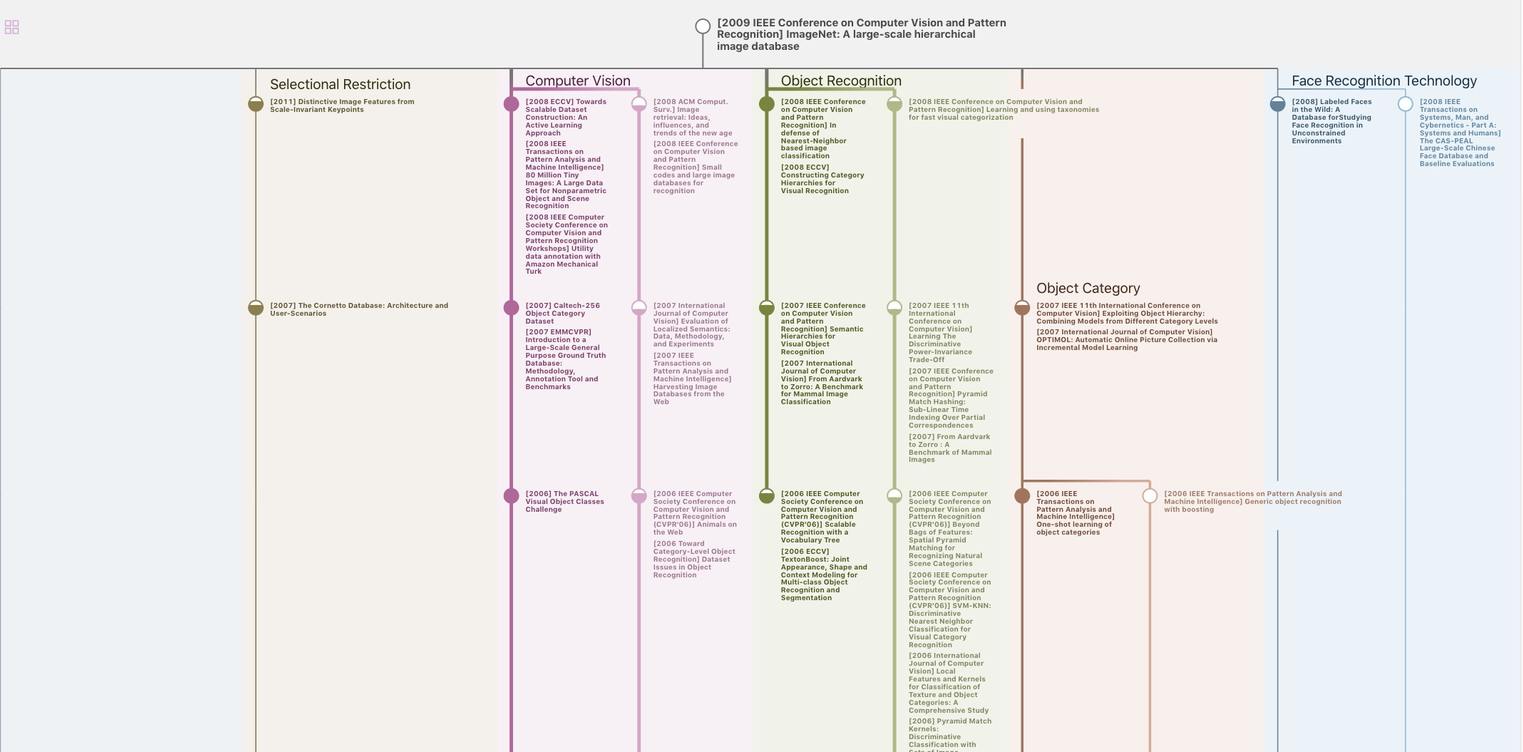
生成溯源树,研究论文发展脉络
Chat Paper
正在生成论文摘要