MAGCNSE: predicting lncRNA-disease associations using multi-view attention graph convolutional network and stacking ensemble model
BMC Bioinformatics(2022)
摘要
Background Many long non-coding RNAs (lncRNAs) have key roles in different human biologic processes and are closely linked to numerous human diseases, according to cumulative evidence. Predicting potential lncRNA-disease associations can help to detect disease biomarkers and perform disease analysis and prevention. Establishing effective computational methods for lncRNA-disease association prediction is critical. Results In this paper, we propose a novel model named MAGCNSE to predict underlying lncRNA-disease associations. We first obtain multiple feature matrices from the multi-view similarity graphs of lncRNAs and diseases utilizing graph convolutional network. Then, the weights are adaptively assigned to different feature matrices of lncRNAs and diseases using the attention mechanism. Next, the final representations of lncRNAs and diseases is acquired by further extracting features from the multi-channel feature matrices of lncRNAs and diseases using convolutional neural network. Finally, we employ a stacking ensemble classifier, consisting of multiple traditional machine learning classifiers, to make the final prediction. The results of ablation studies in both representation learning methods and classification methods demonstrate the validity of each module. Furthermore, we compare the overall performance of MAGCNSE with that of six other state-of-the-art models, the results show that it outperforms the other methods. Moreover, we verify the effectiveness of using multi-view data of lncRNAs and diseases. Case studies further reveal the outstanding ability of MAGCNSE in the identification of potential lncRNA-disease associations. Conclusions The experimental results indicate that MAGCNSE is a useful approach for predicting potential lncRNA-disease associations.
更多查看译文
关键词
LncRNA-disease associations, Multi-view, Graph convolutional network, Attention mechanism, Convolutional neural network, Stacking ensemble model
AI 理解论文
溯源树
样例
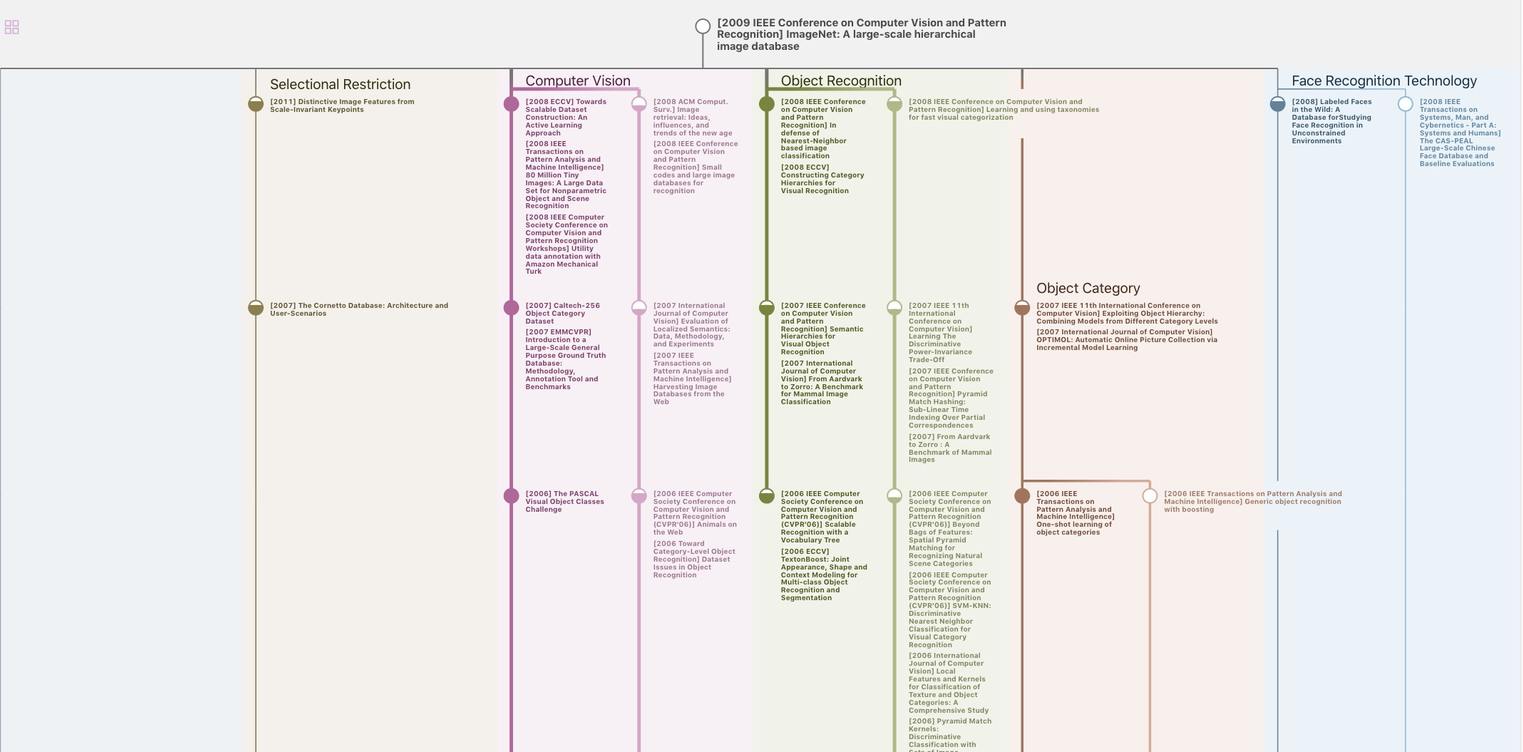
生成溯源树,研究论文发展脉络
Chat Paper
正在生成论文摘要