Hormone ratios suffer from striking lack of robustness to measurement error
Psychoneuroendocrinology(2022)
摘要
Hormone ratios are often used to capture the joint effect (or “balance”) of two hormones with opposing or mutually suppressive effects. Despite some statistical and interpretative problems, hormone ratios are being increasingly used to examine associations of testosterone/cortisol, estradiol/progesterone, testosterone/estradiol, and other hormone pairs. Here we discuss a methodological problem that has not been previously recognized, namely, the striking lack of robustness of raw hormone ratios in the face of measurement error. Hormone levels are measured with error, both due to inability of assays to perfectly assess concentrations “in the tube” and due to discrepancies between levels at the time of sample collection and effective levels that produce the physiological and/or behavioral effect of interest. Noise in measured hormone levels can be substantially exaggerated by ratios, especially when the distribution of the hormone at the denominator is positively skewed, as is frequently observed. To evaluate the extent of this problem and explore the conditions that exacerbate it, we present two sets of simulations, one using idealized distributions and one using empirically observed distributions from studies of estrogen and progesterone. Results show that the validity of raw hormone ratios—the correlation between measured levels and underlying effective levels—drops rapidly in the presence of realistic levels of measurement error. Log-ratios are much more robust to measurement error, and their validity is more stable across samples; under some conditions (e.g., moderate amounts of noise with positively correlated hormone levels), they may provide a more valid measurement of the underlying raw ratio than the measured raw ratio itself. These findings have important implications for research that uses hormone ratios as predictors.
更多查看译文
关键词
Hormone ratios,Log-ratios,Measurement error,Robustness
AI 理解论文
溯源树
样例
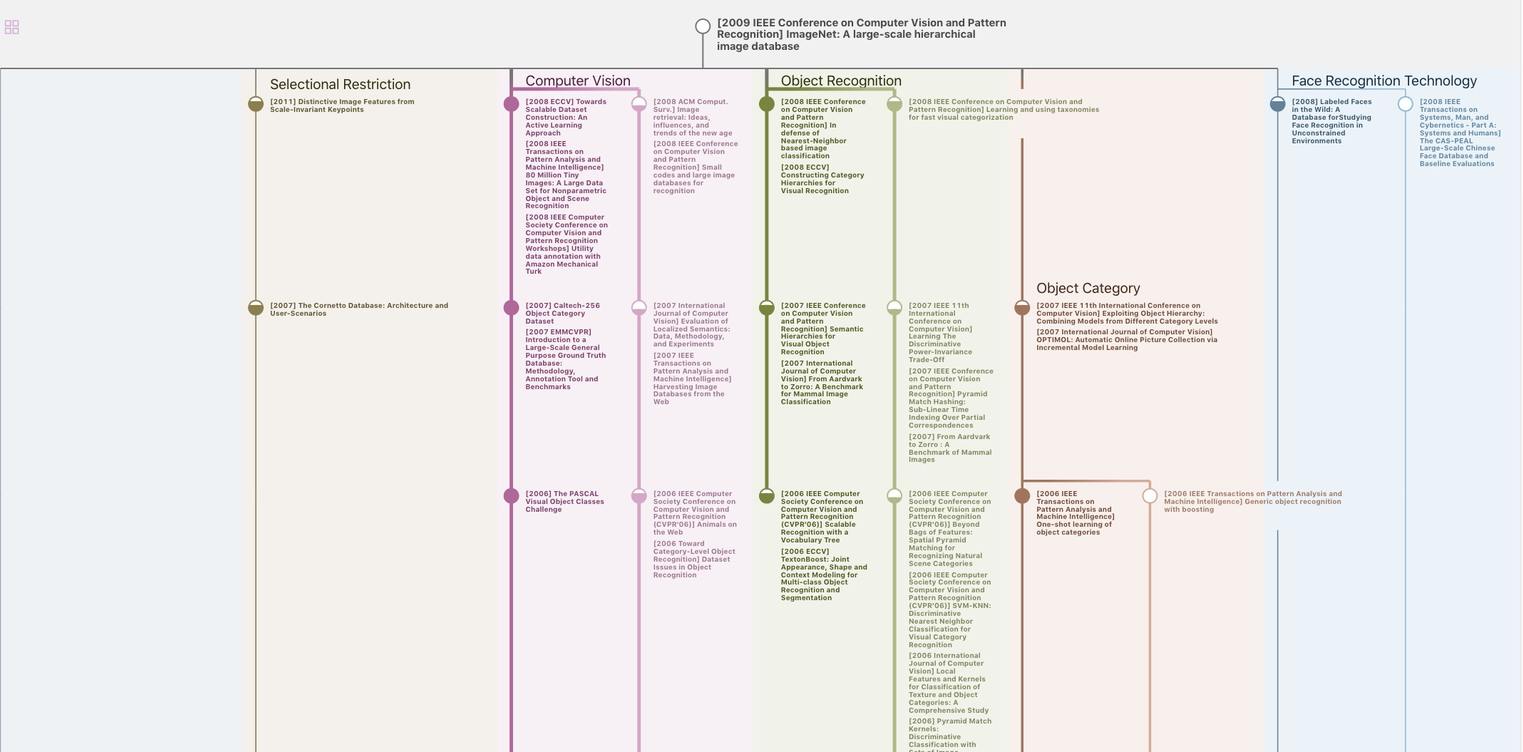
生成溯源树,研究论文发展脉络
Chat Paper
正在生成论文摘要