Federated Learning Aggregation: New Robust Algorithms with Guarantees
arxiv(2022)
摘要
Federated Learning has been recently proposed for distributed model training at the edge. The principle of this approach is to aggregate models learned on distributed clients to obtain a new more general "average" model (FedAvg). The resulting model is then redistributed to clients for further training. To date, the most popular federated learning algorithm uses coordinate-wise averaging of the model parameters for aggregation. In this paper, we carry out a complete general mathematical convergence analysis to evaluate aggregation strategies in a federated learning framework. From this, we derive novel aggregation algorithms which are able to modify their model architecture by differentiating client contributions according to the value of their losses. Moreover, we go beyond the assumptions introduced in theory, by evaluating the performance of these strategies and by comparing them with the one of FedAvg in classification tasks in both the IID and the Non-IID framework without additional hypothesis.
更多查看译文
关键词
aggregation,new robust algorithms,guarantees
AI 理解论文
溯源树
样例
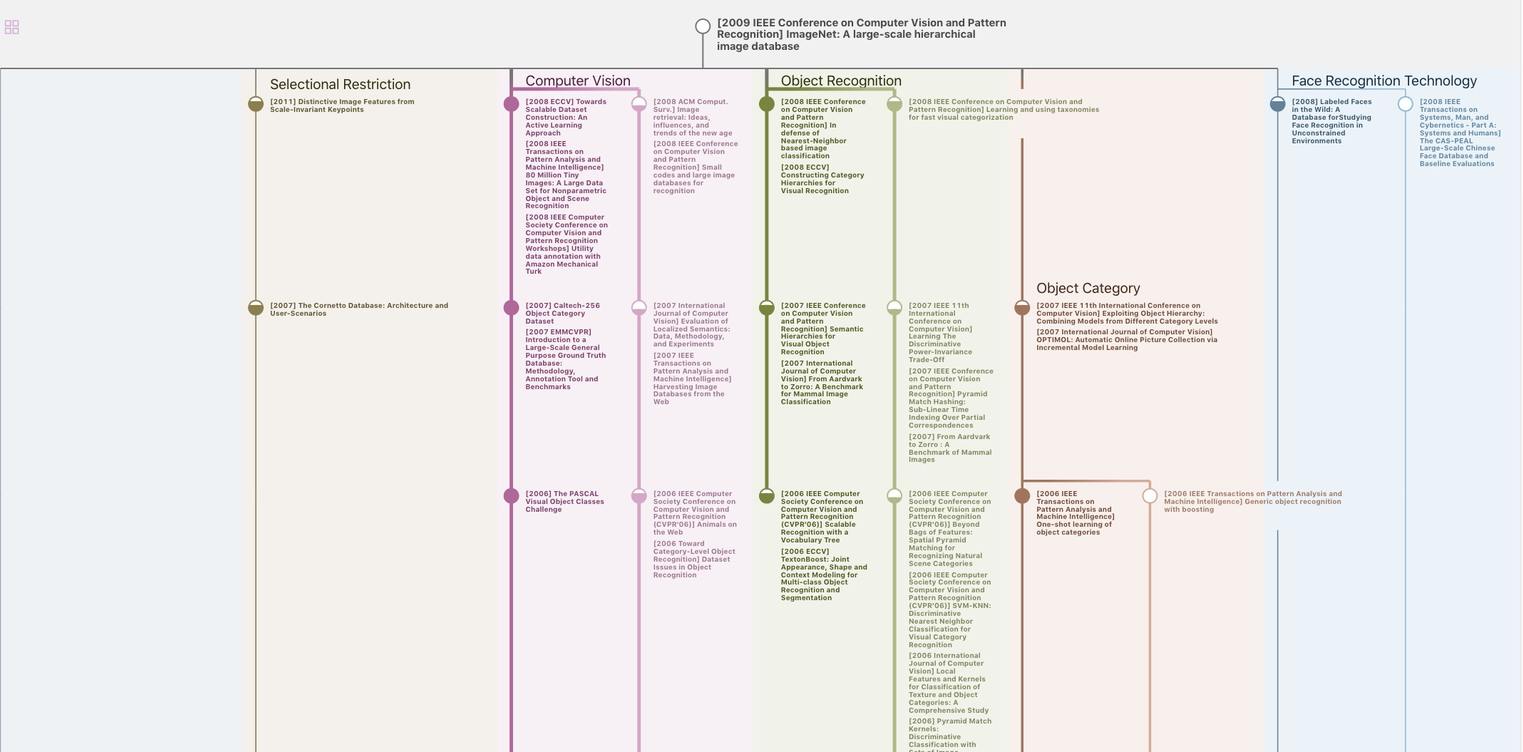
生成溯源树,研究论文发展脉络
Chat Paper
正在生成论文摘要