Analysis of functional neural codes of deep learning models
arXiv (Cornell University)(2022)
摘要
Deep neural networks (DNNs), the agents of deep learning (DL), require a massive number of parallel/sequential operations. This makes it extremely challenging to comprehend DNNs' operations and hinders proper diagnosis. Consequently, DNNs cannot be readily used in high-stakes domains, in which incorrect decisions can lead to catastrophic failures. Therefore, to build more reliable DNNs/DL to be deployed in high-stakes real-world problems, it is imperative that we develop proper analysis tools that will allow us to better understand DNNs' internal operations underlying their decision-making. Here, we used the self-organizing map (SOM) to analyze internal codes of DL models associated with their decision-making. Our analyses suggest that hidden layer activation patterns can be mapped onto a finite number of patterns and are correlated with DL predictions, raising the possibility that they could serve as functional codes of DL models. Encouraged by this observation, we further used SOM to estimate input features coded in hidden layers, analyzed the effects of adversarial inputs to better understand characterized internal representations' evolution and adversarial perturbations' propagation in DL models.
更多查看译文
关键词
functional neural codes,deep learning models,deep learning
AI 理解论文
溯源树
样例
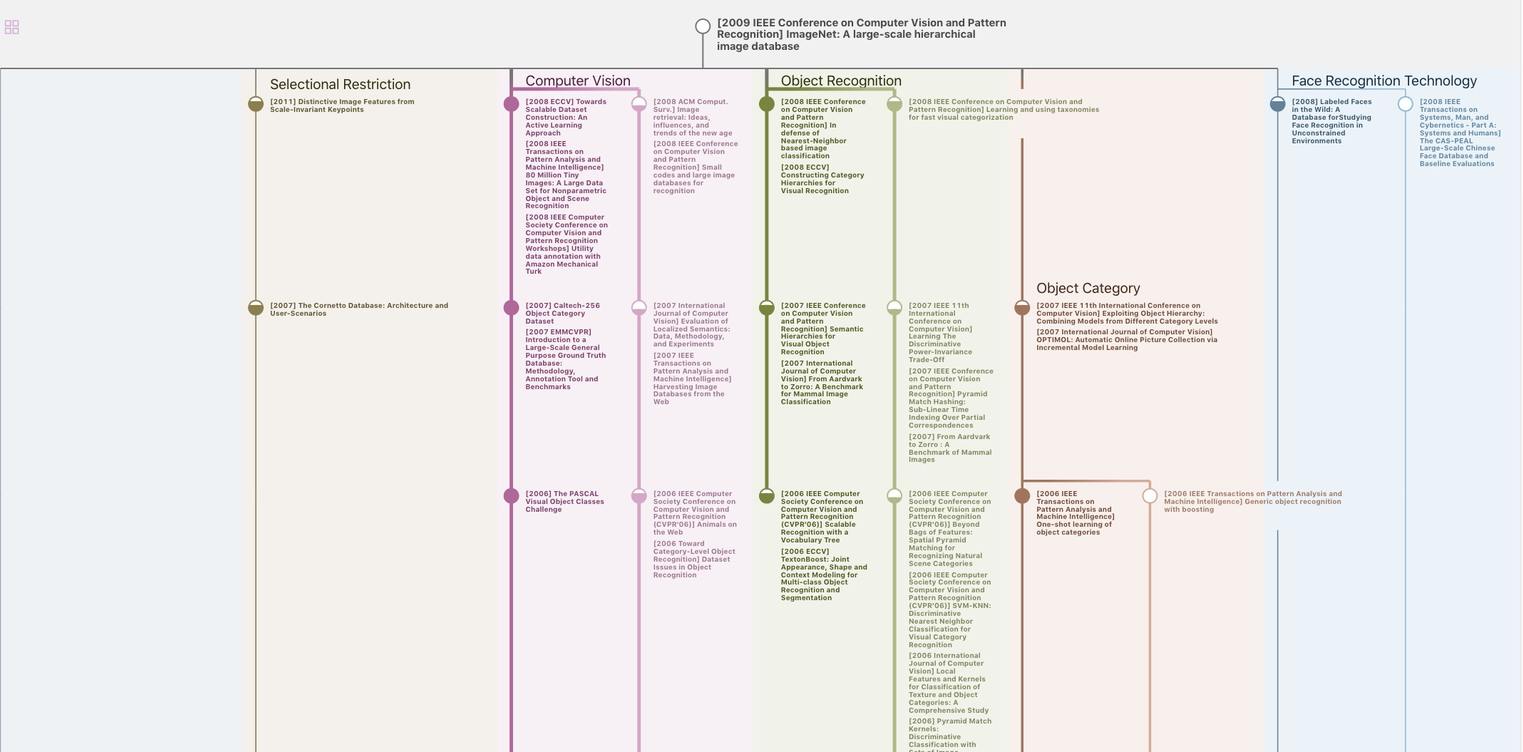
生成溯源树,研究论文发展脉络
Chat Paper
正在生成论文摘要